Advertisement

Teaching and learning of probability
- Original Paper
- Open access
- Published: 04 August 2023
- Volume 56 , pages 5–17, ( 2024 )
Cite this article
You have full access to this open access article
- Carmen Batanero ORCID: orcid.org/0000-0002-4189-7139 1 &
- Rocío Álvarez-Arroyo ORCID: orcid.org/0000-0002-3201-8542 1
10k Accesses
5 Citations
Explore all metrics
In this paper, we develop a personal synthesis of the most outstanding research on the teaching and learning of probability in the past years. We conducted a systematic search to examine publications on this topic in mathematics education, statistics education, education, and psychology journals. This exploration was complemented by additional studies published in conference proceedings or books. We classified these papers to highlight the main recent research tendencies in the field, according to the theme studied and considering the research objectives. Epistemological analyses suggest that informal inference based on simulation diminishes the topic abstraction but reduces probability to its frequentist view. Topics receiving particular attention include children’s probabilistic knowledge, the effect of visualizations on solving conditional probability problems, teachers’ education and probability modelling. In the final section, we recommend relevant points in which more investigation is needed to complete our knowledge about teaching and learning. In particular, we miss research on teachers’ mathematical knowledge of many probability concepts and on their didactic knowledge.
Similar content being viewed by others
Teaching and Learning of Probability
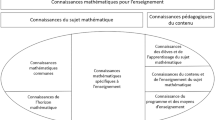
Pratiques déclarées d’enseignement des probabilités: enquête auprès de personnes enseignantes du primaire et secondaire au Québec
Three Approaches for Modelling Situations with Randomness
Avoid common mistakes on your manuscript.
1 Foundations
Research in probability education has a long tradition and comes from psychology, statistics and mathematics education, as summarised in synthesis works (e.g., Batanero et al., 2016 ; Borovcnik & Peard, 1996 ; Jones et al., 2007 ; Pratt & Kazak, 2018 ; Shaughnessy, 1992 ). This research provides support to the citizen's need to cope with uncertainty and risk in the modern world.
The first studies by Piaget and Inhelder ( 1951 ) investigated the developmental growth of children’s probabilistic reasoning and described the stages of this development. Other psychologists continued this research, the most relevant Fischbein ( 1975 ), who analysed stochastic intuitions and recommended early probability teaching. Another research program in psychology described people's strategies to compute probabilities ( heuristics ) and the resultant systematic errors ( biases ). These biases appear in decision-making, even in statistically well-trained adults (Gilovich et al., 2002 ).
A second influence comes from statistics, particularly with the International Conferences on Teaching Statistics (ICOTS) promoted by the International Statistical Institute first and afterwards by the International Association for Statistics Education. Statisticians teach statistics at the university level in service courses directed to various types of students with (in general) little experience in calculus or algebra. Consequently, many statistics lecturers were interested in investigating their students’ difficulties and exploring teaching resources to facilitate understanding.
Research from mathematics education arose to face the specific challenges set by the teaching of probability at the school level, being the only part of mathematics dealing with uncertainty. In addition to teaching and learning problems, technology and resources, mathematics educators were interested in epistemological and curricular studies and the education of teachers. This research has received an impulse from research groups dealing with the topic in the International Congresses of Mathematics Education (ICME), Conferences of the European Group in Mathematics Education (CERME), International Congresses on Teaching Statistics (ICOTS), and other statistics or mathematics education conferences. Specific books (e.g., Batanero & Chernoff, 2018 ; Chernoff & Sriraman, 2014 ; Jones, 2005 ; Kapadia & Borovcnik, 1991 ) have complemented this work, which includes theoretical and empirical studies supported by different theoretical frameworks.
Probability is a part of statistics, which is included in mathematics in schools, although, in university, these topics are often disconnected. In many countries, different graduate programmes train mathematicians and statisticians, who count on their own research conferences and specific journals. Other features distinguishing these areas support the view of statistics education as a specific research field (Batanero & Borovcnik, 2016 ).
Statistics includes exploratory data analysis (EDA), inference, and probability, this providing the mathematical models that make the transition from EDA to inference possible. Because probability is more linked than statistics to mathematics in its historical origins and methods, we view probability education as a part of both mathematics and statistics education.
We concentrate on probability education, although incidentally touch inference when describing modelling. We revisit the investigation conducted since 2018, where a varied and growing production is evident, to identify the main tendencies and suggest ideas to continue research. We classified these studies in areas which include research from psychology, statistics and mathematics education and slightly modify those suggested in Batanero et al. ( 2016 ). The resulting categories organise the different sections in this survey and offer new research directions in the final section:
Epistemological analyses: Reflection on the nature of different concepts is especially relevant in probability because different views of probability still coexist and influence statistical practice and the teaching of probability (Batanero et al., 2016 ; Borovcnik & Peard, 1996 ).
Analysis of probability in the school curricula: The debates between the different views of probability and formalization level preferable in teaching influence the school curricula. Changes in curricular guidelines, textbook content, and examination tests are examined by several researchers .
Probabilistic reasoning of children: Recent interest in introducing intuitive notions of probability and probabilistic language in kindergarten and primary school resulted in new research analysing children’s reasoning in probability.
Intuitions and learning difficulties in probability: Other authors investigated teaching and learning different topics to secondary, high school and university students. The interest was identifying the associated learning problems and erroneous beliefs.
The role of visualization in improving Bayesian reasoning: We observed a wealth of research exploring different resources to increase capacity and overcome reasoning biases in solving conditional probability and Bayes’ problems.
Probability modelling: While the interest in probability modelling is not new, there is a recent interest in using probability models to connect data and chance, as well as the classical and frequentist views of probability.
Education of teachers: Finally, an increasing number of studies analysed different components of preservice and in-service teachers’ knowledge to teach probability and in-service teachers’ practices.
1.1 Methodological approach
We conducted a systematic search for the period 2018–2023 in the journals compiled in the Web of Science and Scopus. We expanded the inquiry to the Google Scholar and Research Gate databases, the recent CERME, ICOTS and ICME proceedings, specific books (e.g., Batanero & Chernoff, 2018 ; Leavy et al., 2018 ) and thematic issues (e.g., in Canadian Journal of Science, Mathematics and Technology Education, 2022; Mathematics, 2022, and ZDM — Mathematics Education issue on Innovations in Statistical Modelling, 2018).
We explored this material using combinations of the keywords teaching, learning, understanding, difficulties, education, curricular issues or epistemology and one of the words chance, distribution, independence, probability, randomness, random variable, sample space and sampling. As inclusion criteria, the papers should be written in English, contain empirical or theoretical research, be peer-refereed and be centred on the survey topic. From the initial set of references, we excluded documents written in other languages, published in regional journals and articles that only described teaching materials or suggestions with no research involved. If possible, we substituted proceedings papers with more complete publications from the same authors. We used recent references in each paper identified and we performed personal requests for papers to some authors to enrich the set of works in the survey. For each article finally selected, we compiled on an Excel file bibliographical information, a short personal abstract and information about the study topic and approach, sample, material analysed and conclusions. We used this information to classify and compare the papers, and after successive readings of all the documents, we progressively refined the initial list of categories in the survey.
To follow, we present the results and finish with some suggestions to continue research in the area. In the references, we include some annotated papers selected to reflect the variety of topics in probability education, and because of their outstanding interest.
2 Epistemological analyses
The epistemological reflection helps clarify different approaches to probability that we describe briefly following Batanero et al. ( 2016 ), Borovcnik ( 2021 ) and Chaput et al. ( 2011 ):
In the classical or Laplacian meaning, probability is the fraction of the number of cases favourable to a particular event divided by the number of all cases possible. It is only applicable when all the elementary events are equally likely, for example, in games of chance. In the frequentist approach, probability is defined as the hypothetical number towards which the relative frequency tends when a random experiment is repeated infinitely many times. Since we only perform a finite number of trials, with this approach, we do not obtain the exact probability value but an approximation of it.
