- Subject List
- Take a Tour
- For Authors
- Subscriber Services
- Publications
- African American Studies
- African Studies
- American Literature
- Anthropology
- Architecture Planning and Preservation
- Art History
- Atlantic History
- Biblical Studies
- British and Irish Literature
- Childhood Studies
- Chinese Studies
- Cinema and Media Studies
- Communication
- Criminology
- Environmental Science
- Evolutionary Biology
- International Law
- International Relations
- Islamic Studies
- Jewish Studies
- Latin American Studies
- Latino Studies
- Linguistics
- Literary and Critical Theory
- Medieval Studies
- Military History
- Political Science
- Public Health
- Renaissance and Reformation
- Social Work
- Urban Studies
- Victorian Literature
- Browse All Subjects

How to Subscribe
- Free Trials
In This Article Expand or collapse the "in this article" section Single-Case Experimental Designs
Introduction, general overviews and primary textbooks.
- Textbooks in Applied Behavior Analysis
- Types of Single-Case Experimental Designs
- Model Building and Randomization in Single-Case Experimental Designs
- Visual Analysis of Single-Case Experimental Designs
- Effect Size Estimates in Single-Case Experimental Designs
- Reporting Single-Case Design Intervention Research
Related Articles Expand or collapse the "related articles" section about
About related articles close popup.
Lorem Ipsum Sit Dolor Amet
Vestibulum ante ipsum primis in faucibus orci luctus et ultrices posuere cubilia Curae; Aliquam ligula odio, euismod ut aliquam et, vestibulum nec risus. Nulla viverra, arcu et iaculis consequat, justo diam ornare tellus, semper ultrices tellus nunc eu tellus.
- Action Research
- Ambulatory Assessment in Behavioral Science
- Effect Size
- Mediation Analysis
- Path Models
- Research Methods for Studying Daily Life
Other Subject Areas
Forthcoming articles expand or collapse the "forthcoming articles" section.
- Data Visualization
- Executive Functions in Childhood
- Remote Work
- Find more forthcoming articles...
- Export Citations
- Share This Facebook LinkedIn Twitter
Single-Case Experimental Designs by S. Andrew Garbacz , Thomas R. Kratochwill LAST REVIEWED: 29 July 2020 LAST MODIFIED: 29 July 2020 DOI: 10.1093/obo/9780199828340-0265
Single-case experimental designs are a family of experimental designs that are characterized by researcher manipulation of an independent variable and repeated measurement of a dependent variable before (i.e., baseline) and after (i.e., intervention phase) introducing the independent variable. In single-case experimental designs a case is the unit of intervention and analysis (e.g., a child, a school). Because measurement within each case is conducted before and after manipulation of the independent variable, the case typically serves as its own control. Experimental variants of single-case designs provide a basis for determining a causal relation by replication of the intervention through (a) introducing and withdrawing the independent variable, (b) manipulating the independent variable across different phases, and (c) introducing the independent variable in a staggered fashion across different points in time. Due to their economy of resources, single-case designs may be useful during development activities and allow for rapid replication across studies.
Several sources provide overviews of single-case experimental designs. Barlow, et al. 2009 includes an overview for the development of single-case experimental designs, describes key considerations for designing and conducting single-case experimental design research, and reviews procedural elements, assessment strategies, and replication considerations. Kazdin 2011 provides detailed coverage of single-case experimental design variants as well as approaches for evaluating data in single-case experimental designs. Kratochwill and Levin 2014 describes key methodological features that underlie single-case experimental designs, including philosophical and statistical foundations and data evaluation. Ledford and Gast 2018 covers research conceptualization and writing, design variants within single-case experimental design, definitions of variables and associated measurement, and approaches to organize and evaluate data. Riley-Tillman and Burns 2009 provides a practical orientation to single-case experimental designs to facilitate uptake and use in applied settings.
Barlow, D. H., M. K. Nock, and M. Hersen, eds. 2009. Single case experimental designs: Strategies for studying behavior change . 3d ed. New York: Pearson.
A comprehensive reference about the process of designing and conducting single-case experimental design studies. Chapters are integrative but can stand alone.
Kazdin, A. E. 2011. Single-case research designs: Methods for clinical and applied settings . 2d ed. New York: Oxford Univ. Press.
A complete overview and description of single-case experimental design variants as well as information about data evaluation.
Kratochwill, T. R., and J. R. Levin, eds. 2014. Single-case intervention research: Methodological and statistical advances . New York: Routledge.
The authors describe in depth the methodological and analytic considerations necessary for designing and conducting research that uses a single-case experimental design. In addition, the text includes chapters from leaders in psychology and education who provide critical perspectives about the use of single-case experimental designs.
Ledford, J. R., and D. L. Gast, eds. 2018. Single case research methodology: Applications in special education and behavioral sciences . New York: Routledge.
Covers the research process from writing literature reviews, to designing, conducting, and evaluating single-case experimental design studies.
Riley-Tillman, T. C., and M. K. Burns. 2009. Evaluating education interventions: Single-case design for measuring response to intervention . New York: Guilford Press.
Focuses on accelerating uptake and use of single-case experimental designs in applied settings. This book provides a practical, “nuts and bolts” orientation to conducting single-case experimental design research.
back to top
Users without a subscription are not able to see the full content on this page. Please subscribe or login .
Oxford Bibliographies Online is available by subscription and perpetual access to institutions. For more information or to contact an Oxford Sales Representative click here .
- About Psychology »
- Meet the Editorial Board »
- Abnormal Psychology
- Academic Assessment
- Acculturation and Health
- Action Regulation Theory
- Addictive Behavior
- Adolescence
- Adoption, Social, Psychological, and Evolutionary Perspect...
- Advanced Theory of Mind
- Affective Forecasting
- Affirmative Action
- Ageism at Work
- Allport, Gordon
- Alzheimer’s Disease
- Analysis of Covariance (ANCOVA)
- Animal Behavior
- Animal Learning
- Anxiety Disorders
- Art and Aesthetics, Psychology of
- Artificial Intelligence, Machine Learning, and Psychology
- Assessment and Clinical Applications of Individual Differe...
- Attachment in Social and Emotional Development across the ...
- Attention-Deficit/Hyperactivity Disorder (ADHD) in Adults
- Attention-Deficit/Hyperactivity Disorder (ADHD) in Childre...
- Attitudinal Ambivalence
- Attraction in Close Relationships
- Attribution Theory
- Authoritarian Personality
- Bayesian Statistical Methods in Psychology
- Behavior Therapy, Rational Emotive
- Behavioral Economics
- Behavioral Genetics
- Belief Perseverance
- Bereavement and Grief
- Biological Psychology
- Birth Order
- Body Image in Men and Women
- Bystander Effect
- Categorical Data Analysis in Psychology
- Childhood and Adolescence, Peer Victimization and Bullying...
- Clark, Mamie Phipps
- Clinical Neuropsychology
- Clinical Psychology
- Cognitive Consistency Theories
- Cognitive Dissonance Theory
- Cognitive Neuroscience
- Communication, Nonverbal Cues and
- Comparative Psychology
- Competence to Stand Trial: Restoration Services
- Competency to Stand Trial
- Computational Psychology
- Conflict Management in the Workplace
- Conformity, Compliance, and Obedience
- Consciousness
- Coping Processes
- Correspondence Analysis in Psychology
- Counseling Psychology
- Creativity at Work
- Critical Thinking
- Cross-Cultural Psychology
- Cultural Psychology
- Daily Life, Research Methods for Studying
- Data Science Methods for Psychology
- Data Sharing in Psychology
- Death and Dying
- Deceiving and Detecting Deceit
- Defensive Processes
- Depressive Disorders
- Development, Prenatal
- Developmental Psychology (Cognitive)
- Developmental Psychology (Social)
- Diagnostic and Statistical Manual of Mental Disorders (DSM...
- Discrimination
- Dissociative Disorders
- Drugs and Behavior
- Eating Disorders
- Ecological Psychology
- Educational Settings, Assessment of Thinking in
- Embodiment and Embodied Cognition
- Emerging Adulthood
- Emotional Intelligence
- Empathy and Altruism
- Employee Stress and Well-Being
- Environmental Neuroscience and Environmental Psychology
- Ethics in Psychological Practice
- Event Perception
- Evolutionary Psychology
- Expansive Posture
- Experimental Existential Psychology
- Exploratory Data Analysis
- Eyewitness Testimony
- Eysenck, Hans
- Factor Analysis
- Festinger, Leon
- Five-Factor Model of Personality
- Flynn Effect, The
- Forensic Psychology
- Forgiveness
- Friendships, Children's
- Fundamental Attribution Error/Correspondence Bias
- Gambler's Fallacy
- Game Theory and Psychology
- Geropsychology, Clinical
- Global Mental Health
- Habit Formation and Behavior Change
- Health Psychology
- Health Psychology Research and Practice, Measurement in
- Heider, Fritz
- Heuristics and Biases
- History of Psychology
- Human Factors
- Humanistic Psychology
- Implicit Association Test (IAT)
- Industrial and Organizational Psychology
- Inferential Statistics in Psychology
- Insanity Defense, The
- Intelligence
- Intelligence, Crystallized and Fluid
- Intercultural Psychology
- Intergroup Conflict
- International Classification of Diseases and Related Healt...
- International Psychology
- Interviewing in Forensic Settings
- Intimate Partner Violence, Psychological Perspectives on
- Introversion–Extraversion
- Item Response Theory
- Law, Psychology and
- Lazarus, Richard
- Learned Helplessness
- Learning Theory
- Learning versus Performance
- LGBTQ+ Romantic Relationships
- Lie Detection in a Forensic Context
- Life-Span Development
- Locus of Control
- Loneliness and Health
- Mathematical Psychology
- Meaning in Life
- Mechanisms and Processes of Peer Contagion
- Media Violence, Psychological Perspectives on
- Memories, Autobiographical
- Memories, Flashbulb
- Memories, Repressed and Recovered
- Memory, False
- Memory, Human
- Memory, Implicit versus Explicit
- Memory in Educational Settings
- Memory, Semantic
- Meta-Analysis
- Metacognition
- Metaphor, Psychological Perspectives on
- Microaggressions
- Military Psychology
- Mindfulness
- Mindfulness and Education
- Minnesota Multiphasic Personality Inventory (MMPI)
- Money, Psychology of
- Moral Conviction
- Moral Development
- Moral Psychology
- Moral Reasoning
- Nature versus Nurture Debate in Psychology
- Neuroscience of Associative Learning
- Nonergodicity in Psychology and Neuroscience
- Nonparametric Statistical Analysis in Psychology
- Observational (Non-Randomized) Studies
- Obsessive-Complusive Disorder (OCD)
- Occupational Health Psychology
- Olfaction, Human
- Operant Conditioning
- Optimism and Pessimism
- Organizational Justice
- Parenting Stress
- Parenting Styles
- Parents' Beliefs about Children
- Peace Psychology
- Perception, Person
- Performance Appraisal
- Personality and Health
- Personality Disorders
- Personality Psychology
- Person-Centered and Experiential Psychotherapies: From Car...
- Phenomenological Psychology
- Placebo Effects in Psychology
- Play Behavior
- Positive Psychological Capital (PsyCap)
- Positive Psychology
- Posttraumatic Stress Disorder (PTSD)
- Prejudice and Stereotyping
- Pretrial Publicity
- Prisoner's Dilemma
- Problem Solving and Decision Making
- Procrastination
- Prosocial Behavior
- Prosocial Spending and Well-Being
- Protocol Analysis
- Psycholinguistics
- Psychological Literacy
- Psychological Perspectives on Food and Eating
- Psychology, Political
- Psychoneuroimmunology
- Psychophysics, Visual
- Psychotherapy
- Psychotic Disorders
- Publication Bias in Psychology
- Reasoning, Counterfactual
- Rehabilitation Psychology
- Relationships
- Reliability–Contemporary Psychometric Conceptions
- Religion, Psychology and
- Replication Initiatives in Psychology
- Research Methods
- Risk Taking
- Role of the Expert Witness in Forensic Psychology, The
- Sample Size Planning for Statistical Power and Accurate Es...
- Schizophrenic Disorders
- School Psychology
- School Psychology, Counseling Services in
- Self, Gender and
- Self, Psychology of the
- Self-Construal
- Self-Control
- Self-Deception
- Self-Determination Theory
- Self-Efficacy
- Self-Esteem
- Self-Monitoring
- Self-Regulation in Educational Settings
- Self-Report Tests, Measures, and Inventories in Clinical P...
- Sensation Seeking
- Sex and Gender
- Sexual Minority Parenting
- Sexual Orientation
- Signal Detection Theory and its Applications
- Simpson's Paradox in Psychology
- Single People
- Single-Case Experimental Designs
- Skinner, B.F.
- Sleep and Dreaming
- Small Groups
- Social Class and Social Status
- Social Cognition
- Social Neuroscience
- Social Support
- Social Touch and Massage Therapy Research
- Somatoform Disorders
- Spatial Attention
- Sports Psychology
- Stanford Prison Experiment (SPE): Icon and Controversy
- Stereotype Threat
- Stereotypes
- Stress and Coping, Psychology of
- Student Success in College
- Subjective Wellbeing Homeostasis
- Taste, Psychological Perspectives on
- Teaching of Psychology
- Terror Management Theory
- Testing and Assessment
- The Concept of Validity in Psychological Assessment
- The Neuroscience of Emotion Regulation
- The Reasoned Action Approach and the Theories of Reasoned ...
- The Weapon Focus Effect in Eyewitness Memory
- Theory of Mind
- Therapy, Cognitive-Behavioral
- Thinking Skills in Educational Settings
- Time Perception
- Trait Perspective
- Trauma Psychology
- Twin Studies
- Type A Behavior Pattern (Coronary Prone Personality)
- Unconscious Processes
- Video Games and Violent Content
- Virtues and Character Strengths
- Women and Science, Technology, Engineering, and Math (STEM...
- Women, Psychology of
- Work Well-Being
- Workforce Training Evaluation
- Wundt, Wilhelm
- Privacy Policy
- Cookie Policy
- Legal Notice
- Accessibility
Powered by:
- [185.66.14.236]
- 185.66.14.236
Thank you for visiting nature.com. You are using a browser version with limited support for CSS. To obtain the best experience, we recommend you use a more up to date browser (or turn off compatibility mode in Internet Explorer). In the meantime, to ensure continued support, we are displaying the site without styles and JavaScript.
- View all journals
- Explore content
- About the journal
- Publish with us
- Sign up for alerts
- Published: 05 April 2024
Single-case experimental designs: the importance of randomization and replication
- René Tanious ORCID: orcid.org/0000-0002-5466-1002 1 ,
- Rumen Manolov ORCID: orcid.org/0000-0002-9387-1926 2 ,
- Patrick Onghena 3 &
- Johan W. S. Vlaeyen ORCID: orcid.org/0000-0003-0437-6665 1
Nature Reviews Methods Primers volume 4 , Article number: 27 ( 2024 ) Cite this article
358 Accesses
3 Citations
8 Altmetric
Metrics details
- Data acquisition
- Human behaviour
- Social sciences
Single-case experimental designs are rapidly growing in popularity. This popularity needs to be accompanied by transparent and well-justified methodological and statistical decisions. Appropriate experimental design including randomization, proper data handling and adequate reporting are needed to ensure reproducibility and internal validity. The degree of generalizability can be assessed through replication.
This is a preview of subscription content, access via your institution
Access options
Access Nature and 54 other Nature Portfolio journals
Get Nature+, our best-value online-access subscription
24,99 € / 30 days
cancel any time
Subscribe to this journal
Receive 1 digital issues and online access to articles
111,21 € per year
only 111,21 € per issue
Buy this article
- Purchase on SpringerLink
- Instant access to full article PDF
Prices may be subject to local taxes which are calculated during checkout
Kazdin, A. E. Single-case experimental designs: characteristics, changes, and challenges. J. Exp. Anal. Behav. 115 , 56–85 (2021).
Article Google Scholar
Shadish, W. & Sullivan, K. J. Characteristics of single-case designs used to assess intervention effects in 2008. Behav. Res. 43 , 971–980 (2011).
Tanious, R. & Onghena, P. A systematic review of applied single-case research published between 2016 and 2018: study designs, randomization, data aspects, and data analysis. Behav. Res. 53 , 1371–1384 (2021).
Ferron, J., Foster-Johnson, L. & Kromrey, J. D. The functioning of single-case randomization tests with and without random assignment. J. Exp. Educ. 71 , 267–288 (2003).
Michiels, B., Heyvaert, M., Meulders, A. & Onghena, P. Confidence intervals for single-case effect size measures based on randomization test inversion. Behav. Res. 49 , 363–381 (2017).
Aydin, O. Characteristics of missing data in single-case experimental designs: an investigation of published data. Behav. Modif. https://doi.org/10.1177/01454455231212265 (2023).
De, T. K., Michiels, B., Tanious, R. & Onghena, P. Handling missing data in randomization tests for single-case experiments: a simulation study. Behav. Res. 52 , 1355–1370 (2020).
Baek, E., Luo, W. & Lam, K. H. Meta-analysis of single-case experimental design using multilevel modeling. Behav. Modif. 47 , 1546–1573 (2023).
Michiels, B., Tanious, R., De, T. K. & Onghena, P. A randomization test wrapper for synthesizing single-case experiments using multilevel models: a Monte Carlo simulation study. Behav. Res. 52 , 654–666 (2020).
Tate, R. L. et al. The single-case reporting guideline in behavioural interventions (SCRIBE) 2016: explanation and elaboration. Arch. Sci. Psychol. 4 , 10–31 (2016).
Google Scholar
Download references
Acknowledgements
R.T. and J.W.S.V. disclose support for the research of this work from the Dutch Research Council and the Dutch Ministry of Education, Culture and Science (NWO gravitation grant number 024.004.016) within the research project ‘New Science of Mental Disorders’ ( www.nsmd.eu ). R.M. discloses support from the Generalitat de Catalunya’s Agència de Gestió d’Ajusts Universitaris i de Recerca (grant number 2021SGR00366).
Author information
Authors and affiliations.
Experimental Health Psychology, Faculty of Psychology and Neuroscience, Maastricht University, Maastricht, the Netherlands
René Tanious & Johan W. S. Vlaeyen
Department of Social Psychology and Quantitative Psychology, Faculty of Psychology, University of Barcelona, Barcelona, Spain
Rumen Manolov
Methodology of Educational Sciences Research Group, Faculty of Psychology and Educational Science, KU Leuven, Leuven, Belgium
Patrick Onghena
You can also search for this author in PubMed Google Scholar
Corresponding author
Correspondence to René Tanious .
Ethics declarations
Competing interests.
The authors declare no competing interests.
Rights and permissions
Reprints and permissions
About this article
Cite this article.
Tanious, R., Manolov, R., Onghena, P. et al. Single-case experimental designs: the importance of randomization and replication. Nat Rev Methods Primers 4 , 27 (2024). https://doi.org/10.1038/s43586-024-00312-8
Download citation
Published : 05 April 2024
DOI : https://doi.org/10.1038/s43586-024-00312-8
Share this article
Anyone you share the following link with will be able to read this content:
Sorry, a shareable link is not currently available for this article.
Provided by the Springer Nature SharedIt content-sharing initiative
Quick links
- Explore articles by subject
- Guide to authors
- Editorial policies
Sign up for the Nature Briefing newsletter — what matters in science, free to your inbox daily.


- CREd Library , Research Design and Method
Single-Subject Experimental Design: An Overview
Cred library, julie wambaugh, and ralf schlosser.
- December, 2014
DOI: 10.1044/cred-cred-ssd-r101-002
Single-subject experimental designs – also referred to as within-subject or single case experimental designs – are among the most prevalent designs used in CSD treatment research. These designs provide a framework for a quantitative, scientifically rigorous approach where each participant provides his or her own experimental control.
An Overview of Single-Subject Experimental Design
What is single-subject design.
Transcript of the video Q&A with Julie Wambaugh. The essence of single-subject design is using repeated measurements to really understand an individual’s variability, so that we can use our understanding of that variability to determine what the effects of our treatment are. For me, one of the first steps in developing a treatment is understanding what an individual does. So, if I were doing a group treatment study, I would not necessarily be able to see or to understand what was happening with each individual patient, so that I could make modifications to my treatment and understand all the details of what’s happening in terms of the effects of my treatment. For me it’s a natural first step in the progression of developing a treatment. Also with the disorders that we deal with, it’s very hard to get the number of participants that we would need for the gold standard randomized controlled trial. Using single-subject designs works around the possible limiting factor of not having enough subjects in a particular area of study. My mentor was Dr. Cynthia Thompson, who was trained by Leija McReynolds from the University of Kansas, which was where a lot of single-subject design in our field originated, and so I was fortunate to be on the cutting edge of this being implemented in our science back in the late ’70s early ’80s. We saw, I think, a nice revolution in terms of attention to these types of designs, giving credit to the type of data that could be obtained from these types of designs, and a flourishing of these designs really through the 1980s into the 1990s and into the 2000s. But I think — I’ve talked with other single-subject design investigators, and now we’re seeing maybe a little bit of a lapse of attention, and a lack of training again among our young folks. Maybe people assume that people understand the foundation, but they really don’t. And more problems are occurring with the science. I think we need to re-establish the foundations in our young scientists. And this project, I think, will be a big plus toward moving us in that direction.