The propensity meaning assumes probability as a measure of the tendency of a random process to produce a given outcome in the long run; indirectly is linked to singular events (Borovcnik, 2021 ). In the logical meaning, probability is a rational degree of confirmation that measures the support provided by some evidence E to a given hypothesis H and is an objective value with implication and incompatibility in the extremes.
In the subjectivist view, probability is a personal degree of belief that depends on a person’s knowledge or experience and the repetition of a situation is not needed to assign probability. Therefore, the field of application of probability is wider. Finally, in the axiomatic view , probability is a normed measure defined in an algebra of events with values in the interval [0, 1] that fulfils a series of axioms.
The classical, frequentist and subjectivist approaches still influence the school curricula while the axiomatic view is mainly included in advanced mathematical courses and the remaining views are primarily studied by philosophers. Moreover, the frequentist and subjectivist views of probability impact the various methodologies of inference and questions related to measuring and justifying these views still are debated (Borovcnik, 2021 ).
Finally, there is an increasing interest in the concept of risk because of its many applications. Moreover, we should link risk and probability in teaching because of the relevance of risk situations as a context to develop the different meanings of probability (Borovcnik & Kapadia, 2018 ).
3 Analysis of probability in the school curricula
Some research inspected the probability curricular content for different school levels or compared this content to that reflected in the textbooks or external examinations.
By 2018, most USA states had eliminated probability from the primary school curricula and diminished it in the lower secondary school (Langrall, 2018 ). However, recent curricula (e.g., ACARA, 2020 , MEFP, 2022 and the countries listed by Langrall, 2018 ) introduce probability since primary school. The rationale is to help children develop correct probability intuitions. Moreover, students who only experience probability in secondary school may not acquire a sound understanding of main probability concepts and not be prepared for a later formal study (Langrall, 2018 ).
Mathematics textbooks support teachers and students, although they can also implement instructional changes (Rezat et al., 2021 ). Consequently, some authors analysed the probability content in textbooks. For example, in Chilean secondary education textbooks (Carrera et al., 2021 ), the random variable was mainly presented as a variable of interest, forgetting other meanings (magnitude, statistical variable or function). Graphic and tabular representations of the random variable were scarce compared with symbols. The textbooks almost omitted the definition and classification of random variables (discrete or continuous). Contexts were much richer in history than in mathematics textbooks.
A strong influence on teaching comes from external assessment. In Spain, the University Entrance Tests evaluate the knowledge and maturity of students who intend to enter university. However, an analysis of these tests over 12 years revealed the potential difficulty of the problems posed and the high emphasis given to conditional probability compared to other probabilistic contents (Batanero et al., 2018 ).
4 Probabilistic reasoning in young children
Classical research on children's probabilistic reasoning assumed that children's ideas about chance and probability take time to develop, as compared to arithmetic or geometric reasoning (Batanero et al., 2016 ; Groth et al., 2021 ; Jones & Thornton, 2005 ). Today researchers have overcome this assumption, and new work has examined young children’s initial ideas of chance and probability (Batanero et al., 2021 ; Nikiforidou, 2018 ). Most of this research has considered the classical meaning of probability; however, some studies also relate the subjectivist and frequentist meanings (Kazak & Leavy, 2018 , 2022 ).
In this section, we examine late research dealing with children until 12 years of age, which has considered four main topics: (a) children's distinction between determinism and randomness, (b) the emergence of probabilistic language, (c) combinatorial reasoning, and (d) children’s reasoning when comparing probabilities (Fig. 1 ).
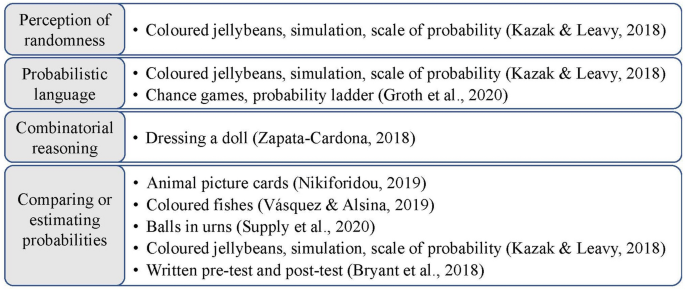
Main topics and material used in research on young children’s probabilistic reasoning
4.1 Perception of randomness
Recent research has analysed the children’s connection between randomness and unpredictability and their understanding of the independence of trials from a random experiment. The conclusion is that between 3 and 4 years of age, children begin to differentiate between deterministic and random situations. They demonstrate an intuitive understanding of the random phenomena uncertainty, and the unpredictability of their outcomes and begin to express their probabilistic intuitions verbally at 4–5 years of age. Around 5, children develop explanations for the impossibility of predicting random outcomes. At about 7, children imagine the distribution of a random set of results and qualitatively predict the likelihood of an event (Batanero et al., 2021 ). Children 7–8 years old can reason about uncertainty from the classical, frequentist and subjectivist perspectives (Kazak & Leavy, 2018 ). Moreover, with appropriate teaching, 9–10-year-olds acquire an understanding of the connection between randomising, unpredictability and independence of successive outcomes in a random sequence (Bryant et al., 2018 ).
4.2 The emergence of probabilistic language
Children use chance language in formal education and their daily lives when playing games of chance. Children (7–8-year-olds) use probability language relatively accurately to describe the likelihood of chance events; most have a quantitative understanding of equal likelihoods; impossibility and certainty, however, are difficult for the children (Kazak & Leavy, 2018 ). In expressing their likelihood before and after experiments and simulations, the children reason about probability from a subjective point of view. It is intuitive for them to modify predictions based on experiment and simulation results (Kazak & Leavy, 2018 ).
Five aspects in developing the probability vocabulary are incrementality, multidimensionality, polysemy, interrelatedness, and heterogeneity. Groth et al. ( 2020 ) analysed how these aspects appeared in a small group of 11–12-year-olds when learning probability in design-based research. One task was to place some events on a probability ladder , with impossible events on the bottom and sure events on the top. Students had considerably more trouble using certain than impossible, especially during the early phases of the research. Moreover, qualitative language for probability did not always precede the children’s quantitative reasoning (Groth et al., 2020 ).
4.3 Combinatorial reasoning
Children need the combinatorial ability to enumerate the different events in the sample space of random experiments and compute related probabilities. Because of its relation to multiplicative reasoning, combinatorial capacity takes longer to develop (Jones & Thornton, 2005 ). Young children solve simple enumeration problems using trial and error, and with no instruction, there is no noticeable improvement in their strategies. Thus, when finding all the different ways to dress a doll, 6–8-year-old children did not use a structured method, although the teacher's interaction helped improve the children’s reasoning (Zapata-Cardona, 2018 ).
4.4 Comparing and estimating probabilities
When comparing probabilities, the children are requested to select, between two choices (for example, two urns with blue and yellow balls), the option which provides a higher likelihood of a given result. Other times children are asked to estimate the probability of a given event. This research has used different materials (see Fig. 1 ). From the age of 3, children start comparing probabilities but systematically prefer the set with more favourable cases (Batanero et al., 2021 ). Between 4 to 6 years, many children can deduce the most likely event in a simple experiment and compare simple probabilities. Thus, most 4–6-year-olds correctly compared probabilities for some events when the number of possible cases was identical or small in an experiment using a material built on their own (colouring and cutting out paper fishes) (Vásquez & Alsina, 2019 ). Similarly, 70% of 4–6-years-olds predicted the most likely animal would result when turning the cards upside down in Nikiforidou’s ( 2019 ) experience with mixed animal picture cards.
Children's strategies improve with age, and by 6–7, they can use favourable and unfavourable elements to decide between two situations when the proportions are simple. A developmental change occurs between 6 and 8 years, with younger children primarily comparing the number of winning elements and older children using more elaborate strategies (Supply et al., 2020 ). Moreover, the numerical abilities of preschool children are related to the children's probability abilities in grades 1–3 of primary school (Supply et al., 2022 ).