What is the Role of Single-Subject Design?
Transcript of the video Q&A with Ralf Schlosser. So what has happened recently, is with the onset of evidence-based practice and the adoption of the common hierarchy of evidence in terms of designs. As you noted the randomized controlled trial and meta-analyses of randomized controlled trials are on top of common hierarchies. And that’s fine. But it doesn’t mean that single-subject cannot play a role. For example, single-subject design can be implemented prior to implementing a randomized controlled trial to get a better handle on the magnitude of the effects, the workings of the active ingredients, and all of that. It is very good to prepare that prior to developing a randomized controlled trial. After you have implemented the randomized controlled trial, and then you want to implement the intervention in a more naturalistic setting, it becomes very difficult to do that in a randomized form or at the group level. So again, single-subject design lends itself to more practice-oriented implementation. So I see it as a crucial methodology among several. What we can do to promote what single-subject design is good for is to speak up. It is important that it is being recognized for what it can do and what it cannot do.
Basic Features and Components of Single-Subject Experimental Designs
Defining Features Single-subject designs are defined by the following features:
- An individual “case” is the unit of intervention and unit of data analysis.
- The case provides its own control for purposes of comparison. For example, the case’s series of outcome variables are measured prior to the intervention and compared with measurements taken during (and after) the intervention.
- The outcome variable is measured repeatedly within and across different conditions or levels of the independent variable.
See Kratochwill, et al. (2010)
Structure and Phases of the Design Single-subject designs are typically described according to the arrangement of baseline and treatment phases.
The conditions in a single-subject experimental study are often assigned letters such as the A phase and the B phase, with A being the baseline, or no-treatment phase, and B the experimental, or treatment phase. (Other letters are sometimes used to designate other experimental phases.) Generally, the A phase serves as a time period in which the behavior or behaviors of interest are counted or scored prior to introducing treatment. In the B phase, the same behavior of the individual is counted over time under experimental conditions while treatment is administered. Decisions regarding the effect of treatment are then made by comparing an individual’s performance during the treatment, B phase, and the no-treatment. McReynolds and Thompson (1986)
Basic Components Important primary components of a single-subject study include the following:
- The participant is the unit of analysis, where a participant may be an individual or a unit such as a class or school.
- Participant and setting descriptions are provided with sufficient detail to allow another researcher to recruit similar participants in similar settings.
- Dependent variables are (a) operationally defined and (b) measured repeatedly.
- An independent variable is actively manipulated, with the fidelity of implementation documented.
- A baseline condition demonstrates a predictable pattern which can be compared with the intervention condition(s).
- Experimental control is achieved through introduction and withdrawal/reversal, staggered introduction, or iterative manipulation of the independent variable.
- Visual analysis is used to interpret the level, trend, and variability of the data within and across phases.
- External validity of results is accomplished through replication of the effects.
- Social validity is established by documenting that interventions are functionally related to change in socially important outcomes.
See Horner, et al. (2005)
Common Misconceptions
Single-Subject Experimental Designs versus Case Studies
Transcript of the video Q&A with Julie Wambaugh. One of the biggest mistakes, that is a huge problem, is misunderstanding that a case study is not a single-subject experimental design. There are controls that need to be implemented, and a case study does not equate to a single-subject experimental design. People misunderstand or they misinterpret the term “multiple baseline” to mean that because you are measuring multiple things, that that gives you the experimental control. You have to be demonstrating, instead, that you’ve measured multiple behaviors and that you’ve replicated your treatment effect across those multiple behaviors. So, one instance of one treatment being implemented with one behavior is not sufficient, even if you’ve measured other things. That’s a very common mistake that I see. There’s a design — an ABA design — that’s a very strong experimental design where you measure the behavior, you implement treatment, and you then to get experimental control need to see that treatment go back down to baseline, for you to have evidence of experimental control. It’s a hard behavior to implement in our field because we want our behaviors to stay up! We don’t want to see them return back to baseline. Oftentimes people will say they did an ABA. But really, in effect, all they did was an AB. They measured, they implemented treatment, and the behavior changed because the treatment was successful. That does not give you experimental control. They think they did an experimentally sound design, but because the behavior didn’t do what the design requires to get experimental control, they really don’t have experimental control with their design.
Single-subject studies should not be confused with case studies or other non-experimental designs.
In case study reports, procedures used in treatment of a particular client’s behavior are documented as carefully as possible, and the client’s progress toward habilitation or rehabilitation is reported. These investigations provide useful descriptions. . . .However, a demonstration of treatment effectiveness requires an experimental study. A better role for case studies is description and identification of potential variables to be evaluated in experimental studies. An excellent discussion of this issue can be found in the exchange of letters to the editor by Hoodin (1986) [Article] and Rubow and Swift (1986) [Article]. McReynolds and Thompson (1986)
Other Single-Subject Myths
Transcript of the video Q&A with Ralf Schlosser. Myth 1: Single-subject experiments only have one participant. Obviously, it requires only one subject, one participant. But that’s a misnomer to think that single-subject is just about one participant. You can have as many as twenty or thirty. Myth 2: Single-subject experiments only require one pre-test/post-test. I think a lot of students in the clinic are used to the measurement of one pre-test and one post-test because of the way the goals are written, and maybe there’s not enough time to collect continuous data.But single-case experimental designs require ongoing data collection. There’s this misperception that one baseline data point is enough. But for single-case experimental design you want to see at least three data points, because it allows you to see a trend in the data. So there’s a myth about the number of data points needed. The more data points we have, the better. Myth 3: Single-subject experiments are easy to do. Single-subject design has its own tradition of methodology. It seems very easy to do when you read up on one design. But there are lots of things to consider, and lots of things can go wrong.It requires quite a bit of training. It takes at least one three-credit course that you take over the whole semester.
Further Reading: Components of Single-Subject Designs
Kratochwill, T. R., Hitchcock, J., Horner, R. H., Levin, J. R., Odom, S. L., Rindskopf, D. M. & Shadish, W. R. (2010). Single-case designs technical documentation. From the What Works Clearinghouse. http://ies.ed.gov/ncee/wwc/documentsum.aspx?sid=229
Further Reading: Single-Subject Design Textbooks
Kazdin, A. E. (2011). Single-case research designs: Methods for clinical and applied settings. Oxford University Press.
McReynolds, L. V. & Kearns, K. (1983). Single-subject experimental designs in communicative disorders. Baltimore: University Park Press.
Further Reading: Foundational Articles
Julie Wambaugh University of Utah
Ralf Schlosser Northeastern University
The content of this page is based on selected clips from video interviews conducted at the ASHA National Office.
Additional digested resources and references for further reading were selected and implemented by CREd Library staff.
Copyright © 2015 American Speech-Language-Hearing Association
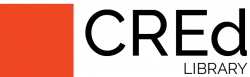
Clinical Research Education
More from the cred library, innovative treatments for persons with dementia, implementation science resources for crisp, when the ears interact with the brain, follow asha journals on twitter.

© 1997-2024 American Speech-Language-Hearing Association Privacy Notice Terms of Use
Breadcrumbs Section. Click here to navigate to respective pages.

Single-Case Experimental Designs for Clinical Research and Neurorehabilitation Settings
DOI link for Single-Case Experimental Designs for Clinical Research and Neurorehabilitation Settings
Get Citation
This book is a practical resource designed for clinicians, researchers, and advanced students who wish to learn about single-case research designs. It covers the theoretical and methodological underpinnings of single-case designs, as well as their practical application in the clinical and research neurorehabilitation setting. The book briefly traces the history of single-case experimental designs (SCEDs); outlines important considerations in understanding and planning a scientifically rigorous single-case study, including internal and external validity; describes prototypical single-case designs (withdrawal-reversal designs and the medical N-of-1 trial, multiple-baseline designs, alternating-treatments designs, and changing-criterion designs) and required features to meet evidence standards, threats to internal validity, and strategies to address them; addresses data evaluation, covering visual analysis of graphed data, statistical techniques, and clinical significance; and provides a practical ten-step procedure for implementing single-case methods. Each chapter includes detailed illustrative examples from the neurorehabilitation literature.
Novel features include:
- A focus on the neurorehabilitation setting, which is particularly suitable for single-case designs because of the complex and often unique presentation of many patients/clients.
- A practical approach to the planning, implementation, data analysis, and reporting of single-case designs.
- An appendix providing a detailed summary of many recently published SCEDs in representative domains in the neurorehabilitation field, covering basic and instrumental activities of daily living, challenging behaviours, disorders of communication and cognition, mood and emotional functions, and motor-sensory disabilities.
It is valuable reading for clinicians and researchers in several disciplines working in rehabilitation, including clinical and neuropsychology, education, language and speech pathology, occupational therapy, and physical therapy. It is also an essential resource for advanced students in these fields who need a textbook for specialised courses on research methodology and use of single-case design in applied clinical and research settings.
TABLE OF CONTENTS
Chapter 1 | 22 pages, introduction to single-case experimental designs, chapter 2 | 28 pages, maximising validity in single-case designs, chapter 3 | 20 pages, the dependent variable, chapter 4 | 19 pages, the independent variable, chapter 5 | 18 pages, withdrawal/reversal designs, chapter 6 | 23 pages, multiple-baseline designs, chapter 7 | 13 pages, alternating-treatments designs, chapter 8 | 10 pages, changing-criterion designs, chapter 9 | 29 pages, data evaluation, chapter 10 | 26 pages, practical application of single-case methods in the neurorehabilitation setting.
- Privacy Policy
- Terms & Conditions
- Cookie Policy
- Taylor & Francis Online
- Taylor & Francis Group
- Students/Researchers
- Librarians/Institutions
Connect with us
Registered in England & Wales No. 3099067 5 Howick Place | London | SW1P 1WG © 2024 Informa UK Limited

An official website of the United States government
The .gov means it’s official. Federal government websites often end in .gov or .mil. Before sharing sensitive information, make sure you’re on a federal government site.
The site is secure. The https:// ensures that you are connecting to the official website and that any information you provide is encrypted and transmitted securely.
- Publications
- Account settings
The PMC website is updating on October 15, 2024. Learn More or Try it out now .
- Advanced Search
- Journal List
- Front Res Metr Anal
- PMC10679716

Single-case design meta-analyses in education and psychology: a systematic review of methodology
Mariola moeyaert.
1 Department of Educational and Counseling Psychology, University at Albany-State University of New York, Albany, NY, United States
Marzieh Dehghan-Chaleshtori
2 Center of Tsinghua Think Tanks, Tsinghua University, Beijing, China
Panpan Yang
3 Center for Research on Child Wellbeing, Princeton University, Wallace Hall, Princeton, NJ, United States
Associated Data
The original contributions presented in the study are included in the article/ Supplementary material , further inquiries can be directed to the corresponding author.
Meta-analysis is of increasing importance as this quantitative synthesis technique has the potential to summarize a tremendous amount of research evidence, which can help making evidence-based decisions in policy, practice, and theory. This paper examines the single-case meta-analyses within the Education and Psychology fields. The amount of methodological studies related to the meta-analysis of Single-Case Experimental Designs (SCEDs) is increasing rapidly, especially in these fields. This underscores the necessity of a succinct summary to help methodologists identify areas for further development in Education and Psychology research. It also aids applied researchers and research synthesists in discerning when to use meta-analytic techniques for SCED studies based on criteria such as bias, mean squared error, 95% confidence intervals, Type I error rates, and statistical power. Based on the summary of empirical evidence from 18 reports identified through a systematic search procedure, information related to meta-analytic techniques, data generation and analysis models, design conditions, statistical properties, conditions under which the meta-analytic technique is appropriate, and the study purpose(s) were extracted. The results indicate that three-level hierarchical linear modeling is the most empirically validated SCED meta-analytic technique, and parameter bias is the most prominent statistical property investigated. A large number of primary studies (more than 30) and at least 20 measurement occasions per participant are recommended for usage of SCED meta-analysis in Education and Psychology fields.
Introduction
Single-Case Experimental Designs (SCEDs) are experimental designs used to repeatedly measure one or multiple participants across at least two conditions: a condition without intervention (i.e., baseline condition) and a condition with intervention (i.e., intervention or treatment condition). Intervention effectiveness can be evaluated by comparing outcome data obtained from each participant's baseline condition with subsequent intervention condition. SCEDs are strong experimental designs because the individual participant provides their own control condition for comparison purposes. The main goal of comparing outcome data under these conditions is to determine whether there is a causal relationship between the introduction of the intervention and changes in outcome data (Onghena, 2005 ). This functional relationship is participant-specific and cannot be generalized beyond the participant (Van den Noortgate and Onghena, 2003a , b ). To increase the external validity of intervention effectiveness, SCED studies traditionally include multiple participants (e.g., Caron and Dozier, 2019 ; Petrocchi et al., 2021 ). Indeed, Shadish and Sullivan ( 2011 ) found an average number of study participants of 3.64, indicating multiple participants per SCED study. A more recent systematic review by Jamshidi et al. ( 2020 ) confirmed this finding and indicated an average of four participants per SCED study. Thus, outcome data obtained through SCED studies are often ordered according to a two-level hierarchical structure: repeated outcome data (i.e., level 1) nested within participants (i.e., level 2). To further generalize intervention effectiveness and contribute to evidence-based practices, summarizing evidence from one SCED study is insufficient. Therefore, several researchers have suggested combining evidence across SCED studies meeting strict inclusion criteria using meta-analytic techniques (i.e., Van den Noortgate and Onghena, 2008 ; Moeyaert et al., 2013a ; Shadish et al., 2013 and, e.g., Asaro-Saddler et al., 2021 ). This results in outcome data structured according to three levels: repeated outcome data (i.e., level 1) are nested within participants (i.e., level 2), and participants, in turn, are nested within studies (i.e., level 3).
Meta-analytic techniques for SCEDs in education and psychology fields
Two meta-analytic techniques for synthesizing SCED studies were previously empirically validated in published methodological research: multilevel modeling (MLM) and weighted average of summary statistics. Both are discussed in the following sections.
Multilevel modeling
The multilevel modeling (MLM) framework is promising for combining SCED data across participants and across studies as it takes the natural hierarchical data structure (and the dependencies derived from it) into account (Borenstein et al., 2009 ): outcome data are clustered within participants, and participants, in turn, are clustered within studies. The hierarchical linear model (HLM), as an extension of the piecewise regression equation (Center et al., 1985 ), has been empirically investigated through multiple Monte Carlo simulation studies and is the most popular SCED meta-analytic technique. For example, Van den Noortgate and Onghena ( 2003a , b ) proposed and validated two-level HLM to combine SCED data across participants. In 2007 and 2008, they extended the two-level HLM to three-level HLM to combine SCED data across studies (Van den Noortgate and Onghena, 2007 , 2008 ). Later, Moeyaert et al. ( 2013a , b ), Moeyaert et al. ( 2014 ) and Ugille et al. ( 2012 , 2014 ) empirically validated three-level HLM for SCED meta-analysis using large-scale Monte Carlo simulation methods.
The HLM meta-analytic model can be used to estimate the overall effect size(s) across participants and studies (i.e., fixed effects) and study-specific and participant-specific deviations from the overall average effect size (i.e., random effects, see Moeyaert, 2019 ). Ever since Van den Noortgate and Onghena ( 2003a , b ) proposed the usage of HLM for meta-analysis of SCED data, its statistical properties have been intensively investigated and validated through Monte Carlo simulation studies (e.g., Ugille et al., 2012 ; Moeyaert et al., 2013a , b , 2014 ; Declercq et al., 2020 ). It also has been applied in many SCED meta-analyses (e.g., Asaro-Saddler et al., 2021 ; Fingerhut and Moeyaert, 2022 ).
The most often seen models for the three-level HLM are the models with two parameters and with four parameters. In the two parameters HLM approach, a dummy variable indicating the phase (i.e., 0 for the baseline phase and 1 for the intervention phase) is the sole independent variable. This model provides an estimate of the baseline level and the level change between the baseline and intervention phases. The four parameters HLM approach, on the other hand, often includes the following four independent variables: phase, time (i.e., session number), and the interaction term between phase and time. This results in an estimate of the level at the start of the baseline, the baseline trend, and the level and trend change between the baseline and intervention phases. Additional parameters can be added to the four parameter HLM approach. For instance, two parameters can be added to model quadratic time trends: time squared and the interaction term between phase and time squared. The six parameters HLM approach has been studied infrequently.
Three-level HLM with two parameters
The two parameters three-level HLM approach is most frequently studied and is represented through Equations 1 to 3:
Equations (1)–(3) represent the first level (i.e., observation level), the second level (i.e., participant level), and the third level (i.e., study level) of the HLM framework, respectively. The SCED meta-analysis includes K number of studies and J number of participants, and each participant has been repeatedly measured over a total of I number of time points. y ijk is the outcome value at observation session i ( i = 1, 2, ..., I ) of participant j ( j = 1, 2, …, J ) in study k ( k = 1, 2, …, K ). Phase is a dummy coded variable with 0 representing the baseline phase and 1 representing the intervention phase. Equation (1) is a regression equation at the observation level that uses phase to model outcome y ijk . Thus, β 0 jk indicates the baseline level of participant j in study k , and β 1 jk represents the level change between the baseline and intervention phases of participant j in study k . Another symbol in Equation (1) is e ijk representing the within-participant residual standard deviation of participant j ( j = 1, 2, …, J ) at observation session i ( i = 1, 2, …, I ) in study k .
Equation (2) is the participant-level equation indicating that the baseline level of participant j (i.e., β 0 jk ) can be obtained by the average baseline level (i.e., θ 00 k ) across all participants from study k and the deviation of participant j from this study average (i.e., u 0 jk ). Similarly, the level change of participant j (i.e., β ijk ) equals the sum of the average level change (i.e., θ 10 k ) across J participants from study k and the deviation of participant j (i.e., u 1 jk ) from this study average . The deviations at level 2 are assumed to be normally distributed with a variance of σ u 0 2 (i.e., between-participant variance in baseline level) and σ u 1 2 (i.e., between-participant variance in intervention effect).
In Equation (3), γ 000 represents the average baseline level across K studies, and v 00 k indicates the between-study deviation of the baseline level. Likewise, γ 100 represents the average level change across K studies, and v 10 k indicates the between-study deviation of the level change. The deviations at level 3 are assumed to be normally distributed with a variance of σ v 0 2 (i.e., between-study variance in baseline level) and σ v 1 2 (i.e., between-study variance in intervention effect).
Three-level HLM model with four parameters
The two parameters HLM approach can be expanded to four parameters HLM by adding two independent variables (i.e., time and the interaction term between time and phase):
Level 1 (observation-level):
Equations (4)–(6) represent the first level (i.e., observation level), the second level (i.e., participant level), and the third level (i.e., study level) of the HLM, respectively. Compared to the two parameters HLM approach, the four parameters HLM approach includes two additional independent variables: Time0 and PhaseTimeC. Time0 is a time-related continuous variable that indicates the sequence in measuring the outcomes. Time0 equals zero for the first observation session. TimeC is also a time-related continuous variable, but it is centered around the beginning of the intervention phase. PhaseTimeC is an interaction term between TimeC and Phase. With these two additional parameters, this four parameters HLM approach can estimate the outcome level at the start of the baseline phase, baseline trend, outcome level change between the baseline and intervention phases at the start of the intervention, and trend change between baseline and intervention phases. In Equation (4), β 2 jk and β 3 jk represent the baseline trend and trend change between the baseline and intervention phase for participant j from study k . θ 20 k and θ 30 k represent the average baseline trend and the trend change across J participants in study k . γ 200 and γ 300 represent the average baseline trend and the trend change across K studies. u 2 jk and u 3 jk are the between-participant deviations of baseline trend and trend change of participant j in study k (assumed to be normally distributed with variance σ u 2 2 and σ u 3 2 , respectively). v 20 k and v 30 k are the between-study deviations of baseline trend and trend change in study k (assumed to be normally distributed with variance σ v 2 2 and σ v 3 2 , respectively).
Three-level HLM model with six parameters
The six parameters model includes the same parameters as in Equations (4)–(6) with two additional ones: Time squared (i.e., Time2) and the interaction term between Phase and Time squared (PhaseTime2), see Equation (7):
With these two additional parameters, this six parameters HLM approach can estimate the outcome level at the start of the baseline phase, baseline linear trend, baseline quadratic trend, outcome level change between baseline and intervention phases at the start of the intervention, linear trend change and quadratic trend change between baseline and intervention phases. In Equation (7), β 2 jk and β 5 jk represent the baseline quadratic trend and quadratic trend change between the baseline and intervention phase for participant j from study k . Similar to the previous approaches, the level 1 parameters vary at levels 2 and 3, and similar interpretations can be made.
The three aforementioned models (i.e., three-level HLM with two, four, and six parameters) are suitable for replicated AB designs and multiple baseline designs (MBDs). The models can be modified to meta-analyze data from ABAB withdrawal designs, alternating treatment designs (ATD), and other SCED designs (see Moeyaert et al., 2015 , 2022 ). In addition, moderators can be added to explain the variance when there is between-participant variance and/or between-study variance in parameter estimates. More information about moderators in SCEDs meta-analysis using HLM can be found in Moeyaert et al. ( 2021 , 2022 ).
Average of summary statistics
For each study participant, an effect size (e.g., the difference in means, non-overlap statistic, or regression-based statistic) can be calculated (i.e., summary statistic). Next, a simple average or weighted average of these participant-specific summary statistics can be estimated using the simple average or multilevel modeling techniques. Both results in an estimate of the overall average intervention effect. Because effect sizes from participants nested within one study are dependent, it is recommended to use cluster-robust variance estimation (RVE) in combination with calculating the simple average or multilevel modeling (see Chen and Pustejovsky, 2022 ). Because RVE is new to SCED meta-analysis, no methodological publications validating this approach were identified in the current systematic review.
Data analysis estimation methods in SCED meta-analyses in education and psychology fields
When a model is selected, there are many ways to identify the best-fit statistic value as the estimate of the parameters. The data analysis estimation methods include but are not limited to maximum likelihood estimation (MLE), least square estimation (LSE), and Bayesian analysis. MLE is a likelihood-based estimation approach that is frequently used for parameter estimation (Rossi, 2018 ). MLE finds the value with the highest likelihood based on the data under the assumed probability distribution (Pan and Fang, 2002 ; Myung, 2003 ). Restrict maximum likelihood estimation (RMLE) is a variation of MLE that uses a likelihood function to calculate the best-fit estimate instead of identifying the highest likelihood estimate based on the data. LSE is also widely used to estimate parameters. It identifies the best-fit statistic value by minimizing the squared discrepancies between the observed and expected data (Rosenbaum et al., 2005 ; Everitt and Howell, 2021 ). There are various kinds of LSE, such as ordinary least squares (OLS) and weighted least squares (WLS). Both OLS and WLS are a type of generalized least squares (GLS). Another estimation method is Bayesian estimation. When using Bayesian estimation techniques, the researcher begins with the specification of one or more parameters, such as regression coefficients and variances or standard deviations or precisions (i.e., the inverse of variances) known as the prior distribution. This preliminary information is combined with the information in the data to create the posterior distribution. The posterior distribution captures the researcher's knowledge of the parameters at the end of the study (see Rindskopf, 2014 , for a detailed demonstration of using Bayesian analysis for single-case designs).
Current study
Previously, a few systematic reviews of SCED meta-analyses were conducted to summarize characteristics of content-related SCED meta-analyses (e.g., Moeyaert et al., 2021 ; Jamshidi et al., 2022 ). However, none of them focused on the methodology, and they excluded methodological papers in their reviews. Methodological work related to SCED meta-analytic techniques is largely overlooked; therefore, this study is timely and targets a broad audience. Applied SCED meta-analysts can learn about the available techniques that have been empirically investigated and the conditions under which the technique(s) is(are) suitable (given its desirable statistical properties). Methodologists can learn about the need for potential further methodological research and the commonly encountered realistic design conditions and parameter values included in previous methodological work. More specifically, this paper aims to address the following research questions:
- What meta-analytic techniques, suitable to quantitatively synthesize SCED research, have been empirically validated through the Monte Carlo simulation?
- What SCED meta-analytic data generation models were used in the Monte Carlo simulation studies?
- What are the design conditions and parameter values included in the Monte Carlo simulation studies?
- What statistical properties are investigated in the Monte Carlo simulation studies?
- What are the conclusions regarding the statistical properties, per meta-analytic technique? In other words, under which realistic SCED meta-analytic conditions are investigated meta-analytic techniques recommended?
- What is the purpose of each meta-analytic study?
This systematic review was performed following the Preferred Reporting Items for Systematic Reviews and Meta-Analyses (PRISMA; Page et al., 2021 ) guideline. The PRISMA checklist is included as an Appendix .
Eligibility criteria
The two specific inclusion criteria were: (1) methodological research, including Monte Carlo simulation or other validation techniques, and (2) the design is a SCED meta-analysis. The exclusion criteria were (1) designs other than SCED meta-analysis, (2) non-methodological research, (3) fields outside Education and Psychology, (4) non-human participants, and (5) non-English resources.
Information sources
An extensive systematic literature search was conducted in December 2021 using the following databases: PsycINFO, Education Resources Information Center (ERIC), Web of Science (WOS), PubMed, DANS EASY (Archive hosting thousands of datasets), and ProQuest Dissertation and Thesis. PsycINFO, ERIC, and WOS were identified to have the most considerable scope within the field of psychology and education sciences. PubMed, DANS EASY, and ProQuest Dissertation and Thesis were used to search for gray literature.
Search strategy
Two independent researchers conducted the systematic search. These two researchers were doctoral students trained in the field of single-case design and meta-analysis (and had taken graduate-level courses on both subjects and collaborated on a few other systematic review and meta-analysis projects).
The independent researchers used the search terms presented in Table 1 for each electronic database. These were modified to fit the search format for each database (see Appendix A for the syntax used in each database). Finally, the search terms were combined using the “OR” and “AND” Boolean operators. An interobserver agreement of 100% was obtained.
Search strings electronic databases.
1. “single case” |
2. “single subject” |
3. “N of 1” |
4. “small N” |
5. “multiple baseline” |
6. “alternating treatment” |
7. “reversal design” |
8. “withdrawal design” |
9. (1 OR 2 OR 3 OR 4 OR 5 OR 6 OR 7 OR 8) |
10. “meta-analysis” |
11. “synthesis” |
12. “review” |
13. (10 OR 11 OR 12) |
14. “simulation” |
15. “Monte Carlo” |
16. “monte carlo” |
17. (14 OR 15 OR 16) |
18. (9 AND 13 AND 17) |
Next, the title and abstract of the retained reports were downloaded, exported, and imported into Rayyan. Rayyan ( http://rayyan.qcri.org , Quzzani et al., 2016 ) is a free Application that helps screen the studies and was used to de-duplicate and select the eligible reports.
After the first screening, the eligible reports were classified per journal. This was used to identify the top three journals publishing methodological research related to SCED meta-analyses: Behavior Research Methods, Journal of Experimental Education , and Multivariate Behavioral Research . The three journal websites were systematically searched using the same search strings (see Table 1 ; Appendix A ) as the systematic search of electronic databases. Once the final pool of eligible studies was set, the two independent researchers completed the backward search. Finally, the references of all reports included in the final pool were searched, and there was 100% interobserver agreement that no extra studies met the eligibility criteria.
Data collection
Every study was perused, and information about the meta-analytic technique, data generation model, design conditions, parameter values, statistical properties, conclusions and recommendations per meta-analytic technique, and research purpose were extracted.
The meta-analytic techniques in SCED were coded into four categories: (1) three-level hierarchical linear modeling (HLM, multilevel modeling, or linear mixed effects modeling), (2) generalized linear mixed effects modeling (GLMM), (3) meta-analysis of summary data (including the simple average of effect sizes, the median of effect sizes, and weighted average of effect sizes), and (4) other(s).
The data generation model was coded into three categories: (1) three-level HLM approach with two parameters (i.e., baseline level and level change between baseline and intervention phases), (2) three-Level HLM approach with four parameters (i.e., baseline level, baseline trend, level and trend change between baseline and intervention phases), and (3) other(s).
The data-generation design included information about the design of the primary SCED studies (e.g., MBD, AB), the number of primary SCED studies, the number of participants in each study, and the number of observation sessions.
The data analysis estimation method was coded into four categories: (1) MLE or REML, (2) OLS, (3) GLS, and (4) other(s).
The parameter values referred to the hypothesized values that researchers assigned for simulating SCED meta-analytic data. The type and number of parameters were based on the data-generation models.
Information about studied statistical properties was also retrieved. The statistical properties investigated can be defined as follow: the difference between the expected effect estimate and the true population effect (absolute bias); absolute bias divided by the population parameter value (relative bias; Moeyaert et al., 2014 ); the relative bias for the standard error parameter (relative SE bias); the proportion of 95% confidence intervals that contain the estimated parameter (95% confidence interval coverage; Owens, 2011 ); a measure of the average squared errors (mean squared error or MSE; Petit-Bois, 2014 ); the MSE divided by the squared nominal parameter value (relative MSE; Declercq et al., 2019 ); square root of mean of the squared difference of the estimated parameter from the true parameter (root mean square error or RMSE; Baek et al., 2020 ); the standard deviation of the sampling distribution of the effect estimator (standard error or SE; Moeyaert et al., 2014 ); the probability of rejecting the null hypothesis when the null hypothesis is true (Type I error); the probability of rejecting the null hypothesis when in fact a certain alternative parameter value is true (power; Cohen, 2013 ).
The research purpose was coded into six categories considering examination of the performance/appropriateness of a model or comparing the performances/appropriateness of models in terms of (1) statistical properties; (2) handling different SCED designs; (3) handling data complexity; (4) accounting for different effect size metrics; (5) handling model misspecification; and (6) others.
These items can be found in the codebook (see Appendix B ) and were piloted by the same two independent researchers involved in the data selection. Six studies were randomly selected to be coded by two independent reviewers to calculate the IOA. The calculated IOA was 87%. After discussing and resolving the discrepancies, one coder proceeded with the coding of the remaining studies. Data extracted from included studies and used for the analysis can be accessed by contacting the corresponding author.
Study selection
Through Rayyan, the two independent researchers reviewed the titles and abstracts and applied the inclusion and exclusion criteria. First, the researchers added labels reporting the reason for excluding reports. Then, based on the exclusion criteria, the following labels were predefined to select from (1) designs other than SCED meta-analysis, (2) non-methodological research, (3) studies reported in languages other than English, (4) fields outside education and psychology, and (5) non-human participants. The first screening (reviewing the titles and abstracts) was over-inclusive, as recommended by Higgins et al. ( 2021 ). For instance, titles and abstracts that did not contain enough information to determine eligibility were included. The same procedure was followed for the full-text screening.
The initial search yielded 204 results [PsychINFO ( n = 48), ERIC ( n = 16), WOS ( n = 78), DANS EASY Archive ( n = 2), PubMed ( n = 42), and ProQuest Dissertation and Thesis ( n = 18)]. For a more detailed overview, see the PRISMA flowchart displayed in Figure 1 . All study references per database were imported into Rayyan (Quzzani et al., 2016 ). Using Rayyan, sixty-three duplicates were found, resulting in 141 remaining reports for screening. When the abstract screening was completed, 40 reports remained. The titles and abstracts screening was done by two reviewers with an IOA of 87%. Discrepancies were discussed and resolved between the two reviewers. The discrepancies were due to demonstration/illustration studies that were not looking into empirical validation of a meta-analytic model suitable for quantitative synthesis of SCED studies.

Flowchart of study selection.
After the title and abstract screening was completed and discrepancies were solved, the top three journals (i.e., Behavior Research Methods, Journal of Experimental Education , and Multivariate Behavioral Research ) were systematically searched using the same search terms. This search yielded 145 articles [ Multivariate Behavioral Journal ( n = 70), Behavior Research Method ( n = 49), and Journal of Experimental Research ( n = 26)]. After importing the articles in Rayyan and checking for duplicates, 126 new articles were identified. Out of the 126 articles, only nine met the inclusion criteria after title and abstract screening. The title and abstract screening at this stage was also conducted by the same two independent researchers, who reached 79.2% of IOA. The discrepancies were discussed and resolved afterward. Discrepancies were due to insufficient information in the abstract to decide if the resource met the inclusion criteria.
The full-text screening was accomplished by the same two independent researchers, and an IOA of 79.2% was obtained. Discrepancies were discussed and resolved. Discrepancies were because of demonstration/illustration studies that are not looking into empirical validation of a meta-analytic model suitable for quantitative synthesis of SCED studies.
After the full-text screening, the final pool of eligible reports contained 18 entries (both journal articles and dissertations). Later, the two independent reviewers went over the references of all 18 resources to do a citation search and found no additional articles meeting the inclusion criteria.
The raw data retrieved from the SCED meta-analysis methodological studies were saved and analyzed in Excel (Microsoft Corporation, 2018 ). Descriptive analyses were used to summarize the meta-analytic characteristics (i.e., meta-analytic technique, data generation model, data generation design, and data estimation) of the included 18 SCED meta-analysis methodology studies. Then, the design conditions and statistical properties were summarized using frequency tables.
Using data retrieved from these 18 reports, the next section contains a summary of the meta-analytic techniques, data generation models, data analysis models, and data generation designs. Next, the results related to design conditions, statistical properties, recommended conditions under which each meta-analytic technique is appropriate, and the research purpose of each study are synthesized.
Meta-analytic techniques
An overview of meta-analytic characteristics, including meta-analytic technique and data generation model used in SCED meta-analysis methodological studies in Education and Psychology fields using HLM, is presented in Table 2 of Appendix C . HLM (or multilevel analysis, linear mixed-effects model) was the most frequently investigated SCED meta-analytic technique (i.e., n = 17, 94.4%). The other SCED meta-analytic technique, empirically validated, was the average of effect sizes, which was only used in one study (i.e., Idleman, 1993 ). Idleman ( 1993 ) compared three quantification metrics (i.e., PND, Glass' effect sizes, and Hedge's unbiased estimators) and used the average of the effect size as the method to aggregate the treatment effect.
Data generation and data analysis models
The majority of the studies used one model to generate data, while two studies used multiple models to generate data (Idleman, 1993 ; Declercq et al., 2020 ). Among the 17 studies that used HLM as the meta-analysis technique, 14 (78%) used three-level HLM with four parameters, two (11%) used three-level HLM with two parameters (i.e., Owens, 2011 ; Moeyaert et al., 2016 ), and one used three-level HLM with two, four, and six parameters (i.e., Declercq et al., 2020 ) to generate data. The only study that used a meta-analysis of summary statistics (i.e., Idleman, 1993 ) generated normal or chi-squared distributed data with and without autocorrelation.
Most of the studies used the same analysis model as the data generation model to estimate the parameters of interest (specifically, about half of included studies: n = 8; 44.4%). Two studies (Declercq et al., 2020 ; Joo et al., 2021 ) used a slightly alternative analysis model, which allowed us to investigate the impact of model misspecification. Five studies (28%) used both the same analysis model and a slightly alternative analysis model. These studies are Moeyaert et al. ( 2013b ), Petit-Bois ( 2014 ), Moeyaert et al. ( 2016 ), Petit-Bois et al. ( 2016 ), and Jamshidi et al. ( 2020 ). Three studies used completely different alternative analysis models. For example, Baek et al. ( 2020 ) did not specify the data generation model but used eight different analysis models (i.e., four three-level models and four four-level models). Manolov et al. ( 2014 ) used the three-level HLM with four parameters to simulate data. They used the weighted average of non-overlap statistics (e.g., NAP) and the standardized mean difference to estimate the overall average intervention effect. Similarly, Idleman ( 1993 ) simulated normal or chi-squared distributed data and analyzed the data using the average PND, average Glass' delta, and average Hedge's g.
Data generation designs
Although multilevel models can be used to meta-analyze SCED data from a variety of designs, such as alternating-treatment designs (ATD), replicated ABAB designs, and changing criterion designs (Shadish et al., 2013 ; Jamshidi et al., 2022 ), the majority of reports generated data using a multiple baseline design (MBD; 78%; n = 14). This is not surprising as MBDs are the most frequently used designs in practice (Jamshidi et al., 2022 ) and are recognized as having higher internal and external validity than other SCED types (Baek et al., 2020 ; Jamshidi et al., 2022 ). Three studies (i.e., Idleman, 1993 ; Owens, 2011 ; Tsai, 2011 ) simulated basic AB design data (17%), and one study (i.e., Declercq et al., 2020 ) used replicated AB design data (5%).
Data analysis estimation methods
The most popular estimation procedure was REML. Among the 17 studies that used HLM as their meta-analytic technique, 15 used MLE/REML. Two studies (i.e., Moeyaert et al., 2013b ; Manolov et al., 2014 ) did not specify the estimation procedures they used. The remaining study (i.e., Idleman, 1993 ) used the sample average of the effect sizes as the aggregate treatment effect; the data analysis estimation methods were varied based on how the effect sizes (i.e., PND, Glass' delta, and Hedges' g) were calculated. Thus, the data-analysis estimation method of this study was coded as others.
Design conditions
SCED meta-analytic data in Education and Psychology fields were generated under a variety of design conditions. This allows for investigating the appropriateness of the analysis model under various realistic conditions, allowing for more generalized conclusions. The first factor influencing the design condition is the number of units. The second factor pertains to the number of parameters (and values assigned to these parameters) used in the data generation model.
Number of units
The first factor influencing the design condition was the number of units at the observation level (repeated observations within participants), participant level (number of participants in each SCED study), and study level (number of studies in the meta-analysis). An overview of the number of units at the three levels is presented in this section, and more details can be found in Appendix C . The most often used value for the number of studies was 10 (88.9%; n = 16), followed by 30 (72.2%; n = 13). Regarding the number of participants, 72.2% (n = 13) included four participants, and 50% ( n = 9) of the studies included five participants. When setting the number of observation sessions, 20 (58.3; n = 15) was the most commonly used value, followed by 10 (61.1%; n = 11) and 40 (44.4%; n = 8) measurement occasions. The most commonly used design condition related to the number of units was 10 studies with four participants within each primary study and 10 measurement occasions within each participant. Over half of the reports (55.6%; n =10) used this design condition. The second most commonly used conditions contained 10 studies, with seven participants for each primary study and 10 measurement occasions within each participant (i.e., 38.8%; n = 7).
Parameters in data generation model
The second factor influencing the design condition was the number of parameters and the values assigned to these parameters in the data generation model. The parameter values for studies using three-level HLM with four parameters ( n = 14) are discussed first, followed by the two studies that used three-level HLM with two parameters. Lastly, the parameter values of the study by Idleman ( 1993 ) and Declercq et al. ( 2020 ), using other generation models, are presented.
Three-level HLM with four parameters
As discussed previously, 14 studies used HLM with four parameters as their data generation model. These studies included four fixed-effect parameters: the baseline level, baseline trend, the level and trend change between baseline, and intervention effects (i.e., γ 000 , γ 100 , γ 200 and γ 300 , respectively; see Equations 4–6). The majority set the parameter value of the baseline level to zero ( n = 9) or did not mention it ( n = 3). Baek et al. ( 2020 ) set the baseline level at 70, and Manolov et al. ( 2014 ) set the baseline level at 7 or 40.
The average level change between baseline and intervention phases (i.e., γ 200 ), which represents the intervention effect, was most often set to a value of 2 ( n = 9), followed by 0 ( n = 6) and 1 ( n = 3). Eight studies included more than two conditions for the intervention effect. Five studies (i.e., Moeyaert et al., 2013a , b , 2014 , 2016 ; Declercq et al., 2020 ) set the intervention effect as 0 or 2; Jamshidi et al. ( 2020 ) set the intervention effect at 0.2 or 2; Joo et al. ( 2021 ) set the intervention effect at 0.5 or 1; and Tsai ( 2011 ) set the intervention effect to 0, 0.5, or 1.
For the time trend in baseline data (i.e., γ 100 ), nine out of the 14 studies set this parameter to zero. When researchers did include a trend change, time trends were set at 0.2, 04, 06, 1, 2, and 3. Two studies (i.e., Tsai, 2011 ; Ugille et al., 2012 ) had two or more values for the time trend. Expressly, Ugille et al. ( 2012 ) set the time trend in the baseline as either 0 or 2. Tsai ( 2011 ) set the time trend as 0.2, 0.4, or 0.6. Some studies (3 out of 14) did not mention the time trend in the baseline.
For the trend change parameter (γ 300 ), 0.2 and 0 ( n = 9 for each value) were the most often used values. Unlike the time trend in the baseline phase, quite a few studies ( n = 11) included two conditions for trend change. Five studies (i.e., Ugille et al., 2012 ; Moeyaert et al., 2013a , b , 2014 ; Petit-Bois, 2014 ) kept the trend change as either zero or 0.2. Idleman ( 1993 ), Tsai ( 2011 ), Declercq et al. ( 2020 ), and Joo et al. ( 2021 ), and kept the trend change as zero. They also set the trend change as 2, 0.3, 0.25, and 0.4, respectively. For the remaining two studies that included two conditions for trend change, Jamshidi et al. ( 2020 ) set the trend change as either 0.2 or 2. Manolov et al. ( 2014 ) kept the trend change as either 1 or 3. One study (i.e., Baek et al., 2020 ) did not mention the value for the trend change between the baseline and intervention phases.