While the above research focused on the classical view of probability, Kazak and Leavy ( 2022 ) investigated 7–8-year-olds understanding of subjectivist and frequentist probability. The author explored how children related these views of probability when estimating the probability of an event using data obtained by physical experiments and computer simulations. Although the children taking part in the study still had not reached enough quantitative reasoning of probability, many of them used the data to update their initial subjective probabilities, which were based on their personal beliefs.

5 Intuitions and learning difficulties in probability
Successful probability teaching requires foreseeing the informal ideas and difficulties that students bring to the classroom. Part of the research on this area continues previous psychological studies on reasoning biases summarised in Gilovich et al. ( 2002 ). We also found studies of difficulties in the formal learning of specific concepts.
5.1 Biases in probabilistic reasoning
Recent research agrees that formal education in probability does not significantly influence reasoning biases. For example, Kaplar et al. ( 2021 ) found many engineering students holding the following wrong reasoning: insensitivity to sample size (disregarding the effect of sample size on the sampling variability), base rate fallacy (not taking into account prior probabilities in applications of conditional probability), representativeness (expecting a short sequence of outcomes of random experiments will have the main characteristics of the random process), illusory correlation (assuming correlation when it does not exist) and not distinguishing conjunctive and disjunctive events. In the fallacy of composition (Chernoff et al., 2018 ) individuals infer something to be true about a whole (e.g., equiprobability of compound events in flipping several coins) when it is true for a part (equiprobability of events in a single coin).
Children’s and adults’ judgments about the fairness of chance games revealed similar low performance and misconceptions, which indicated that maturity and experience are not enough to develop understanding of independence, evaluation of the sample space, and perception of proportionality when comparing probabilities (Batista et al., 2022 ). Representativeness and the base-rate fallacy were partially overcame in a teaching experiment where 9th graders helped develop the teaching material and acted as teachers for a group of 5th graders. Data were provided in absolute frequencies, and the teaching was supported by the visualizations described in the next section (Vargas et al., 2019 ). The results suggested an overall improvement in the students in both grades.
5.2 Formal leaning
Beckman and delMas ( 2018 ) explored undergraduates’ responses to a task where students should identify the sample, population, statistic, and parameter in an invented context. The students’ responses describing the sample and population were accurate, while statistics and parameters were confused with a variety of other concepts. Salinas-Herrera and Salinas-Hernández ( 2022 ) analysed formal learning of the binomial distribution supported by the software Fathom. Although most high school students correctly interpreted the simulation results and used the software to solve probability problems, not all accepted the convergence of the binomial towards the normal distribution. Thus, the teacher should explicitly introduce the Law of Large Numbers since the students did not discover this theorem.
6 The role of visualization in improving Bayesian reasoning
Many reasoning biases, such as the base-rate fallacy or the confusion of the inverse (identifying P(A|B) with P(B|A) ) appear in Bayesian tasks (problems involving conditional probability and the Bayes’ rule). People do not only face these problems in the mathematics classroom but in many professional settings, where a sound decision depends on their solutions. Presenting the problem information in natural frequencies (frequencies obtained by sampling elements preserving base rate and sub-sample information) rather than in probability or percentages increases people’s performance in these situations (Vargas et al., 2019 ). Still, some people translate these problems to probability format instead of using natural frequencies to solve them. Moreover, many students fail to obtain the correct solution for Bayesian tasks when data are in natural frequency format (Budgett & Pfannkuch, 2019 ; Weber et al., 2018 ).
In this section, we describe research using different visualisation resources to help overcome these problems (see Fig. 2 for the tools and variables analysed). We distinguish three components of Bayesian reasoning: (a) interpreting the problem data, (b) performance and strategies in computing a conditional probability, and (c) applying the results to inductive reasoning or decision-making. Böcherer-Linder et al. ( 2022 ) add the ability to appropriately communicate probabilistic information in a Bayesian situation, in an expert-laymen setting, such as doctor–patient.
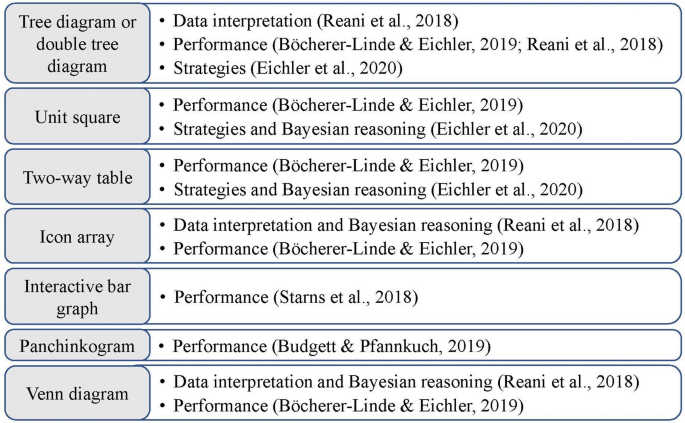
Visualization tools and variables investigated by different authors
Due to technological advances, both static and dynamic visual representations of probability are gaining interest in mathematics education (Budgett & Pfannkuch, 2019 ). These tools include Venn diagrams, two-way tables, tree and double tree diagrams, icon arrays, and unit square graphs, each preferable for representing joint or conditional probabilities. Böcherer-Linder and Eichler ( 2019 ) analysed the performance of undergraduate students in Bayesian problems with the above tools. Their results showed additional benefits in representing discrete objects (icon array), although not in displaying area proportionality (unit square representation). Undergraduate strategies and faulty procedures in Bayesian tasks depend on the visualization used. Thus, the displays that make the nested-sets structure of a Bayesian task transparent (tree diagrams) facilitate students’ Bayesian reasoning. However, compared to a unit square, a tree diagram partly hinder people from finding the correct denominator in a Bayesian problem (Eichler et al., 2020 ).
Budgett and Pfannkuch ( 2019 ) proposed a pachinkogram , a dynamic and interactive version of a tree diagram with a unit square graph added to it. Using this tool, students can vary the problem data, such as the base rate, and observe the result in both representations (tree and unit square). This tool allowed undergraduate students to make conjectures when solving a Bayesian problem and testing them using simulation. In simulating the problem, the ability to vary the branch widths of the pachinkogram may convey the impact of the base rate. Furthermore, the representation afforded by the pachinkogram helped clarify the distinction between a conditional probability and its inverse (Budgett & Pfannkuch, 2019 ).
Different techniques for representing conditional probabilities may impact students' understanding and use of visualization to interpret the data. In Reani et al. ( 2018 ), Venn diagrams were similar to tree diagrams in task performance, although the latter was easier to interpret; moreover, participants perceived icon arrays to be poor at conveying information about conditional probabilities.
One objective of instruction in Bayesian reasoning should be to learn strategies that are easy to apply in everyday settings. To achieve this aim, Starns et al. ( 2019 ) used an interactive bar graph to represent the odds involved in the problem. The method substitutes Bayes’ formula, which is difficult for students. Furthermore, odds carry the same information as probability and can be related to familiar contexts such as gambling or medical diagnosis. A short training with the new tool improved problem-solving performance to a great extent. Afterwards, participants in the study applied the technique adequately using interactive tools and with only paper and pencil.
7 Probability modelling
The teaching and learning of modelling are inextricably linked to those of mathematics, which can be never isolated from the extra-mathematical domain, and mathematical work always involves modelling (Niss & Blum, 2020 ). Probability is not an exception, as the French Commission inter-IREM Statistique et Probabilités proposed modelling at the beginning of the century to help students connect the classical and frequentist views of probability (Henry, 2001 ).
In this perspective, probability is considered a theoretical value representing the likelihood of a random outcome. We can obtain this theoretical probability a priori in the classical approach, estimate it a posteriori from its relative frequencies (frequentist view), or assigned and updated it subjectively (subjectivist view) (Batanero & Borovcnik, 2016 ; Chaput et al., 2011 ). Moreover, the probability distributions of observable characteristics in a population can be interpreted as models of the data observed in reality (samples from these populations). This connection between the classical and frequentist views of probability is also essential in informal statistical inference (ISI) (Pfannkuch et al., 2018 ), which is today recommended to introduce inference.
Makar and Rubin ( 2018 ) describe ISI as producing claims beyond the data at hand, recognising the uncertainty involved, using data to support these claims, and considering the context. ISI could be taught with little formalization to diminish the probability content required (Borovcnik, 2022 ). However, a good understanding of ISI depends on students’ knowledge of concepts such as population, distribution, sample, sampling distribution and independence. Consequently, teachers should help their students understand these concepts (Lee, 2018 ).
Often the model that represents a chance situation can be simulated in a computer, so running the simulation many times help students explore the long-term behaviour of their model (Kazak & Pratt, 2021 ). This fact led to different approaches to statistical inference based on repeated sampling simulation (Batanero & Borovcnik, 2016 ).
All the above ideas have been developed in recent years (see Pfannkuch et al., 2018 , for a survey). For example, Eichler and Vogel ( 2014 ) analysed probability modelling and teaching situations in the classical, frequentist and subjectivist views of probability. Two relevant initiatives were the thematic issues on Reasoning about Models and Modelling in the Context of Informal Statistical Inference (Statistics Education Research Journal, 1917) and Innovations in Statistical Modelling (ZDM—Mathematics 2018). Conclusions of papers dealing with simulation and modelling include:
Even primary school children can create simulated statistical models related to real data with appropriate teaching and software (Ainsley & Pratt, 2017 ; Aridor & Ben-Zvi, 2017 ).
Promoting aggregate reasoning (reasoning with global characteristics of a group of data) is crucial to understand concepts, such as sampling distribution. Although this type of reasoning is difficult, it can be fostered with adequate simulation activities (Aridor & Ben-Zvi, 2017 , 2018 ).
Simulation helps undergraduate students better understand the link between different theoretical distributions (e.g., the Poisson and exponential distributions) and gain a deeper comprehension of distribution and randomness (Budgett & Pfannkuch, 2019 ).
In teacher education, modelling activities can help develop both their mathematical content and pedagogical knowledge (Biehler et al., 2018 ).
8 Education of teachers
In the past decades, research centred on mathematics teachers' education has increased enormously. Different theoretical models have been suggested to organise this research and coincide that teachers need common mathematical knowledge related to the level of education where he teaches and advanced knowledge of the mathematical content that allows the teacher to articulate this knowledge with the teaching of the topic in higher educational levels. In addition, the teacher needs didactic knowledge conceptualized in the different theoretical frameworks. The Didactic-Mathematical Knowledge (DMK) model (Pino-Fan et al., 2015 ) considers the following facets: epistemic (specialized mathematical knowledge or mathematical knowledge specific to teaching), ecological (knowing the relation of the subject with other themes in the curriculum and with society), cognitive (understanding the students' learning, difficulties and reasoning), affective (managing students and own attitudes, beliefs and emotions), mediational (familiarity with teaching resources and technology) and interactional (managing the classroom discourse).
In this section, we summarise recent advances in each component of DMK for teaching probability (see Fig. 3 ). Subjects in the sample include elementary and middle school prospective teachers (PTs) (Abu-Ghalyoun, 2021 ; Alonso-Castaño et al., 2021 ; Hourigan & Leavy, 2020 ), mathematics and science secondary school PTs (Batanero et al., 2022 ; Gravir, 2019 ; Ingram, 2022 ), in-service primary school teachers (Grando & Lopes, 2020 ; Malaspina & Malaspina, 2020 ) and in-service high school teachers (Martin et al., 2022 ; Salinas-Herrera & Salinas-Hernández, 2022 ).
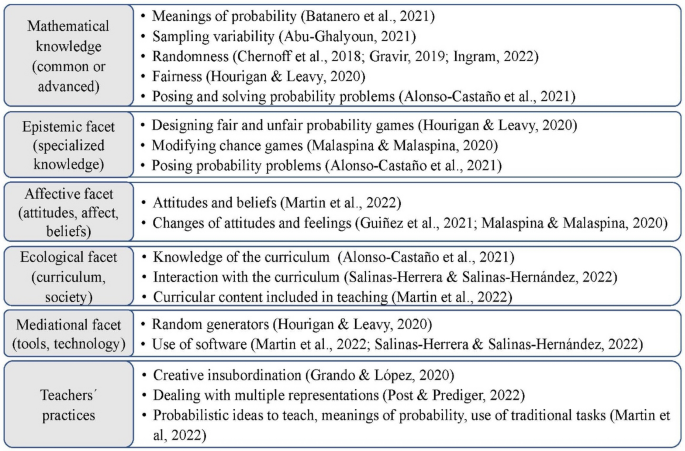
Component of teacher’ knowledge and competence and topics investigated
Studies dealing with mathematical knowledge to teach probability have centred on understanding and reasoning about randomness and random processes (Chernoff et al., 2018 ; Gravir, 2019 ; Ingram, 2022 ), relating the classical and frequentist probability in sampling (Batanero et al., 2022 ), fairness (Hourigan & Leavy, 2020 , posing and solving probability problems (Alonso-Castaño et al., 2021 ), the effect of the sample size on sampling variability and the confidence of the inferences based on these samples (Abu-Ghalyoun, 2021 ). This research points to mathematical difficulties in part of PTs, particularly in primary school PTs.
Primary school PTs can work with mathematical processes such as problem-posing and solving, communication, and argumentation (Alonso-Castaño et al., 2021 ) and demonstrate an understanding of probabilistic fairness (Hourigan & Leavy, 2020 ). However, many are unfamiliar with the various meanings of randomness and probability, and some do not perceive the independence of trials (Ingram, 2022 ) or present biases such as representativeness (Hourigan & Leavy, 2020 ) or the fallacy of composition (Chernoff et al., 2018 ).
When asked to generate a binary sequence of 40 symbols appearing as random as possible, secondary school PTs underestimated the number of runs with four or more identical symbols and produced too many short runs (Gravir, 2019 ). Although they built an urn model consistent with frequentist data, a large proportion of secondary school PTs forgot the previously constructed urn model, using only the frequency data to estimate the probability of new extractions (Batanero et al., 2022 ). In both PTs, some associated randomness with equiprobable outcomes (Batanero et al., 2022 ; Hourigan & Leavy, 2020 ; Ingram, 2022 ). These difficulties might not necessarily arise from a lack of expertise but because of the activation of less-productive knowledge elements over others (Abu-Ghalyoun, 2021 ).
Several studies used creative tasks to mix the evaluation of teachers’ mathematical knowledge with their didactical knowledge. These tasks included creating and solving probability problems for a teaching level (Alonso-Castaño et al., 2021 ), inventing and modifying games (Malaspina & Malaspina, 2020 ), designing fair and unfair activities for primary school (Hourigan & Leavy, 2020 ), and planning a teaching experience on the normal distribution (Salinas-Herrera & Salinas-Hernández, 2022 ).
Concerning the epistemic facet (specialized knowledge), primary school PTs successfully constructed fair and unfair games for three or two different random generators (Hourigan & Leavy, 2020 ). Some of them, however, lacked specialized mathematical knowledge when proposing problems for a given school level because they posed tasks that either did not correspond to specific content or were inadequate for the students' age (Alonso-Castaño et al., 2021 ). Specialized knowledge was better in in-service primary school teachers, who successfully adapted games of chance to be used in teaching (Malaspina & Malaspina, 2020 ).