Many studies did not specify their parameter values for random effects (i.e., within-participant, between-participant, and between-study variance). Among the studies that did specify the within-participant variance, the most often used within-participant variance parameter value was 1 ( n = 8). Researchers often kept the variance of the intervention effect the same as the variance of the baseline and the variance of the baseline time trend the same as the trend change. The most often used values of the between-participant variance of the baseline and intervention effect were 0.5 ( n = 9) and 2 ( n = 9), followed by 8 ( n = 4). For between-participant variance of the time trend and trend change, the most often used parameter values were 0.05 and 0.2 ( n = 8), followed by 0.08 ( n = 4). When setting the between-study variance of the baseline level, 0.5 ( n = 9), 2 ( n = 7), and 8 ( n = 4) were often used. The values 0.5 ( n = 9), 2 ( n = 8), and 8 ( n =4) were often used for the between-study variance of the intervention effect. The values 0.05 ( n =8), 0.2 ( n =7), and 0.08 ( n = 4) were often used for the between-study variance of the time trend in the baseline. Lastly, 0.05 ( n = 9), 0.2 ( n = 8), and 0.08 ( n = 4) were often used for the between-study trend change between the baseline and intervention phases.
The between-participant and between-study covariances were often not modeled ( n = 8). For studies that did specify the covariance, most studies set the covariance to 0 ( n = 6). Like covariance, most studies did not specify autocorrelation ( n = 6). For studies that did specify autocorrelation, most studies set the autocorrelation as 0 ( n = 7) or 0.2 ( n = 4).
Two studies (Owens, 2011 ; Moeyaert et al., 2016 ) used three-level HLM with two parameters to generate SCED meta-analytic data. These studies included two fixed-effect parameters: the average baseline level and the level change between baseline and intervention level (i.e., γ 000 , γ 100 ; see Equations 1–3). Moeyaert et al. ( 2016 ) kept the average baseline level at zero and set the level change between baseline and intervention at 0 or 2. Owens ( 2011 ) kept both fixed effects as 1.
In terms of random effects, Moeyaert et al. ( 2016 ) kept the within-participant variance at 1 and set the between-participant variances of baseline level and level change at 2 or 8. The between-study variance of baseline level and level change was also set at 2 or 8. Moeyaert et al. ( 2016 ) included two simulation studies. One kept the between-participant covariances and between-study covariances at zero. The other one set the between-participant and between-study covariances at −0.6 or −1.4 when the between-participant and between-study variances were 2, and −2.4 or −5.6 when the between-participant and between-study variances were 8. Moeyaert et al. ( 2016 ) focused on the covariance structure and did not include autocorrelation. Owens ( 2011 ) also kept the within-participant variance at 1. The between-participant variance of baseline level and level change were set at 2 or 0.2. The between-study variances of baseline level and level change were set at 0.05 or 0.5. Both between-participant and between-study covariances were kept at zero. Owens ( 2011 ) included autocorrelation and set the values at 0, 0.2, or 0.4.
Other meta-analytic models
Declercq et al. ( 2020 ) compared HLM with two parameters, four parameters, and six parameters. Idleman ( 1993 ) used simple average of effect sizes to synthesize effect sizes across studies and generated normal or chi-squared distributed data with and without autocorrelation. When the data followed a normal distribution, the baseline level was generated around 5, and the intervention effect was generated as 3.3, 2.2, or 1.5. When the data followed a chi-squared distribution, the baseline level was 5, while the intervention effect was 4.24, 6.23, or 9.33.
Statistical properties per meta-analytic technique
An overview of the statistical properties investigated by the 18 included SCED meta-analysis reports is displayed in Appendix C . Among the 17 studies that used HLM as their meta-analytic method, 15 studied confidence interval coverage (CI). CI was the most often studied statistical property, followed by bias ( n = 14) and relative bias ( n = 13). More than half of the included reports ( n = 11) also investigated standard error (SE). MSE was also investigated by a large proportion of meta-analytic studies (n = 11). Other statistical properties such as Power ( n = 6), Type I error ( n = 6), relative SE bias ( n = 5), and RMSE ( n = 4) were also investigated by some studies. One thing worth mentioning is that Manolov et al. ( 2014 ) did not study any statistical properties. The possible reason is that Manolov et al. ( 2014 ) focused on the weighting stages instead of evaluating the statistical properties of parameter estimates. The majority of studies investigated both Power and Type I error together. However, Moeyaert et al. ( 2013a ) reported Power but not Type I error. Declercq et al. ( 2020 ) studied Type I error rate but not Power.
Research purpose and recommendations
The most commonly reported purpose was to examine the performance or the appropriateness of the model(s) in terms of their statistical properties ( n = 5) and handling data complexity ( n = 5). Data complexities included but were not limited to heterogeneity, autocorrelation, and dependent effect sizes. Handling model misspecification was next, investigated by three studies. Two studies (i.e., Moeyaert et al., 2013b ; Declercq et al., 2020 ) examined the performance/appropriateness of the model(s) in handling different SCED designs, and two (i.e., Idleman, 1993 ; Ugille et al., 2012 ) looked at different effect size metrics. Idleman ( 1993 ) compared the appropriateness of PND, Glass' effect sizes, and Hedge's unbiased estimators for single-case research. Ugille et al. ( 2012 ) compared the performance of unstandardized and standardized regression coefficients as the effect size metrics in SCED meta-analysis. Although most included studies focused on the meta-analysis techniques and models, two studies focused on some other statistical techniques. Specifically, one study (i.e., Manolov et al., 2014 ) compared several weighting strategies, and another study (i.e., Ugille et al., 2014 ) explored four bias correction approaches for HLM meta-analysis of single-case studies with four parameters. An overview of the specific studies per research purpose is presented in Table 2 .
Research purpose per study.
(%) | ||
---|---|---|
Examining the performance/appropriateness of a model or comparing the performances/appropriateness of multiple models in terms of statistical properties. | 5 (28%) | Owens, ; Tsai, ; Moeyaert et al., ; Jamshidi et al., ; Joo et al., |
Examining the performance/appropriateness of a model or comparing the performances/appropriateness of multiple models in handling data complexity. | 5 (28%) | Moeyaert et al., ; Petit-Bois, ; Joo et al., ; Baek et al., ; Jamshidi et al., |
Examining the performance/appropriateness of a model or comparing the performances/appropriateness of multiple models in handling model misspecification. | 3 (17%) | Petit-Bois, ; Moeyaert et al., ; Petit-Bois et al., |
Examining the performance/appropriateness of a model or comparing the performances/appropriateness of multiple models in handling different SCED designs. | 2 (11%) | Moeyaert et al., ; Declercq et al., |
Examining the performance/appropriateness of a model or comparing the performances/appropriateness of multiple models with different effect size metrics. | 2 (11%) | Idleman, ; Ugille et al., |
Others | 2 (11%) | Manolov et al., ; Ugille et al., |
Some of the included studies made recommendations about the number of studies and observations to use. Moeyaert et al. ( 2013a , 2014 , 2016 ) recommended using a set of homogeneous primary studies (i.e., low between-study variance). The number of primary studies should be more than 30, and the number of observations should be at least 30 to reach a reasonable power. Jamshidi et al. ( 2020 ) encouraged including more than 40 studies in a meta-analysis, especially when the research interest lies in estimating the between-study variance. Tsai ( 2011 ) recommended to use 50 studies in a meta-analysis of SCEDs unless the expected intervention effect is large. Ugille et al. ( 2012 ) suggested including 20 or more observation sessions per participant when using standardized effect sizes in the multilevel meta-analysis. Lastly, Jamshidi et al. ( 2021 ) recommended using more than 10 observation sessions, especially when estimating the between-participant and between-study variance.
This study provides an overview of empirically validated meta-analytic techniques for synthesizing SCED studies in Education and Psychology fields. The findings of this systematic research can be helpful for methodologists in Education and Psychology fields to further develop the methodology of SCED meta-analytic techniques. Additionally, applied researchers and research synthesists in Education and Psychology fields can gain insight into the conditions under which the studied meta-analytic techniques for SCED studies are appropriate and recommended.
This systematic review results show that three-level HLM approach is the most commonly empirically validated meta-analytic technique. Using three-level HLM is reasonable as a hierarchical data structure characterizes SCED meta-analysis data: repeated observations (i.e., level 1) are nested within participants (i.e., level 2), and participants, in turn, are nested within studies (i.e., level 3). HLM can take this clustered data structure into account (Van den Noortgate and Onghena, 2003a , b , 2008 ), making it the most promising meta-analytic technique for SCED studies. Other benefits of using HLM are robustness in handling complex data hierarchies, and enhanced sensitivity to detect treatment effects (Price et al., 2008 ; McNeish et al., 2017 ). These benefits are often of paramount importance in SCED research.
For any research and statistical technique to be applied and recommended, sufficient methodological work is needed to investigate and empirically validate it. In addition, methodologists should ensure the technique has good statistical properties, such as providing unbiased estimation, and can perform well with complex data due to robustness against data complexity. Monte Carlo simulation study is a common way to examine the techniques' statistical properties. These simulation studies enable methodologists to systematically explore the technique's behavior under various conditions, including different sample sizes, effect sizes, and data distributions, helping researchers gain insights into the robustness and suitability of the used technique for practical application.
Concerning the research purpose, when the studies aimed to examine the performance of HLM, most simulation studies directly adapted three-level HLM, including four parameters as proposed by Van den Noortgate and Onghena ( 2003b , 2008 ) to generate SCED data across studies. A couple of simulation studies used the multilevel models proposed by Huitema and McKean ( 1991 , 2000 , 2007 ). The difference between these two models is how they estimate the trend change between the baseline and intervention phases. However, these two models have the same parameters and estimate the same number of fixed parameters. Both models included phase (i.e., a dummy variable with 0 = baseline and 1 = intervention phase), time, and the interaction between time and phase in their model. They estimate the baseline level, time trend, level change and trend change between baseline and intervention. Some researchers have extended these models by adding additional variables. For example, Moeyaert et al. ( 2014 ) proposed an extended model with an additional dummy-coded variable to control for external event effects. These extensions reflect the dynamic nature of SCED research and the necessity of considering external factors that may influence outcomes.
Most studies used HLM as the meta-analytic simulated SCED data with MBD. Using MBD is reasonable as the MBD is the most commonly used design in the SCED field (Hammond and Gast, 2010 ; Shadish and Sullivan, 2011 ). The remaining studies used either AB or repeated AB designs (i.e., Idleman, 1993 ; Coleman, 2006 ; Owens, 2011 ; Tsai, 2011 ; Declercq et al., 2020 ). One possible reason is that meta-analysis of MBD data across multiple participants and repeated AB design data can directly adapt the models proposed by Van den Noortgate and Onghena ( 2003b , 2008 ). Meta-analysis of other SCED design data, such as ATD, requires model modification, which is not yet developed. This raises important questions about the generalizability of HLM to various SCED designs and underscores the need for future research to address these gaps in modeling strategies.
Most studies included more than one design condition based on the number of units (i.e., the number of studies, participants, and measurements), the number of parameters, and the values assigned to the parameters. The most commonly used design condition includes 10 studies with four participants for each study and 10 observation sessions for each participant. Some extreme conditions, such as 80 studies and 50 participants per study, were also investigated by some methodological studies. These unrealistic design conditions were often simulated as an ideal situation to test the model. In terms of the parameters, the baseline level, time trend, and trend change between baseline and intervention were often set at zero, whereas the level change between baseline and intervention was often set at 1 or 2. Researchers often use the level change between the baseline and intervention phase as an indicator of the intervention effect, making it the parameter of interest in a SCED study. This is the possible reason why studies often set a level change for the simulated data while keeping the other parameter values constant. For random effects such as between-participant, between-study, and within-participant variance, the hypothesized values were selected to reflect the design conditions in practice. The variability in the design conditions used in SCED studies suggests that researchers should carefully consider and justify their choice of design conditions.
A large number of primary studies (more than 30) and observation sessions (more than 20) per participant are recommended. Some studies made recommendations about which conditions for a specific model work best. For example, Coleman ( 2006 ) recommended that researchers include 20 units in level one (e.g., 20 measurements) and 30 units in level two (e.g., 30 participants) for a meta-analysis to have enough power. Ugille et al. ( 2012 ) recommended including 20 or more observations when conducting a multilevel meta-analysis of SCEDs. Moeyaert et al. ( 2013a , 2014 ) also recommended that researchers include 30 or more studies and 20 or more observations within participants to have sufficient power to detect the intervention effect. Ugille et al. ( 2012 ) and Moeyaert et al. ( 2014 ) both mentioned the importance of using a homogeneous set of studies (i.e., small between-study variance). Jamshidi et al. ( 2020 , 2021 ) recommended having 40 or more studies to better estimate between-study variance. A critical assessment of these suggestions reveals their potential influence on the accuracy and reliability of between-study variance estimation. It is pertinent to consider whether these conditions can be met in diverse research context.
Most methodological studies not only considered the baseline level and the level change (between baseline and intervention phases) of the SCED data but also considered the baseline trend and trend change. However, some studies, such as Owens ( 2011 ) and Moeyaert et al. ( 2016 ), only considered the baseline level and the level change. One possible reason is that the three-level HLM with two parameters has fewer parameters, which is a good starting point to examine the appropriateness of the three-level HLM. For example, Moeyaert et al. ( 2016 ) focused on the misspecifications of the covariance structure. Thus, Moeyaert et al. ( 2016 ) used a three-level HLM with two parameters, which reduces the number of fixed parameters that need to be estimated to isolate and simplify the situation. A critical analysis of this choice should explore the potential implications for model fit and the validity of conclusions drawn from the simplified model.
In addition, researchers in the field recommend bootstrapping or Bayesian approaches to reduce parameter bias. For example, Rindskopf ( 2014 ) argues that despite the multilevel structure of single-case data, Bayesian methods are more useful than simple linear models due to several reasons: taking into account uncertainty in random effects when estimating fixed effects, being able to fit complex models that represent accurately the behavior being modeled; more accurately estimating groups of parameters using shrinkage methods; including prior information; and stating more straightforward interpretation. These approaches are particularly well-suited to address the unique challenges and complexities associated with single-case data. However, researchers in the field should carefully assess the applicability of these methods to their specific research contexts while recognizing that context-specific considerations may influence the choice of statistical approach.
Especially, Declercq et al. ( 2019 ) compared LMM (also known as HLM, multilevel analysis) with GLMM (Generalized linear mixed model) using REML as the estimation method in their simulation. They found that GLMM performed better regarding the goodness of fit and power. However, in estimating the average effect size across studies, the simple LMM works equally well with GLMM, as the parameter recovery is the same under both models. Furthermore, they assumed the outcomes followed a Poisson or a normal distribution. With transformation, Declercq et al. ( 2019 ) claimed that the average baseline response was 2, 4, 20, or 30, and the average treatment effect was 1, 1.5, 1.6, or 3.5. Shadish et al. ( 2013 ) expanded the HLM framework for synthesizing SCED effect sizes across studies by proposing a generalized multilevel analysis (i.e., generalized linear mixed model). The generalized linear mixed model (GLMM) is a combination of linear mixed modeling (LMM) and generalized linear modeling (GLM), taking both the hierarchical data structure and count data into account (Declercq et al., 2019 ). Furthermore, besides supporting a non-continuous data format, it supports dependent variables that are not normally distributed (Garson, 2013 ).
It is worth noting that Coleman ( 2006 ) and Declercq et al. ( 2019 ) studied two-level HLM but called their study a multilevel meta-analysis. These studies synthesized SCED data across participants within one study. Chen and Chen's ( 2014 ) study, which was excluded from the current systematic review, investigated combining n-of-1 trials and considered each subject an n -of-1 trial. Consequently, it is debatable if these studies can be classified as meta-analyses as they do not combine research evidence across studies, which is the original meaning of meta-analysis (Glass, 2012 ).

Limitations
Like any systematic review, this systematic review has its limitations. The search was done in December 2021, and it has been more than a year during which new studies may have been published. This means that the results of this review may reflect less up-to-date evidence.
Moreover, We acknowledge the limitation imposed by the relatively small number of studies included in this systematic review, comprising a total of 18 reports. This constraint naturally affects the generality and level of detail in the conclusions drawn from our analysis. While we have made every effort to extract meaningful insights from this limited pool of studies, we recognize that our findings must be considered within the context of this constraint. The limited number of studies does not allow for highly detailed claims. As such, our conclusions should be understood as reflective of the available evidence rather than comprehensive or all-encompassing. We have, however, strived to mitigate this limitation by employing rigorous systematic review methods, including well-defined inclusion and exclusion criteria. We view this review as a foundational exploration of the topic, intended to guide future research endeavors and offer initial insights into the subject matter.
It is important to note that we purposely narrowed down the scope to the field of Education and Psychology, guided by the principles of feasibility, interest, novelty, ethics, and relevance outlined in the FINER criteria (Cummings et al., 2013 ). This strategic focus enhances the applicability of our findings to these specific domains. Given the exponential increase in SCED meta-analyses, narrowing our focus to Education and Psychology makes our systematic review not only feasible but also highly relevant within these fields. Moreover, this systematic review underscores the need for a separate systematic review tailored to other fields such as medicine, recognizing the distinct challenges and requirements that each domain presents.
Data availability statement
Author contributions.
MM: conceptualization, interpretation results, write-up, and supervision. XX, MD-C, and PY: systematic search, analysis, and write-up. All authors contributed to the article and approved the submitted version.
* (indicates inclusion in study: N = 18).
Conflict of interest
The authors declare that the research was conducted in the absence of any commercial or financial relationships that could be construed as a potential conflict of interest.
Publisher's note
All claims expressed in this article are solely those of the authors and do not necessarily represent those of their affiliated organizations, or those of the publisher, the editors and the reviewers. Any product that may be evaluated in this article, or claim that may be made by its manufacturer, is not guaranteed or endorsed by the publisher.