As regards the affective facet , all in-service teachers in Martin et al. ( 2022 ) agreed on the social usefulness of probability and the interest in their students' education. Modifying a chance game helped in-service teachers strengthen their creativity, self-efficacy, self-esteem, ability to ask questions, and enjoyment of learning (Malaspina & Malaspina, 2020 ). A positive change in attitude and feelings towards probability and its teaching was also observed in primary school PTs after working with an interactive storybook (Guiñez et al., 2021 ).
As for the ecological facet, there was a good interaction with the curriculum of in-service teachers when working together to design and analyse a teaching experience of the normal distribution (Salinas-Herrera & Salinas-Hernández, 2022 ). Additionally, in-service teachers in Martin et al. ( 2022 ) agreed in the relevance of the theoretical approach to probability, the main probabilistic concepts to teach and the use of traditional tasks. However, there was little knowledge of the curriculum in primary school PTs (Alonso-Castaño et al., 2021 ).
About the knowledge of tools for teaching probability ( mediational facet ), playing cards, dice and coins were the random generators presenting more challenges for PTs (Hourigan & Leavy, 2020 ). Regarding in-service secondary school teachers, although some were unfamiliar with the software and sceptical about its role before teaching, their views changed after a teaching experience (Salinas-Herrera & Salinas-Hernández, 2022 ). Teachers used various tasks, manipulative resources and technology to exploit the frequentist approach and there was ample agreement on the added value of technological tools for exploration and amplification; however, they used different resources and the same tools in distinct ways (Martin et al., 2022 ). Biehler et al. ( 2018 ) also analysed simulation activities, which served to educate the teachers mathematical and technological knowledge.
Other research focussed on the teaching practices of in-service teachers when teaching probability. Thus, teachers in Martin et al. ( 2022 )’ research introduced the frequentist approach to their students, although how they performed this introduction and the situations, they proposed to the students varied. There were distinct ways to connect the different views of probability, and the subjectivist one only occupied a marginal place in the curriculum. Post and Prediger ( 2022 ) analysed the teachers’ practices when dealing with multiple representations in teaching conditional probability. Translating concepts of a given text into other representations (visualization, symbolism, language) was sufficient for some students. Other needed support for unfolding the compacted concepts into several concept elements and explicitly connecting them in multiple representations. Grando and Lopes ( 2020 ) studied creative insubordination, which describes professionals who act counter to rules and directives when seeking to protect those to whom they provide services. They concluded that creatively insubordinate teachers provide an investigative atmosphere in which children discuss, question and reflect, proposing creative solutions to statistical problems and making learning more significant for children.
9 Future directions
The research tendencies in probability education in the past few years described in the survey help identify topics where the investigation is still scarce. A limitation of this research is that it has mainly focussed on the classical and frequentist views of probability, while subjective probability and the modelling approach deserve more attention.
We found scarce epistemological and curricular analyses. Possible new topics are finding ways to introduce the relationships between risk and probability in the classrooms (Borovnick & Kapadia, 2018 ) and investigating how the relationships between probability and other areas can reinforce interdisciplinary work in the school (Carrera et al., 2021 ). Analysis of textbooks and probability tasks proposed in assessment tests in different countries, like that of Batanero et al. ( 2018 ) is another topic of interest.
Research on probabilistic reasoning in kindergarten and primary school children suggests they can develop ideas about uncertainty and probability (e.g., Nikiforidou, 2019 ; Vásquez & Alsina, 2019 ), from the classical, frequentist and subjectivist perspectives (Kazak & Leavy, 2018 , 2022 ; Nilsson et al., 2018 ). We should complement this research with more children and variation in tasks and contexts to ensure the generalizability of findings. Since most studies reduce to the classical view and subjectivist or frequentist probability are introduced early in some countries (Langrall, 2018 ), further research is needed to investigate how we should work other approaches with children.
Even when research on students’ intuitions and learning has a long history, changes in curricular contents and the impact of technology predict that this investigation will continue in the next years. Given the dependence of probabilistic reasoning on the ideas of randomness, sample space, comparison of probabilities and correlation (Bryant & Nunes, 2012 ), further research is missing to investigate how students at different school levels understand these topics and which is the best teaching to help them overcome their reasoning biases. Because many probability ideas depend on proportional reasoning (Jones & Thornton, 2005 ; Nikiforidou, 2018 ), these studies should also relate the students’ understanding to their proportional reasoning levels.
In some experiences, simulation was insufficient to promote relevant ideas, for example, convergence (Salinas-Herrera & Salinas-Hernández, 2022 ). Thus, the complementary role of technology and other materials to solve these problems needs more investigation. More cross-cultural studies concerning understanding probability in different cultural contexts, such as that by Morris ( 2021 ), will also be welcome. Moreover, research on probability modelling has mainly been linked to informal approaches to inference and not specifically to probability (Pfannkuch et al., 2018 ). More investigation is needed to analyse students' modelling activities when working with probability distributions (Kazak & Pratt, 2021 ).
Although there is much research on different visualization techniques, it has only considered the effectiveness of these tools in conditional probability and Bayesian problems (Budgett & Pfannkuch, 2019 ; Eichler et al., 2020 ). We lack papers investigating how visualization tools improve the study of other probability notions, such as comparing probabilities, random variables and distributions, or sampling. More research is also needed on students’ challenges and learning processes when working simultaneously with multiple representations and how they connect them (Post & Prediger, 2022 ). Because students need Bayesian reasoning in out-of-school contexts (Starns et al., 2019 ), we should design and analyse teaching tasks based on interpreting these situations; for example, taken from the media news. Research on using probability education to prevent gambling-related distortions (Primi & Donati, 2022 ) and to improve the ability to communicate probability information should also be pursued (Böcherer-Linder et al., 2022 ). Since many students do not correctly use natural frequencies to solve Bayesian tasks, the extent to which implementing natural frequencies is reasonable and the degree to which teachers are familiar with the concept is another topic (Weber et al., 2018 ).
As exposed previously, successful teaching of probability requires adequate preparation of teachers. However, we still miss research on their mathematical knowledge of many probability concepts. Teachers’ attitudes and beliefs towards teaching probability also need new investigation as the teachers’ emotions are often transmitted to their students (Guiñez et al, 2021 ). Moreover, there are still very few papers centred on the cognitive and interactional components of their didactic knowledge (Pino-Fan et al., 2015 ): how teachers conceive their students’ learning, predict their difficulties and strategies, and instructional practices to overcome these problems. As for the mediational facet, an interesting line of work is analysing the teachers’ practice when working on concepts, such as conditional probability with multiple representations (Post & Prediger, 2022 ). There are also scarce studies on the teachers’ technological knowledge to teach probability, and most of these works only consider specific software.
Papers published within the period of review, have been highlighted as: * included in the review; ** of special interest, annotated
*Abu-Ghalyoun, O. (2021). Pre-service teachers’ difficulties in reasoning about sampling variability. Educational Studies in Mathematics, 108 (3), 553–577. https://doi.org/10.1007/s10649-021-10067-8
Article Google Scholar
*Alonso-Castaño, M., Alonso, P., Mellone, M., & Rodríguez-Muñiz, L. J. (2021). What mathematical knowledge do prospective teachers reveal when creating and solving a probability problem? Mathematics, 9 (24), 3300. https://doi.org/10.3390/math9243300
Ainley, J., & Pratt, D. (2017). Computational modelling and children’s expressions of signal and noise. Statistics Education Research Journal, 16 (2), 15–37. https://doi.org/10.52041/serj.v16i2.183
Aridor, K., & Ben-Zvi, D. (2017). The co-emergence of aggregate and modelling reasoning. Statistics Education Research Journal, 16 (2), 38–63. https://doi.org/10.52041/serj.v16i2.184
*Aridor, K., & Ben-Zvi, D. (2018). Statistical modeling to promote students’ aggregate reasoning with sample and sampling. ZDM, 50 , 1165–1181. https://doi.org/10.1007/s11858-018-0994-5
Australian Curriculum, Assessment and Reporting Authority (ACARA). (2020). The Australian curriculum: Mathematics. ACARA.