Supplementary material
The Supplementary Material for this article can be found online at: https://www.frontiersin.org/articles/10.3389/frma.2023.1190362/full#supplementary-material
- Asaro-Saddler K., Moeyaert M., Xu X., Yerden X. (2021). Multilevel meta-analysis of the effectiveness of self-regulated strategy development in writing for children with ASD . Exceptionality 29 , 150–166. 10.1080/09362835.2020.1850457 [ CrossRef ] [ Google Scholar ]
- * . Baek E., Luo W., Henri M. (2020). Issues and solutions in meta-analysis of single-case design with multiple dependent variables using multilevel modeling . J. Exp. Educ . 10.1080/00220973.2020.1821342 [ CrossRef ] [ Google Scholar ]
- Borenstein M., Cooper H., Hedges L., Valentine J. (2009). Effect sizes for continuous data . Handb. Res. Synth. Meta Anal. 2 , 221–235. [ Google Scholar ]
- Caron E. B., Dozier M. (2019). Effects of fidelity-focused consultation on clinicians' implementation: an exploratory multiple baseline design . Administr. Policy Mental Health Serv. Res. 46 , 445–457. 10.1007/s10488-019-00924-3 [ PMC free article ] [ PubMed ] [ CrossRef ] [ Google Scholar ]
- Center B. A., Skiba R. J., Casey A. (1985). A methodology for the quantitative synthesis of intra-subject design research . J. Spec. Educ. 19 , 387–400. [ Google Scholar ]
- Chen M., Pustejovsky J. E. (2022). Multi-level meta-analysis of single-case experimental designs using robust variance estimation . Psychol. Methods . 10.1037/met0000510 [ PubMed ] [ CrossRef ] [ Google Scholar ]
- Chen X., Chen P. (2014). A comparison of four methods for the analysis of N-of-1 trials . PLoS ONE 9 , e87752. 10.1371/journal.pone.0087752 [ PMC free article ] [ PubMed ] [ CrossRef ] [ Google Scholar ]
- Cohen J. (2013). Statistical Power Analysis for the Behavioral Sciences . New York, NY: Accademic Press. [ Google Scholar ]
- Coleman J. L. (2006). A Simulation Study of the Piecewise Hierarchical Model Approach to Meta-analysis of Single-Subject Data, Vol . 68. ProQuest Dissertations Publishing. [ Google Scholar ]
- Cummings S. R., Browner W. S., Hulley S. B. (2013). Conceiving the research question and developing the study plan . Design. Clin. Res. 4 , 14–22. [ Google Scholar ]
- * . Declercq L., Jamshidi L., Fernandez Castilla B., Moeyaert M., Beretvas S. N., Ferron J. M., et al.. (2020). Multilevel meta-analysis of individual participant data of single-case experimental designs: One-stage versus two-stage methods . Multivar. Behav. Res . 10.1080/00273171.2020.1822148 [ PubMed ] [ CrossRef ] [ Google Scholar ]
- Declercq L., Jamshidi L., Fernández-Castilla B., Beretvas S. N., Moeyaert M., Ferron J. M., et al.. (2019). Analysis of single-case experimental count data using the linear mixed effects model: a simulation study . Behav. Res. Methods 51 , 2477–2497. 10.3758/s13428-018-1091-y [ PubMed ] [ CrossRef ] [ Google Scholar ]
- Everitt B. S., Howell D. C. (2021). Encyclopedia of Statistics in Behavioral Science–Vol. 2 . Chichester: Wiley. [ Google Scholar ]
- Fingerhut J., Moeyaert M. (2022). Training individuals to implement discrete trials with fidelity: a meta-analysis . Focus Autism Other Dev. Disabl. 37 , 239–250. 10.1177/10883576221081076 [ CrossRef ] [ Google Scholar ]
- Garson G. D. (2013). Fundamentals of hierarchical linear and multilevel modeling . Hierarch. Linear Model. Guide Appl. 3–25. 10.4135/9781483384450.n1 [ CrossRef ] [ Google Scholar ]
- Glass G. V. (2012). Meta-analysis: the quantitative synthesis of research findings . In: Handbook of Complementary Methods in Education Research , eds J. L. Green, J. L. Green, G. Camilli, P. B. Elmore, and P. B. Elmore (Lawrence Erlbaum Associates, Inc.), 427–438. [ Google Scholar ]
- Hammond D., Gast D. L. (2010). Descriptive analysis of single subject research designs: 1983–2007. Educ. Train. Autism Dev. Disabil. 45, 187–202 . Available online at: https://www.jstor.org/stable/23879806
- Higgins J. P. T., Thomas J., Chandler J., Cumpston M., Li T., Page M. J., et al.. (2021). Handbook for Systematic Reviews of Interventions, Version 6.2 . [ Google Scholar ]
- Huitema B. E., McKean J. W. (1991). Autocorrelation estimation and inference with small samples . Psychol. Bull. 110 , 291–304. 10.1037/0033-2909.110.2.291 [ CrossRef ] [ Google Scholar ]
- Huitema B. E., McKean J. W. (2000). Design specification issues in time-series intervention models . Educ. Psychol. Meas. 60 , 38–58. 10.1177/00131640021970358 [ CrossRef ] [ Google Scholar ]
- Huitema B. E., McKean J. W. (2007). Identifying autocorrelation generated by various error processes in interrupted time-series regression designs: a comparison of AR1 and portmanteau tests . Educ. Psychol. Meas. 67 , 447–459. 10.1177/0013164406294774 [ CrossRef ] [ Google Scholar ]
- * . Idleman L. S. (1993). The Comparison of Three Meta-Analytic Metrics for Single-Subject Research . ProQuest Dissertations Publishing, Georgia State University. [ Google Scholar ]
- * . Jamshidi L., Declercq L., Fernández-Castilla B., Ferron J. M., Moeyaert M., Beretvas S. N., et al.. (2020). Multilevel meta-analysis of multiple regression coefficients from single-case experimental studies . Behav. Res. Methods 52 , 2008–2019. 10.3758/s13428-020-01380-w [ PubMed ] [ CrossRef ] [ Google Scholar ]
- * . Jamshidi L., Declercq L., Fernández-Castilla B., Ferron J. M., Moeyaert M., Beretvas S. N., et al.. (2021). Bias adjustment in multilevel meta-analysis of standardized single-case experimental data . J. Exp. Educ. 89 , 344–361. 10.1080/00220973.2019.1658568 [ CrossRef ] [ Google Scholar ]
- Jamshidi L., Heyvaert M., Declercq L., Fernández-Castilla B., Ferron J. M., Moeyaert M., et al.. (2022). A systematic review of single-case experimental design meta-analyses: characteristics of study designs, data, and analyses . Evid. Based Commun. Assess. Intervent. 1–25. 10.1080/17489539.2022.2089334 [ CrossRef ] [ Google Scholar ]
- * . Joo S. H., Ferron J. M., Moeyaert M., Beretvas S. N., Van den Noortgate W. (2019). Approaches for specifying the level-1 error structure when synthesizing single-case data . J. Exp. Educ. 87 , 55–74. 10.1080/00220973.2017.1409181 [ CrossRef ] [ Google Scholar ]
- * . Joo S. H., Wang Y., Ferron J., Beretvas S. N., Moeyaert M., Van Den Noortgate W. (2021). Comparison of within-and between-series effect estimates in the meta-analysis of multiple baseline studies . J. Educ. Behav. Stat. 47 , 131–166. [ Google Scholar ]
- * . Manolov R., Guilera G., Sierra V. (2014). Weighting strategies in the meta-analysis of single-case studies . Behav. Res. Methods 46 , 1152–1166. 10.3758/s13428-013-0440-0 [ PubMed ] [ CrossRef ] [ Google Scholar ]
- McNeish D., Stapleton L. M., Silverman R. D. (2017). On the unnecessary ubiquity of hierarchical linear modeling . Psychol. Methods 22 , 114. 10.1037/met0000078 [ PubMed ] [ CrossRef ] [ Google Scholar ]
- Microsoft Corporation (2018). Microsoft Excel . Retrieved from: https://office.microsoft.com/excel (accessed January 2023).
- Moeyaert M. (2019). Quantitative synthesis of research evidence: multilevel meta-analysis . Behav. Disord. 44 , 241–256. 10.1177/0198742918806926 [ CrossRef ] [ Google Scholar ]
- * . Moeyaert M., Ugille M., Ferron J. M., Beretvas S. N., den Noortgate W. V. (2014). Three-level analysis of single-case experimental data: empirical validation . J. Exp. Educ. 82 , 1–21. 10.1080/00220973.2012.745470 [ CrossRef ] [ Google Scholar ]
- * . Moeyaert M., Ugille M., Ferron J. M., Beretvas S. N., Van den Noortgate W. (2013a). The three-level synthesis of standardized single-subject experimental data: a Monte Carlo simulation study . Multivariate Behav. Res. 48 , 719–748. 10.1080/00273171.2013.816621 [ PubMed ] [ CrossRef ] [ Google Scholar ]
- * . Moeyaert M., Ugille M., Ferron J. M., Beretvas S. N., Van den Noortgate W. (2013b). Modeling external events in the three-level analysis of multiple-baseline across-participants designs: a simulation study . Behav. Res. Methods 45 , 547–559. 10.3758/s13428-012-0274-1 [ PubMed ] [ CrossRef ] [ Google Scholar ]
- * . Moeyaert M., Ugille M., Ferron J. M., Beretvas S. N., Van den Noortgate W. (2016). The misspecification of the covariance structures in multilevel models for single-case data: a Monte Carlo simulation study . J. Exp. Educ. 84 , 473–509. 10.1080/00220973.2015.1065216 [ CrossRef ] [ Google Scholar ]
- Moeyaert M., Ugille M., Ferron J. M., Onghena P., Heyvaert M., Beretvas S. N., et al.. (2015). Estimating intervention effects across different types of single-subject experimental designs: empirical illustration . Sch. Psychol. Q. 30 , 50. 10.1037/spq0000068 [ PubMed ] [ CrossRef ] [ Google Scholar ]
- Moeyaert M., Yang P., Xu X. (2022). The power to explain variability in intervention effectiveness in single-case research using hierarchical linear modeling . Perspect. Behav. Sci. 45 , 13–35 10.1007/s40614-021-00304-z [ PMC free article ] [ PubMed ] [ CrossRef ] [ Google Scholar ]
- Moeyaert M., Yang P., Xu X., Kim E. (2021). Characteristics of moderators in meta-analyses of single-case experimental design studies . Behav. Modif. 10.1177/01454455211002111 [ PubMed ] [ CrossRef ] [ Google Scholar ]
- Myung I. J. (2003). Tutorial on maximum likelihood estimation . J. Math. Psychol. 47 , 90–100. 10.1016/S0022-2496(02)00028-7 [ CrossRef ] [ Google Scholar ]
- Onghena P. (2005). “Single-case designs,” in Encyclopedia of Statistics in Behavioral Science. Vol. 4 , ed B. E. D. Howell (Chichester: Wiley; ), 1850–1854. [ Google Scholar ]
- * . Owens C. M. (2011). Meta-Analysis of Single-Case Data: A Monte Carlo Investigation of a Three Level Model. ProQuest Dissertations Publishing, University of South Florida. [ Google Scholar ]
- Page M. J., McKenzie J. E., Bossuyt P. M., Boutron I., Hoffmann T. C., Mulrow C. D., et al.. (2021). The PRISMA 2020 statement: an updated guideline for reporting systematic reviews . Syst. Rev. 10 , 1–11. 10.1186/s13643-021-01626-4 [ PMC free article ] [ PubMed ] [ CrossRef ] [ Google Scholar ]
- Pan J. X., Fang K. T. (2002). “Maximum likelihood estimation,” in Growth Curve Models and Statistical Diagnostics (New York, NY: Springer; ), 77–158. [ Google Scholar ]
- * . Petit-Bois M. (2014). A Monte Carlo Study: The Consequences of the Misspecification of the Level-1 Error Structure when Meta-Analyzing Single-Case Designs . ProQuest Dissertations Publishing, University of South Florida. [ Google Scholar ]
- * . Petit-Bois M., Baek E. K., Van den Noortgate W., Beretvas S. N., Ferron J. M. (2016). The consequences of modeling autocorrelation when synthesizing single-case studies using a three-level model . Behav. Res. Methods 48 , 803–812. 10.3758/s13428-015-0612-1 [ PubMed ] [ CrossRef ] [ Google Scholar ]
- Petrocchi N., Cosentino T., Pellegrini V., Femia G., D'Innocenzo A., Mancini F. (2021). Compassion-focused group therapy for treatment-resistant OCD: initial evaluation using a multiple baseline design . Front. Psychol. 11 , 594277. 10.3389/fpsyg.2020.594277 [ PMC free article ] [ PubMed ] [ CrossRef ] [ Google Scholar ]
- Price M., Anderson P., Henrich C. C., Rothbaum B. O. (2008). Greater expectations: Using hierarchical linear modeling to examine expectancy for treatment outcome as a predictor of treatment response . Behav. Ther. 39 , 398–405. 10.1016/j.beth.2007.12.002 [ PMC free article ] [ PubMed ] [ CrossRef ] [ Google Scholar ]
- Quzzani M., Hammady H., Fedorowicz Z., Elmagarmid A. (2016). Rayyan — a web and mobile app for systematic reviews . Syst. Rev . 5, 210. 10.1186/s13643-016-0384-4 [ PMC free article ] [ PubMed ] [ CrossRef ] [ Google Scholar ]
- Rindskopf D. (2014). Nonlinear Bayesian analysis for single case designs . J. Sch. Psychol. 52 , 179–189. 10.1016/j.jsp.2013.12.003 [ PubMed ] [ CrossRef ] [ Google Scholar ]
- Rosenbaum P. R., Everitt B. S., Howell D. (2005). Encyclopedia of Statistics in Behavioral Science . New York, NY: Wiley. [ Google Scholar ]
- Rossi R. J. (2018). Mathematical Statistics: An Introduction to Likelihood Based Inference . Hoboken, NJ: John Wiley and Sons. [ Google Scholar ]
- Shadish W. R., Kyse E. N., Rindskopf D. M. (2013). Analyzing data from single-case designs using multilevel models: new applications and some agenda items for future research . Psychol. Methods 18 , 385. 10.1037/a0032964 [ PubMed ] [ CrossRef ] [ Google Scholar ]
- Shadish W. R., Sullivan K. J. (2011). Characteristics of single-case designs used to assess intervention effects in 2008 . Behav. Res. Methods 43 , 971–980. 10.3758/s13428-011-0111-y [ PubMed ] [ CrossRef ] [ Google Scholar ]
- * . Tsai S. P. (2011). Using Multilevel Modeling in Synthesizing Single-Subject Research Data With Trend—A Monte Carlo Study . ProQuest Dissertations Publishing, University of Washington. [ Google Scholar ]
- * . Ugille M., Moeyaert M., Beretvas S. N., Ferron J., Van den Noortgate W. (2012). Multilevel meta-analysis of single-subject experimental designs: a simulation study . Behav. Res. Methods 44 , 1244–1254. 10.3758/s13428-012-0213-1 [ PubMed ] [ CrossRef ] [ Google Scholar ]
- * . Ugille M., Moeyaert M., Beretvas S. N., Ferron J. M., Van den Noortgate W. (2014). Bias corrections for standardized effect size estimates used with single-subject experimental designs . J. Exp. Educ. 82 , 358–374. 10.1080/00220973.2013.813366 [ CrossRef ] [ Google Scholar ]
- Van den Noortgate W., Onghena P. (2003a). A parametric bootstrap version of Hedges' homogeneity test . J. Mod. Appl. Stat. Methods 2 , 7. 10.22237/jmasm/1051747620 [ CrossRef ] [ Google Scholar ]
- Van den Noortgate W., Onghena P. (2003b). Combining single-case experimental data using hierarchical linear models . Sch. Psychol. Q. 18 , 325. 10.1521/scpq.18.3.325.22577 [ CrossRef ] [ Google Scholar ]
- Van den Noortgate W., Onghena P. (2007). The aggregation of single-case results using hierarchical linear models . Behav. Anal. Today 8 , 196. 10.1037/h0100613 [ CrossRef ] [ Google Scholar ]
- Van den Noortgate W., Onghena P. (2008). A multilevel meta-analysis of single-subject experimental design studies . Evid. Based Commun. Assess. Interv. 2 , 142–151 10.1080/17489530802505362 [ CrossRef ] [ Google Scholar ]
Log in using your username and password
- Search More Search for this keyword Advanced search
- Latest content
- Current issue
- For authors
- New editors
- BMJ Journals
You are here
- Online First
- Single case experimental design: a rigorous method for addressing inequity and enhancing precision within Para sport and exercise medicine research
- Article Text
- Article info
- Citation Tools
- Rapid Responses
- Article metrics

- http://orcid.org/0000-0002-2011-3382 Sean Tweedy 1 , 2 ,
- Iain Mayank Dutia 1 , 3 ,
- John Cairney 1 , 2 ,
- Emma Beckman 1 , 4
- 1 The School of Human Movement and Nutrition Sciences , The University of Queensland , Saint Lucia , Queensland , Australia
- 2 The Queensland Centre for Olympic and Paralympic Studies , The University of Queensland - St Lucia Campus , Brisbane , Queensland , Australia
- 3 School of Allied Health , Australian Catholic University, Banyo Campus , Brisbane , Queensland , Australia
- 4 Para sport , Queensland Academy of Sport , Sunnybank , Queensland , Australia
- Correspondence to Dr Sean Tweedy; s.tweedy{at}uq.edu.au
https://doi.org/10.1136/bjsports-2024-108587
Statistics from Altmetric.com
Request permissions.
If you wish to reuse any or all of this article please use the link below which will take you to the Copyright Clearance Center’s RightsLink service. You will be able to get a quick price and instant permission to reuse the content in many different ways.
- Disabled Persons
- Neurological rehabilitation
Approximately 4400 athletes from 184 nations competed in 22 sports at the 2024 Paris Paralympic Games. However, it is recognised that athletes with more severe disabilities and high support needs are under-represented in sport, and strategies to increase representation are required. Focusing on individuals with cerebral palsy (CP), we present evidence that people with high support needs are also under-represented in Para sport and exercise medicine (P-SEM) research. We outline why single case experimental designs (SCEDs) are a rigorous and effective means of addressing under-representation in P-SEM research.
Cerebral palsy
CP is an eligible underlying health condition for 17 of the 22 Paralympic sports. It results from a non-progressive brain lesion and is defined as a heterogeneous group of permanent disorders affecting movement and posture. 1 CP heterogeneity is multidimensional and can be classified based on:
Neurological subtype: Spastic CP (quadriplegia/diplegia/hemiplegia); dyskinetic; ataxic; and mixed. 2 Subtypes vary in severity and anatomical distribution.
Functional effects: The Gross Motor Function Classification System (GMFCS) is the most common and has five levels: GMFCS level I (least severe) and II are able to walk independently; GMFCS IV/V use wheeled mobility and typically have high support needs (CP-HSN).
Exercise training research in people with CP
At least three major reviews have analysed randomised controlled trials (RCTs) that evaluate exercise training in people with CP and all report that CP-HSN participants are under-represented. 4–6 CP-HSN constitutes approximately 30% of the CP population 7 but …
Contributors ST is the guarantor of this work. All authors made substantial contributions to conception of the work, provided critical revisions, approved the final version and agreed to be accountable for the accuracy and integrity of the work.
Funding The authors have not declared a specific grant for this research from any funding agency in the public, commercial or not-for-profit sectors.
Competing interests ST, JC and EB work for The University of Queensland, the Official Higher Education Partner for Paralympics Australia. EB is on secondment as Head of Parasport with the Queensland Academy of Sport and Paralympics Australia. ST is a member of the International Paralympic Committee’s Classification Compliance and Oversight Committee and a member of World Para Athletics Classification Advisory Group.
Provenance and peer review Not commissioned; externally peer reviewed.
Read the full text or download the PDF:

An official website of the United States government
The .gov means it’s official. Federal government websites often end in .gov or .mil. Before sharing sensitive information, make sure you’re on a federal government site.
The site is secure. The https:// ensures that you are connecting to the official website and that any information you provide is encrypted and transmitted securely.
- Publications
- Account settings
- My Bibliography
- Collections
- Citation manager
Save citation to file
Email citation, add to collections.
- Create a new collection
- Add to an existing collection
Add to My Bibliography
Your saved search, create a file for external citation management software, your rss feed.
- Search in PubMed
- Search in NLM Catalog
- Add to Search
Single-case experimental designs to assess intervention effectiveness in rehabilitation: A practical guide
Affiliations.
- 1 Institut universitaire de réadaptation Clemenceau-Strasbourg, 45, boulevard Clemenceau, 67082 Strasbourg, France; Service de chirurgie orthopédique infantile, hôpital de Hautepierre, CHU de Strasbourg, avenue Molière, 67098 Strasbourg, France; GRC handicap cognitif et réadaptation (HanCRe), hôpitaux universitaires Pitié-Salpêtière, 75013 Paris, France. Electronic address: [email protected].
- 2 Institute of Health and Wellbeing, University of Glasgow, The Academic Centre, Gartnavel Royal Hospital, 1055 Great Western Road, Glasgow G12 0XH, United Kingdom.
- PMID: 29253607
- DOI: 10.1016/j.rehab.2017.12.002
Single-case experimental designs (SCED) are experimental designs aiming at testing the effect of an intervention using a small number of patients (typically one to three), using repeated measurements, sequential (±randomized) introduction of an intervention and method-specific data analysis, including visual analysis and specific statistics. The aim of this paper is to familiarise professionals working in different fields of rehabilitation with SCEDs and provide practical advice on how to design and implement a SCED in clinical rehabilitation practice. Research questions suitable for SCEDs and the different types of SCEDs (e.g., alternating treatment designs, introduction/withdrawal designs and multiple baseline designs) are reviewed. Practical steps in preparing a SCED design are outlined. Examples from different rehabilitation domains are provided throughout the paper. Challenging issues such as the choice of the repeated measure, assessment of generalisation, randomization, procedural fidelity, replication and generalizability of findings are discussed. Simple rules and resources for data analysis are presented. The utility of SCEDs in physical and rehabilitation medicine (PRM) are discussed.
Keywords: Alternating treatment; Methodology; Multiple baseline; Rehabilitation; Single-case.
Copyright © 2018 Elsevier Masson SAS. All rights reserved.
PubMed Disclaimer
Similar articles
- Single-case experimental designs for child neurological rehabilitation and developmental disability research. Krasny-Pacini A. Krasny-Pacini A. Dev Med Child Neurol. 2023 May;65(5):611-624. doi: 10.1111/dmcn.15513. Epub 2023 Jan 31. Dev Med Child Neurol. 2023. PMID: 36721909 Review.
- A practitioner's guide to conducting and analysing embedded randomized single-case experimental designs. Tanious R, Manolov R. Tanious R, et al. Neuropsychol Rehabil. 2023 May;33(4):613-645. doi: 10.1080/09602011.2022.2035774. Epub 2022 Feb 18. Neuropsychol Rehabil. 2023. PMID: 35179088
- Single case experimental designs: introduction to a special issue of Neuropsychological Rehabilitation. Evans JJ, Gast DL, Perdices M, Manolov R. Evans JJ, et al. Neuropsychol Rehabil. 2014;24(3-4):305-14. doi: 10.1080/09602011.2014.903198. Epub 2014 Apr 25. Neuropsychol Rehabil. 2014. PMID: 24766415
- A systematic review of applied single-case research published between 2016 and 2018: Study designs, randomization, data aspects, and data analysis. Tanious R, Onghena P. Tanious R, et al. Behav Res Methods. 2021 Aug;53(4):1371-1384. doi: 10.3758/s13428-020-01502-4. Epub 2020 Oct 26. Behav Res Methods. 2021. PMID: 33104956
- Characteristics of Missing Data in Single-Case Experimental Designs: An Investigation of Published Data. Aydin O. Aydin O. Behav Modif. 2024 Mar;48(2):182-215. doi: 10.1177/01454455231212265. Epub 2023 Nov 17. Behav Modif. 2024. PMID: 37978822 Review.
- Feasibility of a home-based home videogaming intervention with a family-centered approach for children with cerebral palsy: a randomized multiple baseline single-case experimental design. Chan-Víquez D, Fernández-Huertas H, Montserrat-Gonzalez C, Khan A, Fehlings D, Munce S, Wright FV, Biddiss E. Chan-Víquez D, et al. J Neuroeng Rehabil. 2024 Sep 4;21(1):151. doi: 10.1186/s12984-024-01446-2. J Neuroeng Rehabil. 2024. PMID: 39227911 Free PMC article. Clinical Trial.
- Assistive technology for adults on the autism spectrum: A systematic survey. Wang M, Jeon M. Wang M, et al. Int J Hum Comput Interact. 2024;40(10):2433-2452. doi: 10.1080/10447318.2022.2163568. Epub 2023 Jan 8. Int J Hum Comput Interact. 2024. PMID: 38784821 Free PMC article.
- Opening the dialogue: A preliminary exploration of hair color, hair cleanliness, light, and motion effects on fNIRS signal quality. Holmes M, Aalto D, Cummine J. Holmes M, et al. PLoS One. 2024 May 23;19(5):e0304356. doi: 10.1371/journal.pone.0304356. eCollection 2024. PLoS One. 2024. PMID: 38781258 Free PMC article.
- The cognitive remediation of attention in HIV-associated neurocognitive disorders (HAND): A meta-analysis and systematic review. Zondo S. Zondo S. F1000Res. 2023 Sep 11;12:1133. doi: 10.12688/f1000research.132166.1. eCollection 2023. F1000Res. 2023. PMID: 38778812 Free PMC article.
- Aide à la décision pour le dépistage du cancer du sein: Étude de cas pré-test/post-test. McDonald M, Stacey D. McDonald M, et al. Can Oncol Nurs J. 2024 Apr 1;34(2):158-165. doi: 10.5737/23688076342158. eCollection 2024 Spring. Can Oncol Nurs J. 2024. PMID: 38706657 Free PMC article. French.
- Search in MeSH
LinkOut - more resources
Full text sources.
- ClinicalKey
- Elsevier Science
- Enlighten: Publications, University of Glasgow
Other Literature Sources
- scite Smart Citations
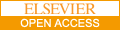
- Citation Manager
NCBI Literature Resources
MeSH PMC Bookshelf Disclaimer
The PubMed wordmark and PubMed logo are registered trademarks of the U.S. Department of Health and Human Services (HHS). Unauthorized use of these marks is strictly prohibited.
Select language

Working at Utrecht University
Phd position in bayesian evaluation of single case experimental designs.
The NWO-funded project “Accelerating the development of effective psychological treatments for traumatised refugees using Bayesian methodology” is looking for a PhD candidate focused on Bayesian evaluation of single case experimental designs.
In single case experimental designs (SCEDs), as applied in therapy effect evaluation, 10-15 measurements of 2-4 outcome variables are typically taken over time. Despite the relative small amount of data, a powerful evaluation can be obtained if (i) the therapy effect on all the outcome variables is evaluated simultaneously, and (ii) the direction of the therapy effect on the outcome variables is specified. Therefore, a Bayesian approach for the evaluation of SCEDs will be developed in this PhD project.
To achieve this, Utrecht University and ARQ are collaborating in a new NWO-funded project “Accelerating the development of effective psychological treatments for traumatised refugees using Bayesian methodology”. This project is focused on further developing interventions for traumatised refugees and evaluating these in small-scale studies.
The project encompasses two PhD projects: one focused on Bayesian evaluation of single case experimental designs (that is, the methodology that will be used for therapy effect evaluation) and the other focused on treatment development and evaluation. The current vacancy applies to Bayesian evaluation of SCEDs.
As a PhD candidate in this project, your responsibilities will include:
- developing, evaluating and applying Bayesian evaluation of single case experimental designs (to real data resulting from ARQ Centrum'45 );
- developing open-access software in R so that therapists and clinical psychologists can use the statistical tools that will be developed;
- writing up findings for publication;
- presenting research findings at conferences and workshops;
- being an active member of the PhD network IOPS (attending courses, giving presentations);
- attending classes and seminars (including those offered at other universities) to further develop your statistical and research skills;
- conducting teaching (10% of the appointment), including undergraduate tutorials.
Your qualities
This position may be the right fit for you, if you:
- hold (or nearly hold) a Master’s degree in Methodology and Statistics, data science, Econometrics, Psychometrics or a related field;
- have programming skills in R;
- have adequate verbal and written communication skills in English;
- are interested in working in an interdisciplinary team consisting of clinical psychologists, therapists, programmers, and statisticians;
- are interested in the problems of, and mental health care for traumatised vulnerable groups
- a position for four years (a working week of 38 hours) or five years (a working week of 30.4 hours) with a trial period of one year;
- a full-time gross monthly salary between €2,872 in the first year and €3,670 in the fourth year (salary scale P under the Collective Labour Agreement for Dutch Universities (CAO NU));
- 8% holiday pay and 8.3% year-end bonus;
- a pension scheme, partially paid parental leave and flexible terms of employment based on the CAO NU.
In addition to the terms of employment laid down in the CAO NU, Utrecht University has a number of schemes and facilities of its own for employees. This includes schemes facilitating professional development , leave schemes and schemes for sports and cultural activities , as well as discounts on software and other IT products. We also offer access to additional employee benefits through our Terms of Employment Options Model. In this way, we encourage our employees to continue to invest in their growth. For more information, please visit Working at Utrecht University .
A better future for everyone. This ambition motivates our scientists in executing their leading research and inspiring teaching. At Utrecht University , the various disciplines collaborate intensively towards major strategic themes . Our focus is on Dynamics of Youth, Institutions for Open Societies, Life Sciences and Pathways to Sustainability. Sharing science, shaping tomorrow .
The Faculty of Social and Behavioural Sciences is one of the leading faculties in Europe providing research and academic teaching in cultural anthropology, educational sciences, interdisciplinary social science, pedagogical sciences, psychology, and sociology. Almost 7,000 students are enrolled in a broad range of undergraduate and graduate programmes. The Faculty of Social and Behavioural Sciences has some 1,100 faculty and staff members, all providing their individual contribution to the training and education of young talent and to the research into and finding solutions for scientific and societal issues. The PhD candidate employed for this vacancy will be based at the Department of Methodology and Statistics.
The faculty strives for diversity among its employees and students and is committed to creating a safe and inclusive environment for everyone, as can be read in Utrecht University's Equality, Diversity and Inclusion policy.
The faculty attaches great importance to the fact that its employees can be widely deployed in the university organisation, now and in the future, to further professionalise the support of education and research. To encourage this, every employee is given the time and facilities – for example in the form of training – at some point in their career to participate in projects or work in other departments. Characteristics that the faculty considers important are involvement, the ability to collaborate and flexibility.
The faculty is located at Utrecht Science Park near the historical city centre of Utrecht.
More information
For more information about this position, please contact Kevin van Kats at [email protected] . The full project description as funded by NWO can be obtained by sending an e-mail to Prof. Herbert Hoijtink at [email protected]
This vacancy applies to the methods and statistics that will be used for therapy effect evaluation. Are you more interested in posttraumatic stress and improving and evaluating interventions for vulnerable groups, together with healthcare professionals who carry out treatments on a daily basis? Then the other PhD position may be interesting to you. You can check out that vacancy through this link .
Please note that international candidates that need a visa/work permit for the Netherlands require at least four months processing time after selection and acceptance. Our International Service Desk (ISD) can answer your questions about living in the Netherlands as international staff . Finding appropriate housing in or near Utrecht is your own responsibility, but the ISD may be able to advise you therewith. In case of general questions about working and living in The Netherlands, please consult the Dutch Mobility Portal.
Candidates for this vacancy will be recruited by Utrecht University.
As Utrecht University, we want to be a home for everyone. We value staff with diverse backgrounds, perspectives and identities, including cultural, religious or ethnic background, gender, sexual orientation, disability or age. We strive to create a safe and inclusive environment in which everyone can flourish and contribute.
To apply, please send your curriculum vitae, including a letter of motivation, via the ‘apply now’ button. Please combine your curriculum vitae and letter of motivation in one document. If the application system requires you to upload two separate documents, simply upload this one document twice.
The first round of interviews will take place in October 2024. The starting date is end of 2024 or early in 2025.
The application deadline is 6 October 2024.
- Share on LinkedIn
- Share on Facebook
- Share on WhatsApp
- Share via email
Utrecht University Heidelberglaan 8 3584 CS Utrecht The Netherlands Tel. +31 (0)30 253 35 50
Magnet.me - Het slimme netwerk waarop hbo‑ en wo‑studenten hun baan of stage vinden.
Het slimme netwerk waarop hbo‑ en wo‑studenten hun baan of stage vinden.
PhD position in Bayesian Evaluation of Single Case Experimental Designs
Je carrière begint op Magnet.me
Maak een profiel aan en ontvang slimme aanbevelingen op basis van je gelikete vacatures.
The NWO-funded project “Accelerating the development of effective psychological treatments for traumatised refugees using Bayesian methodology” is looking for a PhD candidate focused on Bayesian evaluation of single case experimental designs.
In single case experimental designs (SCEDs), as applied in therapy effect evaluation, 10-15 measurements of 2-4 outcome variables are typically taken over time. Despite the relative small amount of data, a powerful evaluation can be obtained if (i) the therapy effect on all the outcome variables is evaluated simultaneously, and (ii) the direction of the therapy effect on the outcome variables is specified. Therefore, a Bayesian approach for the evaluation of SCEDs will be developed in this PhD project.
To achieve this, Utrecht University and ARQ are collaborating in a new NWO-funded project “Accelerating the development of effective psychological treatments for traumatised refugees using Bayesian methodology”. This project is focused on further developing interventions for traumatised refugees and evaluating these in small-scale studies.The project encompasses two PhD projects: one focused on Bayesian evaluation of single case experimental designs (that is, the methodology that will be used for therapy effect evaluation) and the other focused on treatment development and evaluation. The current vacancy applies to Bayesian evaluation of SCEDs.
As a PhD candidate in this project, your responsibilities will include:
- developing, evaluating and applying Bayesian evaluation of single case experimental designs (to real data resulting from ARQ Centrum'45);
- developing open-access software in R so that therapists and clinical psychologists can use the statistical tools that will be developed;
- writing up findings for publication;
- presenting research findings at conferences and workshops;
- being an active member of the PhD network IOPS (attending courses, giving presentations);
- attending classes and seminars (including those offered at other universities) to further develop your statistical and research skills;
- conducting teaching (10% of the appointment), including undergraduate tutorials.
Your qualities
This position may be the right fit for you, if you:
- hold (or nearly hold) a Master’s degree in Methodology and Statistics, data science, Econometrics, Psychometrics or a related field;
- have programming skills in R;
- have adequate verbal and written communication skills in English;
- are interested in working in an interdisciplinary team consisting of clinical psychologists, therapists, programmers, and statisticians;
- are interested in the problems of, and mental health care for traumatised vulnerable groups
- a position for four years (a working week of 38 hours) or five years (a working week of 30.4 hours) with a trial period of one year;
- a full-time gross monthly salary between €2,872 in the first year and €3,670 in the fourth year (salary scale P under the Collective Labour Agreement for Dutch Universities (CAO NU));
- 8% holiday pay and 8.3% year-end bonus;
- a pension scheme, partially paid parental leave and flexible terms of employment based on the CAO NU.
We work on a better future. In order to do that, we join forces with academics, students, alumni, social partners, the government and the corporate world. Together, we look for sustainable solutions to the big challenges of today and tomorrow.
Anderen bekeken ook
Case Manager
Amsterdam, NL
PhD position in Global rap performance
University of Groningen
Groningen, NL
PhD Position: Transformative Potential of Climate Mitigation Strategies
Radboud Universiteit
Nijmegen, NL
PhD position ‘Survivor. The selection process of political elites in Europe’
Vrije Universiteit Amsterdam
PhD Position Immersive Lab
Delft University of Technology
PhD Position on Speculative Fiction and Imaginaries of the Future
University of Amsterdam
PhD position: ultrafast broadband-THz spectroscopy of perovskite materials
Universiteit Leiden
PhD Position: Burden of Zoonoses in Europe
Utrecht University
Utrecht, NL
Bekijk ons aanbod:
- Traineeships
- HBO Traineeships
- WO Traineeships
- Afstudeerstages
- Business courses
- Inhousedagen
- Alle locaties
- Meest populair
Direct naar:
- Communicatie stages
- Marketing stages
- Consultancy stages
- ICT traineeships
- Consultancy traineeships
- Motivatiebrief - een complete handleiding
- Solliciteren voor een stage - een complete gids
- 20 sollicitatievragen die gesteld gaan worden tijdens je volgende sollicitatiegesprek
- Alles wat je moet weten over een stagevergoeding
- Wat is een traineeship?
- Wat is campus recruitment en hoe zet ik het in?
- Careers guide
- Over ons (pers)
- Voor werkgevers
- Privacybeleid & Gebruikersvoorwaarden
- Werken bij Magnet.me

Change language to: English
Deze pagina is geoptimaliseerd voor mensen uit Nederland. Bekijk de versie geoptimaliseerd voor mensen uit het Verenigd Koninkrijk.
Advertisement
Optimization strategies for enhanced production of single cell protein: recent advances and perspectives
- Review Paper
- Published: 19 September 2024
Cite this article
- Sharda Devi Rajput 1 ,
- Neha Pandey 1 &
- S. Keshavkant ORCID: orcid.org/0000-0003-3679-479X 1
The increasing demand for protein supplementation in both animal and human nutrition, coupled with the limitations of conventional protein sources, necessitates research into sustainable alternatives. Single-cell proteins (SCPs) are the dried biomass of microorganisms such as algae, yeast, and bacteria that are cultured under controlled conditions. Production of SCP has emerged as a promising solution, offering advantages such as rapid production, minimal land requirement, and adaptability to diverse climatic conditions; however, their large-scale production requires meticulous optimization of entire process of production. Efficient optimization enhances productivity, product quality, and cost-efficiency, making SCP production economically viable, safer and sustainable. Optimization involves standardization of various regulating factors such as temperature, pH, nutrient availability and type, oxygen level, agitation, etc., which requires a large number of experimental trials and a high consumption of resources. To overcome these challenges, optimization of SCP production is increasingly using multivariate statistical techniques, including response surface methodology (RSM), and factorial design. Computer modelling and simulation techniques offer insights into the complex dynamics of production systems. This review discusses popularly followed strategies for optimization of SCP production, beginning with an overview of the fundamentals and significance of SCP. Methods of optimization, including classical methods and RSM, along with integration of mathematical modelling into the Design of Experiment (DoE), are then examined. Case studies have also been discussed to illustrate successful optimization approaches while addressing applications of SCP and, challenges and future directions in SCP optimization/ production.
Graphical abstract
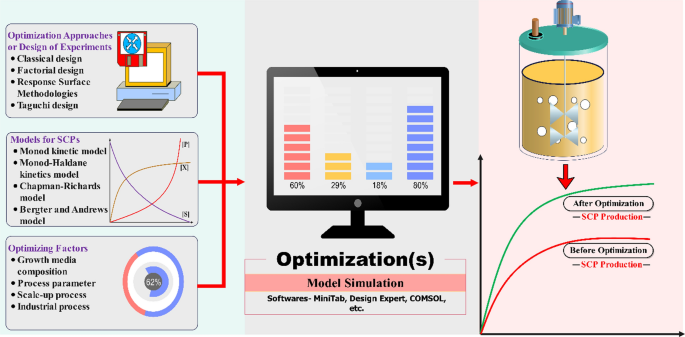
This is a preview of subscription content, log in via an institution to check access.
Access this article
Subscribe and save.
- Get 10 units per month
- Download Article/Chapter or eBook
- 1 Unit = 1 Article or 1 Chapter
- Cancel anytime
Price includes VAT (Russian Federation)
Instant access to the full article PDF.