*Batanero, C., Álvarez-Arroyo, R., Hernández-Solís, L. A., & Gea, M. M. (2021). El inicio del razonamiento probabilístico en la educación infantil (Beginning of probabilistic reasoning in pre-school education). PNA, 15 (4), 267–288. https://doi.org/10.30827/pna.v15i4.22349
*Batanero, C., Begué, N., & Ortiz, J. J. (2022). Prospective teachers’ probabilistic reasoning when solving a sampling task. In S. A. Peters, L. Zapata-Cardona, F. Bonafini, & A. Fan (Eds.), Proceedings of the 11th international conference on teaching statistics. IASE. http://iase-web.org/Conference_Proceedings.php?p=ICOTS_11_2022 . Accessed 1 July 2023.
Batanero, C., & Borovcnik, M. (2016). Statistics and probability in high school . Sense Publishers.
Book Google Scholar
Batanero, C., & Chernoff, E. (2018). Teaching and learning stochastics. Advances in probability education research. ICME-13 monographs . Springer.
Google Scholar
Batanero, C., Chernoff, E., Engel, J., Lee, H., & Sánchez, E. (2016). Research on teaching and learning probability . Springer. https://doi.org/10.1007/978-3-319-31625-3_1
*Batanero, C., López-Martín, M. M., Arteaga, P., & Gea, M. M. (2018a). Characterizing probability problems posed in university entrance tests in Andalucia. In C. Batanero & E. Chernoff (Eds.), Teaching and learning stochastics. Advances in probability education research (pp. 103–123). Springer. https://doi.org/10.1007/978-3-319-72871-1_7
Chapter Google Scholar
*Batista, R., Borba, R., & Henriques, A. (2022). Fairness in games: A study on children’s and adults’ understanding of probability. Statistics Education Research Journal, 21 (1), 1–15. https://doi.org/10.52041/serj.v21i1.79
*Beckman, M. D., & delMas, R. (2018). Statistics students’ identification of inferential model elements within contexts of their own invention. ZDM, 50 , 1295–1309. https://doi.org/10.1007/s11858-018-0986-5
*Biehler, R., Frischemeier, D., & Podworny, S. (2018). Elementary preservice teachers’ reasoning about statistical modeling in a civic statistics context. ZDM, 50 , 1237–1251. https://doi.org/10.1007/s11858-018-1001-x
*Böcherer-Linder, K., & Eichler, A. (2019). How to improve performance in Bayesian inference tasks: A comparison of five visualizations. Frontiers in Psychology, 10 , 267. https://doi.org/10.3389/fpsyg.2019.00267
*Böcherer-Linder, K., Binder, K., Büchter, T., Eichler, A., Krauss, S., Steib, N., & Vogel, M. (2022). Communicating conditional probabilities in medical practice. In S. A. Peters, L. Zapata-Cardona, F. Bonafini, & A. Fan (Eds.), Proceedings of the 11th international conference on teaching statistics. IASE. http://iase-web.org/Conference_Proceedings.php?p=ICOTS_11_2022 . Accessed 1 July 2023.
*Borovcnik, M. (2021). Mutual influence between different views of probability and statistical inference. Paradigma, 42 (Extra1), 221–256. https://doi.org/10.37618/PARADIGMA.1011-2251.2021.p221-256.id1024
*Borovcnik, M. (2022). The role of probability for understanding statistical inference. In S. A. Peters, L. Zapata-Cardona, F. Bonafini, & A. Fan (Eds.), Proceedings of the 11th international conference on teaching statistics . IASE http://iase-web.org/Conference_Proceedings.php?p=ICOTS_11_2022 . Accessed 1 July 2023.
*Borovcnik, M., & Kapadia, R. (2018). Reasoning with risk: Teaching probability and risk as twin concepts. In C. Batanero & E. Chernoff (Eds.), Teaching and learning stochastics. Advances in probability education research (pp. 3–22). Springer. https://doi.org/10.1007/978-3-319-72871-1_1
Borovcnik, M., & Peard, R. (1996). Probability. In A. Bishop, M. A. Clements, C. Keitel, J. Kilpatrick, & C. Laborde (Eds.), International handbook of mathematics education (pp. 239–288). Kluwer.
Bryant, P., & Nunes, T. (2012). Children’s understanding of probability: A literature review . Nuffield Foundation.
*Bryant, P., Nunes, T., Evans, D., Barros, R., Gottardis, L., & Terlektsi, E. (2018b). What 9- and 10-year old pupils already know and what they can learn about randomness. In C. Batanero & E. Chernoff (Eds.), Teaching and learning stochastics. Advances in probability education research (pp. 161–179). Springer. https://doi.org/10.1007/978-3-319-72871-1_10
*Budgett, S., & Pfannkuch, M. (2019). Visualizing chance Tackling conditional probability misconceptions. In G. Burrill & D. Ben-Zvi (Eds.), Topics and trends in current statistics education research (pp. 3–25). Springer. https://doi.org/10.1007/978-3-030-03472-6_1
*Carrera, P. B., Pino-Fan, L. R., Alvarado, H., & Lugo-Armenta, J. G. (2021). Practices of the random variable proposed in the Chilean mathematics curriculum of secondary education. Mathematics, 9 (19), 2435. https://doi.org/10.3390/math9192435
Chaput, B., Girard, J. C., & Henry, M. (2011). Frequentist approach: Modelling and simulation in statistics and probability teaching. In C. Batanero, G. Burrill, & C. Reading (Eds.), Teaching statistics in school mathematics-challenges for teaching and teacher education (pp. 85–95). Springer.
Chernoff, E. J., & Sriraman B. (2014) (Eds.). Probabilistic thinking: Presenting plural perspectives. Springer. https://doi.org/10.1007/978-94-007-7155-0
*Chernoff, E. J., Vashchyshyn, I., & Neufeld, H. (2018). Comparing the relative probabilities of events. In C. Batanero & E. Chernoff (Eds.), Teaching and learning stochastics. Advances in probability education research (pp. 277–291). Springer. https://doi.org/10.1007/978-3-319-72871-1_16
*Eichler, A., Böcherer-Linder, K., & Vogel, M. (2020). Different visualizations cause different strategies when dealing with Bayesian situations. Frontiers in Psychology, 11 , 1897. https://doi.org/10.3389/fpsyg.2020.01897
Eichler, A., & Vogel, M. (2014). Three approaches for modelling situations with ran-domness. In E. Chernoff & B. Sriraman (Eds.), Probabilistic thinking: Presenting plural perspectives (pp. 75–99). Springer.
Fischbein, E. (1975). The intuitive source of probability thinking in children . Reidel.
Gilovich, T., Griffin, D., & Kahneman, D. (2002). Heuristics and biases: The psychology of intuitive judgment . Cambridge University Press.
*Grando, R. C., & Lopes, C. E. (2020). Creative insubordination of teachers proposing statistics and probability problems to children. ZDM, 52 (4), 621–635. https://doi.org/10.1007/s11858-020-01166-6
*Gravir, O. (2019). Testing the negative recency effect among teacher students trying to generate random sequences. In U.T. Jankvist, M. Van den Heuvel-Panhuizen, & M. Veldhuis, (Eds.). Proceedings of the eleventh congress of the European Society for Research in Mathematics Education. Freudenthal Group and Freudenthal Institute, Utrecht University and ERME. https://hal.archives-ouvertes.fr/hal-02411594 . Accessed 1 July 2023.
**Groth, R. E., Austin, J. W., Naumann, M., & Rickards, M. (2021). Toward a theoretical structure to characterize early probabilistic thinking. Mathematics Education Research Journal, 33 , 241–261. https://doi.org/10.1007/s13394-019-00287-w . Presents a model to characterise the structure of early probabilistic thinking (SEPT), attending children’s probabilistic reasoning with two components: One supporting normative thinking and compatible with mathematical norms; another based on beliefs that conflict with normative mathematics. The SEPT framework provides a basis for further curricular development and research.