Rent this article via DeepDyve
Institutional subscriptions
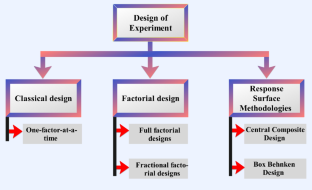
Similar content being viewed by others

Recent advances and challenges in single cell protein (SCP) technologies for food and feed production
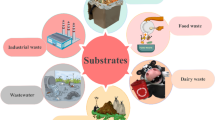
A comprehensive report on valorization of waste to single cell protein: strategies, challenges, and future prospects
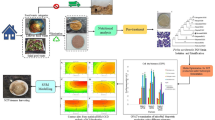
Statistical Evaluation for the Formulation of Low-Cost Single Cell Protein Production in Food Waste Medium by Pichia occidentalis : A Sustainable Valorization Approach
Abalos A, Maximo F, Manresa MA, Bastida J (2002) Utilization of response surface methodology to optimize the culture media for the production of rhamnolipids by Pseudomonas aeruginosa AT10. Journal of Chemical Technology & Biotechnology: International Research in Process, Environmental & Clean Technology 77(7):777–784. https://doi.org/10.1002/jctb.637
Article CAS Google Scholar
Agboola JO, Lapeña D, Øverland M, Arntzen MØ, Mydland LT, Hansen JØ (2022) Yeast as a novel protein source - Effect of species and autolysis on protein and amino acid digestibility in Atlantic salmon (Salmo salar). Aquaculture 546:737312. https://doi.org/10.1016/j.aquaculture.2021.737312
Ahnan-Winarno AD, Cordeiro L, Winarno FG, Gibbons J, Xiao H (2021) Tempeh: A semicentennial review on its health benefits, fermentation, safety, processing, sustainability, and affordability. Compr Rev Food Sci Food Saf 20(2):1717–1767. https://doi.org/10.1002/jctb.637
Al-Hadithi OAA, Alrawi D, Qais M (2018) Single cell protein production by soil Raoutella ornithinolytica incubated on waste potato, paper and corn cob products
Ali S, Mushtaq J, Nazir F, Sarfraz H (2017) Production and processing of single cell protein (SCP)-a review. European Journal of Pharmaceutical and Medical Research 4(7):86–94
Google Scholar
Alvarado KA, García Martínez JB, Matassa S, Egbejimba J, Denkenberger D (2021) Food in space from hydrogen-oxidizing bacteria. Acta Astronaut 180:260–265. https://doi.org/10.1016/j.actaastro.2020.12.009
Alves SC, Díaz-Ruiz E, Lisboa B, Sharma M, Mussatto SI, Thakur VK, Kalaskar DM, Gupta VK, Chandel AK (2023) Microbial meat: A sustainable vegan protein source produced from agri-waste to feed the world. Food Research International 166:112596. https://doi.org/10.1016/j.foodres.2023.112596
Amara AA, El-Baky NA (2023) Fungi as a Source of Edible Proteins and Animal Feed. Journal of Fungi 9(1):73. https://doi.org/10.3390/jof9010073
Amorim ML, Soares J, dos Coimbra JS, R, Leite M de O, Albino LFT, Martins MA, (2021) Microalgae proteins: production, separation, isolation, quantification, and application in food and feed. Crit Rev Food Sci Nutr 61(12):1976–2002. https://doi.org/10.1080/10408398.2020.1768046
Anupama RP (2000) Value-added food: Single cell protein. Biotechnol Adv 18(6):459–479. https://doi.org/10.1016/S0734-9750(00)00045-8
Anvari M, Khayati G (2011) Submerged yeast fermentation of cheese whey for protein production and nutritional profile analysis. Adv J Food Sci Technol 3(2):122–126
CAS Google Scholar
Apandi NM, Mohamed RMSR, Latiffi NAA, Rozlan NFM, Al-Gheethi AAS (2017) Protein and lipid content of microalgae Scenedesmus sp. biomass grown in wet market wastewater. In: MATEC web of conferences. EDP Sciences 06011
Areniello M, Matassa S, Esposito G, Lens PNL (2023) Biowaste upcycling into second-generation microbial protein through mixed-culture fermentation. Trends Biotechnol 41(2):197–213. https://doi.org/10.1016/j.tibtech.2022.07.008
Aruna TE, Aworh OC, Raji AO, Olagunju AI (2017) Protein enrichment of yam peels by fermentation with Saccharomyces cerevisiae (BY4743). Annals of Agricultural Sciences 62(1):33–37. https://doi.org/10.1016/j.aoas.2017.01.002
Article Google Scholar
Asghar A, Abdul Raman AA, Daud WMAW (2014) A Comparison of Central Composite Design and Taguchi Method for Optimizing Fenton Process. Scientific World Journal 2014:1–14. https://doi.org/10.1155/2014/869120
Barragán-Trinidad M, Guadarrama-Pérez O, Guillén-Garcés RA, Bustos-Terrones V, Trevino-Quintanilla LG, Moeller-Chávez G (2023) The Grey-Taguchi Method, a Statistical Tool to Optimize the Photo-Fenton Process: A Review. Water (Basel) 15(15):2685. https://doi.org/10.3390/w15152685
Bedoya MG, Montoya DR, Tabilo-Munizaga G, Pérez-Won M, Lemus-Mondaca R (2022) Promising perspectives on novel protein food sources combining artificial intelligence and 3D food printing for food industry. Trends Food Sci Technol 128:38–52. https://doi.org/10.1016/j.tifs.2022.05.013
Beg S, Akhter S (2021) Box–Behnken Designs and Their Applications in Pharmaceutical Product Development. In: Design of Experiments for Pharmaceutical Product Development. Springer Singapore, Singapore 77–85
Bekatorou A, Psarianos C, Koutinas AA (2006) Production of food grade yeasts. Food Technol Biotechnol 44(3)
Berger PD, Maurer RE, Celli GB (2018) Designs with Factors at Three Levels. Experimental Design. Springer International Publishing, Cham, pp 423–448
Chapter Google Scholar
Bertrand B, Martínez-Morales F, Rosas-Galván N, Morales-Guzmán D, Trejo-Hernández M (2018) Statistical Design, a Powerful Tool for Optimizing Biosurfactant Production: A Review. Colloids and Interfaces 2(3):36. https://doi.org/10.3390/colloids2030036
Bezerra MA, Santelli RE, Oliveira EP, Villar LS, Escaleira LA (2008) Response surface methodology (RSM) as a tool for optimization in analytical chemistry. Talanta 76(5):965–977. https://doi.org/10.1016/j.talanta.2008.05.019
Borujeni NE, Karimi K, Denayer JFM, Kumar R (2022) Apple pomace biorefinery for ethanol, mycoprotein, and value-added biochemicals production by Mucor indicus. Energy 240:122469. https://doi.org/10.1016/j.energy.2021.122469
del Carmen Carranza-Méndez R, Sepúlveda-Torre L, Ramos-González R, Verma DK, Baranwal D, Aguilar CN, Chávez-González ML (2022) Agro-industrial wastes for production of single-cell protein (SCP). In: Innovations in Fermentation and Phytopharmaceutical Technologies. Elsevier 381–396
Carranza-Méndez RC, Chávez-González ML, Sepúlveda-Torre L, Aguilar CN, Govea-Salas M, Ramos-González R (2022) Production of single cell protein from orange peel residues by Candida utilis. Biocatal Agric Biotechnol 40:102298. https://doi.org/10.1016/j.bcab.2022.102298
Chen W-H, Biswas PP, Ubando AT, Park Y-K, Ashokkumar V, Chang J-S (2023) Design of experiment for hydrogen production from ethanol reforming: A state-of-the-art review. Fuel 342:127871. https://doi.org/10.1016/j.fuel.2023.127871
Chilakamarry CR, Sakinah AMM, Zularisam AW, Sirohi R, Khilji IA, Ahmad N, Pandey A (2022) Advances in solid-state fermentation for bioconversion of agricultural wastes to value-added products: Opportunities and challenges. Bioresour Technol 343:126065. https://doi.org/10.1016/j.biortech.2021.126065
Corradini MG, Peleg M (2007) The non-linear kinetics of microbial inactivation and growth in foods. In: Modelling Microorganisms in Food. Elsevier 129–160
del Rodero M, R, Magdalena JA, Steyer J-P, Escudié R, Capson-Tojo G, (2024) Potential of enriched phototrophic purple bacteria for H2 bioconversion into single cell protein. Sci Total Environ 908:168471. https://doi.org/10.1016/J.SCITOTENV.2023.168471
Ding H, Li J, Deng F, Huang S, Zhou P, Liu X, Li Z, Li D (2023) Ammonia nitrogen recovery from biogas slurry by SCP production using Candida utilis. J Environ Manage 325:116657. https://doi.org/10.1016/j.jenvman.2022.116657
dos Reis KC, Coimbra JM, Duarte WF, Schwan RF, Silva CF (2019) Biological treatment of vinasse with yeast and simultaneous production of single-cell protein for feed supplementation. Int J Environ Sci Technol 16(2):763–774. https://doi.org/10.1007/s13762-018-1709-8
Dunuweera AN, Nikagolla DN, Ranganathan K (2021) Fruit waste substrates to produce single-cell proteins as alternative human food supplements and animal feeds using baker’s yeast (Saccharomyces cerevisiae). J Food Qual 2021:1–6. https://doi.org/10.1155/2021/9932762
Efthymiou M-N, Tsouko E, Papagiannopoulos A, Athanasoulia I-G, Georgiadou M, Pispas S, Briassoulis D, Tsironi T, Koutinas A (2022) Development of biodegradable films using sunflower protein isolates and bacterial nanocellulose as innovative food packaging materials for fresh fruit preservation. Sci Rep 12(1):6935. https://doi.org/10.1038/s41598-022-10913-6
Ferreira SLC, Bruns RE, da Silva EGP, Dos Santos WNL, Quintella CM, David JM, de Andrade JB, Breitkreitz MC, Jardim ICSF, Neto BB (2007) Statistical designs and response surface techniques for the optimization of chromatographic systems. J Chromatogr A 1158(1–2):2–14. https://doi.org/10.1016/j.chroma.2007.03.051
Ferreira JA, Lennartsson PR, Taherzadeh MJ (2014) Production of ethanol and biomass from thin stillage using food-grade Zygomycetes and Ascomycetes filamentous fungi. Energies (Basel) 7(7):3872–3885. https://doi.org/10.3390/en7074199
Florez-Orrego D, Sharma S, Navabi S (2022) Editorial: Integration and optimization in the chemical process industry. Frontiers in Chemical Engineering. https://doi.org/10.3389/fceng.2022.961022
Garavand F, Rouhi M, Razavi SH, Cacciotti I, Mohammadi R (2017) Improving the integrity of natural biopolymer films used in food packaging by crosslinking approach: A review. Int J Biol Macromol 104:687–707. https://doi.org/10.1016/j.ijbiomac.2017.06.093
Gomes TA, Zanette CM, Spier MR (2020) An overview of cell disruption methods for intracellular biomolecules recovery. Prep Biochem Biotechnol 50(7):635–654. https://doi.org/10.1080/10826068.2020.1728696
Gómez-Camacho CE, Mollea C, Mazzarino I, Ruggeri B, Bosco F (2022) Repurposing Tempeh Fermentation: a Promising Protein Source Using Food Residues and Edible Filamentous Fungi. Chem Eng Trans 93:37–42. https://doi.org/10.3303/CET2293007
Hamidoghli A, Yun H, Won S, Kim S, Farris NW, Bai SC (2019) Evaluation of a single-cell protein as a dietary fish meal substitute for whiteleg shrimp Litopenaeus vannamei. Fisheries science 85(1):147–155. https://doi.org/10.1007/s12562-018-1275-5
Hardy RW, Patro B, Pujol-Baxley C, Marx CJ, Feinberg L (2018) Partial replacement of soybean meal with Methylobacterium extorquens single-cell protein in feeds for rainbow trout (Oncorhynchus mykiss Walbaum). Aquac Res 49(6):2218–2224. https://doi.org/10.1111/are.13678
Hemmat Esfe M, Rostamian H, Shabani-samghabadi A, Abbasian Arani AA (2017) Application of three-level general factorial design approach for thermal conductivity of MgO/water nanofluids. Appl Therm Eng 127:1194–1199. https://doi.org/10.1016/j.applthermaleng.2017.07.211
Hezarjaribi M, Ardestani F, Ghorbani HR (2016) Single Cell Protein Production by Saccharomyces cerevisiae Using an Optimized Culture Medium Composition in a Batch Submerged Bioprocess. Appl Biochem Biotechnol 179(8):1336–1345. https://doi.org/10.1007/s12010-016-2069-9
Hosseini SM, Khosravi-Darani K (2011) Response Surface Methodology for Mycoprotein Production by Fusarium Venenatum ATCC 20334. J Bioprocess Biotech 01:01. https://doi.org/10.4172/2155-9821.1000102
Hosseini SM, Khosravi-Darani K, Mohammadifar MA, Nikoopour H (2009) Production of mycoprotein by Fusarium venenatum growth on modified vogel medium. Asian J Chem 21(5):4017
Ismail S, Giacinti G, Raynaud CD, Cameleyre X, Alfenore S, Guillouet S, Gorret N (2024) Impact of the environmental parameters on single cell protein production and composition by Cupriavidus necator. J Biotechnol 388:83–95. https://doi.org/10.1016/j.jbiotec.2024.04.009
Jach ME, Baj T, Juda M, Świder R, Mickowska B, Malm A (2020) Statistical evaluation of growth parameters in biofuel waste as a culture medium for improved production of single cell protein and amino acids by Yarrowia lipolytica. AMB Express 10:1. https://doi.org/10.1186/s13568-020-00968-x
Jach ME, Serefko A, Ziaja M, Kieliszek M (2022) Yeast protein as an easily accessible food source. Metabolites 12(1):63. https://doi.org/10.3390/metabo12010063
Jalasutram V, Kataram S, Gandu B, Anupoju GR (2013) Single cell protein production from digested and undigested poultry litter by Candida utilis: optimization of process parameters using response surface methodology. Clean Technol Environ Policy 15(2):265–273. https://doi.org/10.1007/s10098-012-0504-3
Jeder H, Deschamps AM, Lebeault JM (1987) Production of single-cell protein with cellulomonas sp on hempstalk wastes. Acta Biotechnol 7(2):103–109. https://doi.org/10.1002/abio.370070202
Jin Q (2023) Building microbial kinetic models for environmental application: A theoretical perspective. Appl Geochem 158:105782. https://doi.org/10.1016/J.APGEOCHEM.2023.105782
Jin B, Yan XQ, Yu Q, Van Leeuwen JH (2002) A comprehensive pilot plant system for fungal biomass protein production and wastewater reclamation. Advances in Environmental Research 6(2):179–189. https://doi.org/10.1016/S1093-0191(01)00049-1
Junaid F, Khawaja LA, Ali S (2020) Sincle Cell Protein as a Potential Meat Substitute: A Critical Review. World Journal of Pharmaceutical Research www.wjpr.net 9 2 141. https://doi.org/10.20959/wjpr20202-16686
Kam S, Kenari AA, Younesi H (2012) Production of single cell protein in stickwater by lactobacillus acidophilus and aspergillus niger. J Aquat Food Prod Technol 21(5):403–417. https://doi.org/10.1080/10498850.2011.605539
Kamal MM, Ali MR, Shishir MRI, Saifullah M, Haque MR, Mondal SC (2019) Optimization of process parameters for improved production of biomass protein from Aspergillus niger using banana peel as a substrate. Food Sci Biotechnol 28:1693–1702. https://doi.org/10.1007/s10068-019-00636-2
Kantachote D, Nunkaew T, Kantha T, Chaiprapat S (2016) Biofertilizers from Rhodopseudomonas palustris strains to enhance rice yields and reduce methane emissions. Applied Soil Ecology 100:154–161. https://doi.org/10.1016/j.apsoil.2015.12.015
Koukoumaki DI, Tsouko E, Papanikolaou S, Ioannou Z, Diamantopoulou P, Sarris D (2024) Recent advances in the production of single cell protein from renewable resources and applications. Carbon Resources Conversion 7(2):100195. https://doi.org/10.1016/j.crcon.2023.07.004
Kumar T, Jujjavarapu SE (2023a) Advances in anode configurations for a microbial fuel cell via a computational fluid dynamics electrochemistry and its experimental validation. J Chem Technol Biotechnol 98(12):3016–3030. https://doi.org/10.1002/jctb.7397
Kumar T, Jujjavarapu SE (2023b) Carbon dioxide sequestration and wastewater treatment via an innovative self-sustaining algal microbial fuel cell. J Clean Prod 415:137836. https://doi.org/10.1016/j.jclepro.2023.137836
Kumar P, Mehta N, Abubakar AA, Verma AK, Kaka U, Sharma N, Sazili AQ, Pateiro M, Kumar M, Lorenzo JM (2023) Potential Alternatives of Animal Proteins for Sustainability in the Food Sector. Food Rev Intl 39(8):5703–5728. https://doi.org/10.1080/87559129.2022.2094403
Kumar R, Raj T, Næss G, Sørensen M, Dhawan V (2024) Opportunities and challenges in single-cell protein production using lignocellulosic material. Biofuels, Bioprod Biorefin 18(1):310–321. https://doi.org/10.1002/bbb.2563
Li M, Li S (2022) Research on Optimization of Food Industry Processing Process Based on Computational Intelligence. Wirel Commun Mob Comput 2022:1–10. https://doi.org/10.1155/2022/7781369
Li Z, Lu D, Gao X (2021) Optimization of mixture proportions by statistical experimental design using response surface method - A review. Journal of Building Engineering 36:102101. https://doi.org/10.1016/j.jobe.2020.102101
Liu B, Li Y, Song J, Zhang L, Dong J, Yang Q (2014) Production of single-cell protein with two-step fermentation for treatment of potato starch processing waste. Cellulose 21(5):3637–3645. https://doi.org/10.1007/s10570-014-0400-6
Liu K, Huang S, Zhang L, Xiong Y, Wang X, Bao Y, Li D, Li J (2024) Efficient production of single cell protein from biogas slurry using screened alkali-salt-tolerant Debaryomyces hansenii. Bioresour Technol 393:130119. https://doi.org/10.1016/j.biortech.2023.130119
Magalhães CEB, Souza-Neto MS, Astolfi-Filho S, Matos ITSR (2018) Candida tropicalis able to produce yeast single cell protein using sugarcane bagasse hemicellulosic hydrolysate as carbon source. Biotechnology Research and Innovation 2(1):19–21. https://doi.org/10.1016/j.biori.2018.08.002
Mandenius C, Brundin A (2008) Bioprocess optimization using design-of-experiments methodology. Biotechnol Prog 24(6):1191–1203. https://doi.org/10.1002/btpr.67
Marius KS, Cheik ATO, Iliassou M, Mahamadi N, Ibrahim K, Nicolas O, Desire T, Alfred ST (2017) Optimization of Saccharomyces cerevisiae SKM10 single cell protein production from mango (Magnifera indica L.) waste using response surface methodology. Afr J Biotechnol 16(45):2127–2133. https://doi.org/10.5897/ajb2017.16210
Marius KS, Mahamadi N, Ibrahim K, Iliassou M, Sonagnon HSK, Yerobessor D, Wahauwouele HC, Essodolom T, Alfred ST (2018) Production of single cell protein (SCP) and essentials amino acids from Candida utilis FMJ12 by solid state fermentation using mango waste supplemented with nitrogen sources. Afr J Biotechnol 17(23):716–723. https://doi.org/10.5897/ajb2017.16361
Maruyama J (2021) genome editing technology and its application potentials in the industrial filamentous fungus aspergillus oryzae. Journal of Fungi 7(8):638. https://doi.org/10.3390/jof7080638
Mensah JKM, Twumasi P (2017) Use of pineapple waste for single cell protein (SCP) production and the effect of substrate concentration on the yield. J Food Process Eng 40(3):e12478. https://doi.org/10.1111/jfpe.12478
Mitra AC, Jawarkar M, Soni T, Kiranchand GR (2016) Implementation of Taguchi Method for Robust Suspension Design. Procedia Eng 144:77–84. https://doi.org/10.1016/j.proeng.2016.05.009
Molfetta M, Morais EG, Barreira L, Bruno GL, Porcelli F, Dugat-Bony E, Bonnarme P, Minervini F (2022) Protein Sources Alternative to Meat: State of the Art and Involvement of Fermentation. Foods 11(14):2065. https://doi.org/10.3390/foods11142065
Myers RH, Montgomery DC, Anderson-Cook CM (2016) Response surface methodology: process and product optimization using designed experiments. John Wiley & Sons
Nannyonga S, Tchuenbou-Magaia F, Goode K, Fryer P, Robbins P (2018) Growth kinetics and modelling of S. Cerevisiae (NCYC 431) during de-lignified waste banana fermentation and chemical characterization. Biochem Eng J 137:255–261. https://doi.org/10.1016/J.BEJ.2018.06.001
Nunes JJ, Aufderheide B, M. Ramjattan D, Dass R (2016) Enhanced production of single cell protein from M capsulatus (BATH) growing in mixed culture. Journal of Microbiology Biotechnology and Food Sciences 6 3 894–899. https://doi.org/10.15414/jmbfs.2016/17.6.3.894-899
Nyyssölä A, Suhonen A, Ritala A, Oksman-Caldentey K-M (2022) The role of single cell protein in cellular agriculture. Curr Opin Biotechnol 75:102686. https://doi.org/10.1016/j.copbio.2022.102686
Oliveira SD, de Araújo Padilha CE, Asevedo EA, Pimentel VC, de Araújo FR, de Macedo GR, dos Santos ES (2018) Utilization of agroindustrial residues for producing cellulases by Aspergillus fumigatus on Semi-Solid Fermentation. J Environ Chem Eng 6(1):937–944. https://doi.org/10.1016/j.jece.2017.12.038
Ortloff D, Popp J (2010) The challenges of statistical experiment design in process development. Proceedings of NSTI TechConnect World 2
Özyurt M, Deveci EÜ (2004) Conversion of agricultural and industrial wastes for single cell protein production and pollution potential reduction: a review. Fresenius Environ Bull 13(8):693–699
Packiam KAR, Ramanan RN, Ooi CW, Krishnaswamy L, Tey BT (2020) Stepwise optimization of recombinant protein production in Escherichia coli utilizing computational and experimental approaches. Appl Microbiol Biotechnol 104(8):3253–3266. https://doi.org/10.1007/s00253-020-10454-w
Patel RP, Patel MP, Suthar AM (2009) Spray drying technology: an overview. Indian J Sci Technol 2(10):44–47
Patsios SI, Dedousi A, Sossidou EΝ, Zdragas A (2020) Sustainable Animal Feed Protein through the Cultivation of Yarrowia Lipolytica on Agro-Industrial Wastes and by-Products. Sustainability 12(4):1398. https://doi.org/10.3390/su12041398
Patthawaro S, Saejung C (2019) Production of single cell protein from manure as animal feed by using photosynthetic bacteria. Microbiologyopen 8(12):e913. https://doi.org/10.1002/mbo3.913
Peng X, Yang G, Shi Y, Zhou Y, Zhang M, Li S (2020) Box-Behnken design based statistical modeling for the extraction and physicochemical properties of pectin from sunflower heads and the comparison with commercial low-methoxyl pectin. Sci Rep 10(1):3595. https://doi.org/10.1038/s41598-020-60339-1
Pietraszek J, Radek N, Goroshko A V. (2020) Challenges for the DOE methodology related to the introduction of Industry 4.0. Production Engineering Archives 26 4 190–194. https://doi.org/10.30657/pea.2020.26.33
Pogaku R, Rudravaram R, Chandel AK, Linga VR, Yim ZH (2009) The effect of de-oiled rice bran for single cell protein production using fungal cultures under solid state fermentation. International Journal of Food Engineering 5:2. https://doi.org/10.2202/1556-3758.1502
Prado-Rubio OA, Jørgensen JB, Jørgensen SB (2010) Systematic model analysis for single cell protein (SCP) production in a U-loop reactor. Computer Aided Chemical Engineering 28:319–324. https://doi.org/10.1016/S1570-7946(10)28054-9
Prakash P, Namasivayam SKR, Swetha S (2015) Design of medium components for the enhanced production of mycoprotein by Fusarium venenatum using Plackett Burman model. Res J Pharm Biol Chem Sci 6(1):1251–1255
Prastujati AU, Hilmi M, Khusna A, Sjofjan O, Natsir MH, Talitha SU (2022) Optimization of Single Cell Protein (SCP) Production using Saccharomyces Cerevisiae by Response Surface Methodology (RSM) on Cell Biomass and Protein Percentage. In: Proceedings of the International Conference on Improving Tropical Animal Production for Food Security (ITAPS 2021). Atlantis Press, pp 483–489
Procházka P, Abrham J, Cerveny J, Soukupová J, Ouma CN, Mullen KJ, Sanova P, Smutka L (2023) Algae as a source of protein in the sustainable food and gastronomy industry. Front Sustain Food Syst. https://doi.org/10.3389/fsufs.2023.1256473
Putra MD, Abasaeed AE (2018) A more generalized kinetic model for binary substrates fermentations. Process Biochem 75:31–38. https://doi.org/10.1016/j.procbio.2018.09.017
Putri D, Ulhidayati A, Musthofa IA, Wardani AK (2018) Single cell protein production of Chlorella sp. using food processing waste as a cultivation medium. In: IOP Conference Series: Earth and Environmental Science. IOP Publishing, 012052
Quinn GP, Keough MJ (2002) Experimental design and data analysis for biologists. Cambridge University Press
Book Google Scholar
Rachamontree P, Phusantisampan T, Woravutthikul N, Pornwongthong P, Sriariyanun M (2015) Selection of Pichia kudriavzevii strain for the production of single-cell protein from cassava processing waste. International Journal of Bioengineering and Life Sciences 9(5):517–521
Rafiqul IM, Jalal KCA, Alam MZ (2004) Environmental Factors for Optimisation of Spirulina Biomass in Laboratory Culture. Biotechnology 4(1):19–22. https://doi.org/10.3923/biotech.2005.19.22
Raita S, Kusnere Z, Spalvins K, Blumberga D (2022) Optimization of Yeast Cultivation Factors for Improved SCP Production. Environmental and Climate Technologies 26(1):848–861. https://doi.org/10.2478/rtuect-2022-0064
Rajoka MI, Khan SH, Jabbar MA, Awan MS, Hashmi AS (2006) Kinetics of batch single cell protein production from rice polishings with Candida utilis in continuously aerated tank reactors. Bioresour Technol 97(15):1934–1941. https://doi.org/10.1016/J.BIORTECH.2005.08.019
Rajput SD, Pandey N, Sahu K (2024) A comprehensive report on valorization of waste to single cell protein: strategies, challenges, and future prospects. Environ Sci Pollut Res. https://doi.org/10.1007/s11356-024-33004-7
Rasouli Z, Valverde-Pérez B, D’Este M, De Francisci D, Angelidaki I (2018) Nutrient recovery from industrial wastewater as single cell protein by a co-culture of green microalgae and methanotrophs. Biochem Eng J 134:129–135. https://doi.org/10.1016/j.bej.2018.03.010
Razzak SA, Lucky RA, Hossain MM, deLasa H (2022) Valorization of Microalgae Biomass to Biofuel Production: A review. Energy Nexus 7:100139. https://doi.org/10.1016/j.nexus.2022.100139
Razzaq ZU, Khan MKI, Maan AA, Rahman S, ur, (2020) Characterization of single cell protein from Saccharomyces cerevisiae for nutritional, functional and antioxidant properties. Journal of Food Measurement and Characterization 14(5):2520–2528. https://doi.org/10.1007/s11694-020-00498-x
Reihani SFS, Khosravi-Darani K (2019) Influencing factors on single-cell protein production by submerged fermentation: A review. Electron J Biotechnol 37:34–40. https://doi.org/10.1016/j.ejbt.2018.11.005
Rheem S (2023) Optimizing Food Processing through a New Approach to Response Surface Methodology. Food Sci Anim Resour 43(2):374–381. https://doi.org/10.5851/kosfa.2023.e7
Ritala A, Häkkinen ST, Toivari M, Wiebe MG (2017) Single Cell Protein—State-of-the-Art, Industrial Landscape and Patents 2001–2016. Front Microbiol 8:2009. https://doi.org/10.3389/fmicb.2017.02009
Rosma A, Ooi KI (2006) Production of Candida utilis Biomass and Intracellular Protein Content: Effect of Agitation Speed and Aeration Rate. Malays J Microbiol 2(2):15–18
Saad S, Hussien MH, Abou-ElWafa GS, Aldesuquy HS, Eltanahy E (2023) Filter cake extract from the beet sugar industry as an economic growth medium for the production of Spirulina platensis as a microbial cell factory for protein. Microb Cell Fact 22(1):136. https://doi.org/10.1186/s12934-023-02146-7
Sharif M, Zafar MH, Aqib AI, Saeed M, Farag MR, Alagawany M (2021) Single cell protein: Sources, mechanism of production, nutritional value and its uses in aquaculture nutrition. Aquaculture 531:735885. https://doi.org/10.1016/J.AQUACULTURE.2020.735885
Sheth U, Patel S (2023) Production, Economics, and Marketing of Yeast Single Cell Protein. In: Food Microbiology Based Entrepreneurship: Making Money From Microbes. Springer, 133–152
Singh N, Rai V, Tripathi CKM (2012) Production and optimization of oxytetracycline by a new isolate Streptomyces rimosus using response surface methodology. Med Chem Res 21(10):3140–3145. https://doi.org/10.1007/s00044-011-9845-4
Singh V, Haque S, Niwas R, Srivastava A, Pasupuleti M, Tripathi CKM (2017) Strategies for Fermentation Medium Optimization: An In-Depth Review. Front Microbiol. https://doi.org/10.3389/fmicb.2016.02087
Song H-S, Cannon W, Beliaev A, Konopka A (2014) Mathematical Modeling of Microbial Community Dynamics: A Methodological Review. Processes 2(4):711–752. https://doi.org/10.3390/pr2040711
Souza Filho PF, Nair RB, Andersson D, Lennartsson PR, Taherzadeh MJ (2018) Vegan-mycoprotein concentrate from pea-processing industry byproduct using edible filamentous fungi. Fungal Biol Biotechnol 5(1):5. https://doi.org/10.1186/s40694-018-0050-9
Spalvins K, Ivanovs K, Blumberga D (2018) Single cell protein production from waste biomass: Review of various agricultural by-products. Agronomy Research 16 1493–1508. https://doi.org/10.15159/AR.18.129
Spanoghe J, Vermeir P, Vlaeminck SE (2021) Microbial food from light, carbon dioxide and hydrogen gas: Kinetic, stoichiometric and nutritional potential of three purple bacteria. Bioresour Technol 337:125364. https://doi.org/10.1016/j.biortech.2021.125364
Sun W, Zhang Z, Li X, Lu X, Liu G, Qin Y, Zhao J, Qu Y (2024) Production of single cell protein from brewer’s spent grain through enzymatic saccharification and fermentation enhanced by ammoniation pretreatment. Bioresour Technol 394:130242. https://doi.org/10.1016/j.biortech.2023.130242
Sundaram T, Rajendran S, Gnanasekaran L, Rachmadona N, Jiang J-J, Khoo KS, Show PL (2023) Bioengineering strategies of microalgae biomass for biofuel production: recent advancement and insight. Bioengineered 14 1 https://doi.org/10.1080/21655979.2023.2252228
Swalley SE, Fulghum JR, Chambers SP (2006) Screening factors effecting a response in soluble protein expression: Formalized approach using design of experiments. Anal Biochem 351(1):122–127. https://doi.org/10.1016/j.ab.2005.11.046
Taran M, Asadi N (2014) A novel approach for environmentally friendly production of single cell protein from petrochemical wastewater using a halophilic microorganism in different conditions. Pet Sci Technol 32(5):625–630. https://doi.org/10.1080/10916466.2011.596888
Taran M, Bakhtiyari S (2012) Production of single cell protein by a halophilic micro-organism using glucose as carbon source: optimization of process variables in extreme conditions by Taguchi experimental design. Global Adv Res J Microbiol 1(3):41–46
Taran M, Bakhtiyari S (2013) Optimization of single-cell protein production from textile effluent at extreme conditions. Toxicol Environ Chem 95(1):110–117. https://doi.org/10.1080/02772248.2012.745861
Thiry M, Cingolani D (2002) Optimizing scale-up fermentation processes. Trends Biotechnol 20(3):103–105. https://doi.org/10.1016/S0167-7799(02)01913-3
Thiviya P, Gamage A, Kapilan R, Merah O, Madhujith T (2022a) Production of Single-Cell Protein from Fruit Peel Wastes Using Palmyrah Toddy Yeast. Fermentation 8(8):355. https://doi.org/10.3390/fermentation8080355
Thiviya P, Gamage A, Kapilan R, Merah O, Madhujith T (2022b) Single Cell Protein Production Using Different Fruit Waste: A Review. Separations 9(7):178. https://doi.org/10.3390/separations9070178
Trajkovska Petkoska A, Daniloski D, D’Cunha NM, Naumovski N, Broach AT (2021) Edible packaging: Sustainable solutions and novel trends in food packaging. Food Res Int 140:109981. https://doi.org/10.1016/j.foodres.2020.109981
Türker M, Selimoğlu SM, Taşpınar-Demir H (2022) Waste (water) to feed protein: Effluent characteristics, protein recovery, and single-cell protein production from food industry waste streams. Clean Energy and Resource Recovery :201–244
Ugwuanyi JO (2008) Yield and protein quality of thermophilic Bacillus spp. biomass related to thermophilic aerobic digestion of agricultural wastes for animal feed supplementation. Bioresour Technol 99 8 3279–3290
Umesh M, Thazeem B, Preethi K (2019) Valorization of Pineapple Peels through Single Cell Protein Production Using Saccharomyces cerevisiae NCDC 364. Research Article APPLIED FOOD BIOTECHNOLOGY 6 4 255–263. https://doi.org/10.22037/afb.v6i4.25906
Verstraete W, Clauwaert P, Vlaeminck SE (2016) Used water and nutrients: Recovery perspectives in a ‘panta rhei’ context. Bioresour Technol 215:199–208. https://doi.org/10.1016/j.biortech.2016.04.094
Wu M, Huusom JK, Gernaey KV, Krühne U (2016) Modelling and simulation of a U-loop Reactor for Single Cell Protein Production. In: Kravanja Z, Bogataj M (eds) Computer Aided Chemical Engineering. Elsevier, pp 1287–1292
Yadav JSS, Bezawada J, Ajila CM, Yan S, Tyagi RD, Surampalli RY (2014) Mixed culture of Kluyveromyces marxianus and Candida krusei for single-cell protein production and organic load removal from whey. Bioresour Technol 164:119–127. https://doi.org/10.1016/j.biortech.2014.04.069
Yan J, Han B, Gui X, Wang G, Xu L, Yan Y, Madzak C, Pan D, Wang Y, Zha G, Jiao L (2018) Engineering Yarrowia lipolytica to Simultaneously Produce Lipase and Single Cell Protein from Agro-industrial Wastes for Feed. Sci Rep 8:1. https://doi.org/10.1038/s41598-018-19238-9
Yang R, Chen Z, Hu P, Zhang S, Luo G (2022a) Two-stage fermentation enhanced single-cell protein production by Yarrowia lipolytica from food waste. Bioresour Technol 361:127677. https://doi.org/10.1016/j.biortech.2022.127677
Yang T, Lin S-Y, Hung Y-H, Hong C-C (2022b) A Study on the Optimization of In-Process Inspection Procedure for Active Pharmaceutical Ingredients Manufacturing Process. Sustainability 14(6):3706. https://doi.org/10.3390/su14063706
Zhang L, Zhou P, Chen YC, Cao Q, Liu XF, Li D (2021) The production of single cell protein from biogas slurry with high ammonia-nitrogen content by screened Nectaromyces rattus. Poult Sci 100(9):101334. https://doi.org/10.1016/j.psj.2021.101334
Zhou YM, Chen YP, Guo JS, Shen Y, Yan P, Yang JX (2019) Recycling of orange waste for single cell protein production and the synergistic and antagonistic effects on production quality. J Clean Prod 213:384–392. https://doi.org/10.1016/j.jclepro.2018.12.168
Zhou P, Zhang L, Ding H, Gao X, Chen Y, Li D (2022) Optimization of culture conditions of screened Galactomyces candidum for the production of single cell protein from biogas slurry. Electron J Biotechnol 55:47–54. https://doi.org/10.1016/j.ejbt.2021.11.006
Download references
Acknowledgements
The authors are thankful to the School of Studies in Biotechnology, Pt. Ravishankar Shukla University, Raipur, Chhattisgarh, India, for providing the necessary facilities to prepare the manuscript and for permission to publish it.
The authors also are thankful to the Department of Biotechnology, Ministry of Science & Technology, Government of India, for awarding research fellowship (No. DBTHRDPMU/JRF/BET-22/I/2022–23/140, dated 26.07.2022) to Sharda Devi Rajput under the DBT-JRF programme.
Author information
Authors and affiliations.
School of Studies in Biotechnology, Pt. Ravishankar Shukla University, Raipur, 492 010, India
Sharda Devi Rajput, Neha Pandey & S. Keshavkant
You can also search for this author in PubMed Google Scholar
Contributions
The First author Sharda Devi Rajput has contributed- Conceptualization, Roles/Writing—original draft; Writing—review & editing. The Second author Neha Pandey contributed- Writing—review & editing. The third author S. Keshavkant (Corresponding author) contributed- Supervision, Writing—review & editing, and Software.
Corresponding author
Correspondence to S. Keshavkant .
Ethics declarations
Conflict of interest.
The authors declare that they have no known competing financial interests or personal relationships that could have appeared to influence the work reported in this paper.
Additional information
Publisher's note.
Springer Nature remains neutral with regard to jurisdictional claims in published maps and institutional affiliations.
Rights and permissions
Springer Nature or its licensor (e.g. a society or other partner) holds exclusive rights to this article under a publishing agreement with the author(s) or other rightsholder(s); author self-archiving of the accepted manuscript version of this article is solely governed by the terms of such publishing agreement and applicable law.
Reprints and permissions
About this article
Rajput, S.D., Pandey, N. & Keshavkant, S. Optimization strategies for enhanced production of single cell protein: recent advances and perspectives. Rev Environ Sci Biotechnol (2024). https://doi.org/10.1007/s11157-024-09706-2
Download citation
Received : 21 June 2024
Accepted : 05 September 2024
Published : 19 September 2024
DOI : https://doi.org/10.1007/s11157-024-09706-2
Share this article
Anyone you share the following link with will be able to read this content:
Sorry, a shareable link is not currently available for this article.
Provided by the Springer Nature SharedIt content-sharing initiative
- Microbial protein;
- Design of experiment (DoE)
- Response surface methodology (RSM)
- Factorial design
- Central composite design (CCD)
- Box-behnken design (BBD)
- Find a journal
- Publish with us
- Track your research

IMAGES
VIDEO
COMMENTS
Research design was classified into one of the types discussed later in the section titled Predominant Single-Case Experimental Designs on the basis of the authors' stated design type. Secondary research designs were then coded when applicable (i.e., mixed designs). Distinctions between primary and secondary research designs were made based ...
Single-case experimental designs (SCEDs) involve repeat measurements of the dependent variable under different experimental conditions within a single case, for example, a patient or a classroom 1 ...
Abstract. Single-case experimental designs (SCEDs) represent a family of research designs that use experimental methods to study the effects of treatments on outcomes. The fundamental unit of analysis is the single case—which can be an individual, clinic, or community—ideally with replications of effects within and/or between cases.
Single-case experimental designs are a family of experimental designs that are characterized by researcher manipulation of an independent variable and repeated measurement of a dependent variable before (i.e., baseline) and after (i.e., intervention phase) introducing the independent variable. In single-case experimental designs a case is the ...
This article highlights core features of single-case experimental designs, how key and ancillary features of the designs have evolved, the special strengths of the designs, and challenges that have impeded their integration in many areas where their contributions are sorely needed. The article ends by placing the methodological approach in the ...
2. Single-Case Experimental Designs: Definition and Overview of Design Options. Contemporary textbooks on SCEDs follow a long tradition. An early and fierce proponent of SCEDs was B.F. Skinner [], proclaiming in his 1956 seminal paper on the scientific method that "we are within reach of a science of the individual.This will be achieved, not by resorting to some special theory of knowledge ...
Single-case designs (also called single-case experimental designs) are system of research design strategies that can provide strong evidence of intervention effectiveness by using repeated measurement to establish each participant (or case) as his or her own control. The flexibility of the designs, and the focus on the individual as the unit of ...
Single-case experimental designs are rapidly growing in popularity. This popularity needs to be accompanied by transparent and well-justified methodological and statistical decisions. Appropriate ...
Single-case experimental designs have been used extensively with a variety of clinical populations and in a wide range of in-patient, outpatient, school, business, and community settings (Kazdin, 2011; Kratochwill & Levin, 2014). "Single-case" can mislead because the designs are not necessarily restricted to the single case, that is, one ...
Recommendations for Clinical Practice. When rigorously designed, single-case studies can be particularly useful experimental designs in a variety of situations, even when researcher resources are limited, studied conditions have low incidences, or when examining effects of novel or expensive interventions.
Single-case experimental designs are used by scientist-practitioners who employ strategies from the experimental analysis of behavior (Baer, Wolf, & Risley, 1968). These designs are those in which the effects of an intervention are evaluated with one, or few, subjects. There is an assortment of single-case designs including, but not limited to ...
Single-case experimental designs (SCED) are experimental designs aiming at testing the effect of an intervention using a small number of patients (typically one to three), using repeated measurements, sequential (± randomized) introduction of an intervention and method-specific data analysis, including visual analysis and specific statistics.The aim of this paper is to familiarise ...
Abstract. Single-case designs refer to a methodological approach that can be used to investigate the effectiveness of treatment with the individual client. The designs permit scientifically valid inferences to be drawn about the effects of treatment and hence offer advantages over alternative strategies such as the uncontrolled case study or ...
This article highlights core features of single-case experimental designs, how key and ancillary features of the designs have evolved, the special strengths of the designs, and challenges that have impeded their integration in many areas where their contributions are sorely needed. The article ends by placing the methodological approach in the ...
Single-subject experimental designs - also referred to as within-subject or single case experimental designs - are among the most prevalent designs used in CSD treatment research. These designs provide a framework for a quantitative, scientifically rigorous approach where each participant provides his or her own experimental control.
This article highlights core features of single‐case experimental designs, how key and ancillary features of the designs have evolved, the special strengths of the designs, and challenges that have impeded their integration in many areas where their contributions are sorely needed. The article ends by placing the methodological approach in ...
Research methods routinely taught in psychology and the sciences more generally focus on null hypothesis significance testing. This chapter provides an overview of single-case experimental designs. The unique feature of single-case research designs is the capacity to conduct experimental investigations with a single case. Single-case designs can evaluate the effects of interventions with large ...
Single-case experimental designs provide a flexible methodology that is readily adaptable to the participant's clinical characteristics. Rigorously designed SCEDs with high internal validity can establish cause-effect relationships. A series of such studies can incorporate strategies to also enhance external validity and furnish evidence ...
The book briefly traces the history of single-case experimental designs (SCEDs); outlines important considerations in understanding and planning a scientifically rigorous single-case study, including internal and external validity; describes prototypical single-case designs (withdrawal-reversal designs and the medical N-of-1 trial, multiple ...
Single-Case Experimental Designs (SCEDs) are experimental designs used to repeatedly measure one or multiple participants across at least two conditions: a condition without intervention (i.e., baseline condition) and a condition with intervention (i.e., intervention or treatment condition). Intervention effectiveness can be evaluated by ...
We outline why single case experimental designs (SCEDs) are a rigorous and effective means of addressing under-representation in P-SEM research. CP is an eligible underlying health condition for 17 of the 22 Paralympic sports. It results from a non-progressive brain lesion and is defined as a heterogeneous group of permanent disorders affecting ...
Single-case experimental designs (SCED) are experimental designs aiming at testing the effect of an intervention using a small number of patients (typically one to three), using repeated measurements, sequential (±randomized) introduction of an intervention and method-specific data analysis, including visual analysis and specific statistics. ...
The project encompasses two PhD projects: one focused on Bayesian evaluation of single case experimental designs (that is, the methodology that will be used for therapy effect evaluation) and the other focused on treatment development and evaluation. The current vacancy applies to Bayesian evaluation of SCEDs.
Single-case Experimental Designs in Clinical Settings. W.C. Follette, in International Encyclopedia of the Social & Behavioral Sciences, 2001. 2 Characteristics of Single-case Design. Single-case designs study intensively the process of change by taking many measures on the same individual subject over a period of time. The degree of control in ...
The NWO-funded project "Accelerating the development of effective psychological treatments for traumatised refugees using Bayesian methodology" is looking for a PhD candidate focused on Bayesian evaluation of single case experimental designs. Your job. In single case experimental designs (SCEDs), as applied in therapy effect evaluation, 10 ...
The principal objective of applying experimental design in analytical processes is to achieve optimal and valid results with a minimum investment of time and resources. In an experimental design, one or more predetermined factors are deliberately manipulated to determine their effects on the experimental outcome (Ferreira et al. 2007).