*Groth, R. E., Bergner, J. A., & Austin, J. W. (2020). Dimensions of learning probability vocabulary. Journal for Research in Mathematics Education, 51 (1), 75–104. https://doi.org/10.5951/jresematheduc.2019.0008
*Guiñez, F., Vásquez, C., Brito, C., & Martínez, S. (2021). Alice in randomland: A resource for improving attitudes towards probability and its teaching. Statistics Education Research Journal, 12 (2), Article 14. https://doi.org/10.52041/serj.v20i2.410
Henry, M. (2001) (Coord). Autour de la modélisation en probabilités. Presses Universitaires de Franche-Comté.
*Hourigan, M., & Leavy, A. M. (2020). Pre-service teachers’ understanding of probabilistic fairness: Analysis of decisions around task design. International Journal of Mathematical Education in Science and Technology, 51 (7), 997–1019. https://doi.org/10.1080/0020739X.2019.1648891
*Ingram, J. (2022). Randomness and probability: exploring student teachers’ conceptions. Mathematical Thinking and Learning . https://doi.org/10.1080/10986065.2021.2016029 . ahead of print.
Jones, G. A. (2005). Exploring probability in school . Springer. https://doi.org/10.1007/0-387-24530-8_4
Jones, G., Langrall, C., & Mooney, E. (2007). Research in probability: responding to classroom realities. In F. Lester (Ed.), Second handbook of research on mathematics teaching and learning. Information Age Publishing and NCTM.
Jones, G., & Thornton, C. (2005). An overview of research into the teaching and learning of probability. In G. Jones (Ed.), Exploring probability in school. Challenges for teaching and learning. Mathematics education library (pp. 65–92). Springer. https://doi.org/10.1007/0-387-24530-8_4
Kapadia, R., & Borovcnik, M. (1991). Chance encounters. Probability in education . Kluwer. https://doi.org/10.1007/978-94-011-3532-0
*Kaplar, M., Lužanin, Z., & Verbić, S. (2021). Evidence of probability misconception in engineering students—why even an inaccurate explanation is better than no explanation. International Journal of STEM Education , 8 (18), 1–15. https://doi.org/10.1186/s40594-021-00279
*Kazak, S., & Leavy, A. M. (2018). Emergent reasoning about uncertainty in primary school children with a focus on subjective probability. In A. Leavy, M. Meletiou-Mavrotheris, & E. Paparistodemou (Eds.), Statistics in early childhood and primary education. Early mathematics learning and development series (pp. 34–54). Springer. https://doi.org/10.1007/978-981-13-1044-7_3
*Kazak, S., & Leavy, A. (2022). The emerging interplay between subjective and objective notions of probability in young children. Canadian Journal of Science, Mathematics and Technology Education, 22 , 538–557. https://doi.org/10.1007/s42330-022-00227-0
**Kazak, S., & Pratt, D. (2021). Developing the role of modelling in the teaching and learning of probability. Research in Mathematics Education, 23 (2), 113–133. https://doi.org/10.1080/14794802.2020.1802328 . Students easily confuse real-world and model-generated data and tend to create models that reflect the structure of the problem context rather than its mathematical structure. This paper analyses the role of an integrated modelling approach to facilitate understanding the connections of data and probability distributions and avoid these problems.
*Langrall, C. W. (2018c). The status of probability in the elementary and lower secondary school mathematics curriculum: The rise and fall of probability in school mathematics in the United States. In C. Batanero & E. Chernoff (Eds.), Teaching and learning stochastics. Advances in probability education research (pp. 39–50). Springer. https://doi.org/10.1007/978-3-319-72871-1_3
*Leavy, A., Meletiou-Mavrotheris, M., & Paparistodemou, E. (2018). Statistics in early childhood and primary education . Springer. https://doi.org/10.1007/978-981-13-1044-7
*Lee, H. S. (2018). Probability concepts needed for teaching a repeated sampling approach to inference. In C. Batanero & E. Chernoff (Eds.), Teaching and learning stochastics. Advances in probability education research (pp. 89–101). Springer. https://doi.org/10.1007/978-3-319-72871-1_6
*Martin, V., Thibault, M., & Homier, M. (2022). Convergence and divergence in probability teaching in primary and secondary school in Québec: A closer look at eight teachers’ self-reported practices. Canadian Journal of Science, Mathematics, and Technology Education, 22 , 659–678. https://doi.org/10.1007/s42330-022-00241-2
*Makar, K., & Rubin, A. (2018). Learning about statistical inference. In D. Ben-Zvi, K. Makar, & J. Garfield (Eds.), International handbook of research in statistics education (pp. 261–294). Springer. https://doi.org/10.1007/978-3-319-66195-7_8
*Malaspina, M., & Malaspina, U. (2020). Game invention as means to stimulate probabilistic thinking. Statistics Education Research Journal, 19 (1), 57–72. https://doi.org/10.52041/serj.v19i1.119
Ministerio de Educación y Formación Profesional. MEFP (2022). Real Decreto 157/2022, de 1 de marzo, por el que se establecen la ordenación y las enseñanzas mínimas de la Educación Primaria (Royal decree establishing the content of primary education) . MEFP. https://www.boe.es/eli/es/rd/2022/03/01/157 . Accessed 1 July 2023.
**Morris, N. (2021). Learning probability in the Kingdom of Tonga: The influence of language and culture. Educational Studies in Mathematics, 107 , 111–134. https://doi.org/10.1007/s10649-020-10022-z . This qualitative study investigates the relationship between cultural norms, languages and thinking in understanding probability and uncertainty in Tonga. It was motivated by students' unusual difficulties in probability compared with other mathematical topics. This investigation contributes new knowledge to cross-cultural studies concerning understanding probability in different cultural contexts.
*Nikiforidou, Z. (2018). Probabilistic thinking and young children: Theory and pedagogy. In A. Leavy, M. Meletiou-Mavrotheris, & E. Paparistodemou (Eds.), Statistics in early childhood and primary education (pp. 21–34). Springer. https://doi.org/10.1007/978-981-13-1044-7_3
*Nikiforidou, Z. (2019). Probabilities and preschoolers: Do tangible versus virtual manipulatives, sample space, and repetition matter? Early Childhood Education Journal, 47 , 769–777. https://doi.org/10.1007/s10643-019-00964-2
**Nilsson, P., Eckert, A., & Pratt, D. (2018). Challenges and opportunities in experimentation-based instruction in probability. In C. Batanero & E. Chernoff (Eds.), Teaching and learning stochastics. Advances in probability education research (pp. 51–71). Springer. https://doi.org/10.1007/978-3-319-72871-1_4 . This study examines experimentation-based instruction in learning the bi-directional relationship between a classical and frequentist probability with 11–12-year-olds. The authors base on inferentialism to conceptualize meaning-making in an interactive probability classroom. The teaching dilemmas that teachers face in this perspective are: hiding or not deterministic issues, inviting versus challenging interactive teaching and known or unknown sample space.
Niss, M., & Blum, W. (2020). The learning and teaching of mathematical modelling . Routledge.
**Pfannkuch, M., Ben-Zvi, D., & Budgett, S. (2018). Innovations in statistical modelling to connect data, chance and context. ZDM, 50 (7), 1113–1123. https://doi.org/10.1007/s11858-018-0989-2 . This paper analyses a shift towards a modelling perspective in statistics education research, and how modelling is interpreted in this field. It also discusses frameworks being developed to understand student reasoning with modelling and statistical models.
Piaget, J., & Inhelder, B. (1951). La genèse de l’idée de hasard chez l’enfant (The origin of the idea of chance in children) . Presses Universitaires de France.
Pino-Fan, L. R., Assis, A., & Castro, W. F. (2015). Towards a methodology for the characterization of teachers’ didactic-mathematical knowledge. EURASIA Journal of Mathematics, Science and Technology Education, 11 (6), 1429–1456. https://doi.org/10.12973/eurasia.2015.1403a
*Post, M., & Prediger, S. (2022). Teaching practices for unfolding information and connecting multiple representations: the case of conditional probability information. Mathematics Education Research Journal . https://doi.org/10.1007/s13394-022-00431-z
*Pratt, D., & Kazak, S. (2018). Research on uncertainty. In D. Ben-Zvi, K. Makar, & J. Garfield (Eds.), International handbook of research in statistics education (pp. 193–227). Springer. https://doi.org/10.1007/978-3-319-66195-7_6
**Primi, C., & Donati, M. A. (2022). The prevention of adolescent problem gambling through probabilistic reasoning: Evidence of the intervention’s efficacy. Canadian Journal of Science, Mathematics, and Technology Education, 22 , 591–601. https://doi.org/10.1007/s42330-022-00229-y . The individuals’ tendency to reasoning and judgment biases makes them particularly vulnerable to cognitive distortions related to gambling. To prevent this problem in adolescents, the authors developed and evaluated a teaching intervention that helped the students reinforce probabilistic rules and knowledge and recognize biases in reasoning with randomness.
*Reani, M., Davies, A., Peek, N., & Jay, C. (2018). How do people use information presentation to make decisions in Bayesian reasoning tasks ? . International Journal of Human- Computer Studies, 111 , 62–77. https://doi.org/10.1016/j.ijhcs.2017.11.004
Rezat, S., Fan, L., & Pepin, B. (2021). Mathematics textbooks and curriculum resources as instruments for change. ZDM, 53 , 1189–1206. https://doi.org/10.1007/s11858-021-01309-3
*Salinas-Herrera, J., & Salinas-Hernández, U. (2022). Teaching and learning the notion of normal distribution using a digital resource. Canadian Journal of Science, Mathematics, and Technology Education, 22 , 576–590. https://doi.org/10.1007/s42330-022-00226-1
Shaughnessy, J. M. (1992). Research in probability and statistics: Reflections and directions. In D. A. Grouws (Ed.), Handbook of research on mathematics teaching and learning (pp. 465–494). Macmillan.
*Starns, J. J., Cohen, A. L., Bosco, C., & Hirst, J. (2019). A visualization technique for Bayesian reasoning. Applied Cognitive Psychology, 33 , 234–251. https://doi.org/10.1002/acp.3470
*Supply, A.-S., Van Dooren, W., Lem, S., & Onghena, P. (2020). Assessing young children’s ability to compare probabilities. Educational Studies in Mathematics, 103 (1), 27–42. https://doi.org/10.1007/s10649-019-09917-3
**Supply, A.-S., Van Dooren, W., & Onghena, P. (2022). Can we count on early numerical abilities for early probabilistic reasoning abilities? Mathematical Thinking and Learning, 24 (1), 19–37. https://doi.org/10.1080/10986065.2020.1805551 . This paper reports a longitudinal study of children in kindergarten (4-year-olds) and one and two years later. The main conclusion is that children’s early numerical abilities in kindergarten are associated to their later probabilistic reasoning abilities in primary education, supporting Fischbein’s ideas about the role of early instruction in probability.
*Vargas, F., Benincasa, T., Cian, G., & Martignon, L. (2019). Fostering probabilistic reasoning away from fallacies: Natural information formats and interaction between school levels. International Electronic Journal of Mathematics Education, 14 (2), 303–330. https://doi.org/10.29333/iejme/5716
*Vásquez, C., & Alsina, Á. (2019). Intuitive ideas about chance and probability in children from 4 to 6 years old. Acta Scientiae, 21 (3), 131–154. https://doi.org/10.17648/acta.scientiae.v21iss3id5215
*Weber, P., Krauss, S., & Binder, K. (2018). Frequency phobia in spite of probability blindness. In M. A. Sorto, A. White, & L. Guyot (Eds.), Proceedings of the tenth international conference on teaching statistics: IASE. http://iase-web.org/Conference_Proceedings.php?p=ICOTS_10_2018 . Accessed 1 July 2023.
*Zapata-Cardona, L. (2018). Supporting young children to develop combinatorial reasoning. In A. Leavy, M. Meletiou-Mavrotheris, & E. Paparistodemou (Eds.), Statistics in early childhood and primary education (pp. 257–272). Springer. https://doi.org/10.1007/978-981-13-1044-7_15
Download references
Acknowledgements
Project PID2019-105601GB-I00 funded by MCIN/AEI/10.13039/501100011033.
Funding for open access publishing: Universidad de Granada/CBUA.
Author information
Authors and affiliations.
University of Granada, Granada, Spain
Carmen Batanero & Rocío Álvarez-Arroyo
You can also search for this author in PubMed Google Scholar
Corresponding author
Correspondence to Carmen Batanero .
Additional information
Publisher's note.
Springer Nature remains neutral with regard to jurisdictional claims in published maps and institutional affiliations.
Rights and permissions
Open Access This article is licensed under a Creative Commons Attribution 4.0 International License, which permits use, sharing, adaptation, distribution and reproduction in any medium or format, as long as you give appropriate credit to the original author(s) and the source, provide a link to the Creative Commons licence, and indicate if changes were made. The images or other third party material in this article are included in the article's Creative Commons licence, unless indicated otherwise in a credit line to the material. If material is not included in the article's Creative Commons licence and your intended use is not permitted by statutory regulation or exceeds the permitted use, you will need to obtain permission directly from the copyright holder. To view a copy of this licence, visit http://creativecommons.org/licenses/by/4.0/ .
Reprints and permissions
About this article
Batanero, C., Álvarez-Arroyo, R. Teaching and learning of probability. ZDM Mathematics Education 56 , 5–17 (2024). https://doi.org/10.1007/s11858-023-01511-5
Download citation
Accepted : 13 July 2023
Published : 04 August 2023
Issue Date : February 2024
DOI : https://doi.org/10.1007/s11858-023-01511-5
Share this article
Anyone you share the following link with will be able to read this content:
Sorry, a shareable link is not currently available for this article.
Provided by the Springer Nature SharedIt content-sharing initiative
- Probability
- Teaching and learning
- Recent research
- Find a journal
- Publish with us
- Track your research

IMAGES
VIDEO
COMMENTS
Probability Theory and Related Fields is a journal dedicated to publishing research papers in modern probability theory and its various fields of application. Publishes research papers related to analysis, geometry and other areas in mathematics.
Journal of Theoretical Probability is a multidisciplinary journal publishing high-quality, original papers in all areas of probability theory. Covers all areas of probability theory, including probability on semigroups, groups, vector spaces, and random matrices.
Journal of Probability and Statistics is an open access journal publishing papers on the theory and application of probability and statistics that consider new methods and approaches to their implementation, or report significant results for the field.
The analysis of random phenomena and variable, stochastic processes and modeling. | Explore the latest full-text research PDFs, articles, conference papers, preprints and more on PROBABILITY...
The section Probability and Statistics of Mathematics publishes original contributions that cover recent advances and reviews in the theory and applications of probability and statistics.
With a publication record spanning more than five decades, the Journal of Applied Probability is the oldest journal devoted to the publication of research in the field of applied probability.
Statistics & Probability Letters adopts a novel and highly innovative approach to the publication of research findings in statistics and probability. It features concise articles, rapid publication and broad coverage of the statistics and probability literature.
In this paper, we develop a personal synthesis of the most outstanding research on the teaching and learning of probability in the past years. We conducted a systematic search to examine publications on this topic in mathematics education, statistics education, education, and psychology journals.
The Annals of Applied Probability publishes research papers of the highest quality reflecting the many facets of contemporary applied probability. Primary emphasis is placed on importance and originality.
Aim of this paper is a general definition of probability, of its main mathematical features and the features it presents under particular circumstances. The behavior of probability is linked...