- Open access
- Published: 10 October 2017

Network science approach to modelling the topology and robustness of supply chain networks: a review and perspective
- Supun Perera 1 ,
- Michael G.H. Bell 1 &
- Michiel C.J. Bliemer 1
Applied Network Science volume 2 , Article number: 33 ( 2017 ) Cite this article
10k Accesses
67 Citations
2 Altmetric
Metrics details
Due to the increasingly complex and interconnected nature of global supply chain networks (SCNs), a recent strand of research has applied network science methods to model SCN growth and subsequently analyse various topological features, such as robustness. This paper provides: (1) a comprehensive review of the methodologies adopted in literature for modelling the topology and robustness of SCNs; (2) a summary of topological features of the real world SCNs, as reported in various data driven studies; and (3) a discussion on the limitations of existing network growth models to realistically represent the observed topological characteristics of SCNs. Finally, a novel perspective is proposed to mimic the SCN topologies reported in empirical studies, through fitness based generative network models.
Introduction
Global supply chain networks (SCNs) play a vital role in fuelling international trade, freight transport by all modes, and economic growth. Due to the interconnectedness of global businesses, which are no longer isolated by industry or geography, disruptions to infrastructure networks caused by natural disasters, acts of war and terrorism, and even labour disputes are becoming increasingly complex in nature and global in consequences (Manuj and Mentzer, 2008 ). Disruptions ripple through global SCNs, potentially magnifying the original damage. Even relatively minor disturbances, such as labour disputes, ground traffic congestion or air traffic delays can result in severe disruptions to local and international trade. Therefore, this ‘fragility of interdependence’ creates new risks to global and local economies (Vespignani, 2010 ).
At the local level, disturbances to SCNs can have major social and economic ramifications. For instance, during the 2011 Queensland floods in Australia, the key transportation routes were shut down, preventing supermarkets from restocking and leading to critical food shortages (Bartos, 2012 ). However, at the global level, these consequences can be magnified, resulting in more significant and longer lasting damage. A recent example of such a global SCN disruption is the 2011 Tohoku earthquake and ensuing tsunami in the northeast coast of Japan. Alongside the appalling humanitarian impact, this tsunami caused destruction of critical infrastructure in Japan, resulting in a domino effect, which propagated through global SCNs, with significant global economic consequences. It is reported that for several weeks following the disaster, Toyota in North America experienced shortages of over 150 parts, leading to curtailed operations at only 30% of capacity (Canis, 2011 ). Similar impacts were observed following the September 11th terrorist attacks on the United States in 2001, where movement of electronic and automotive parts were disrupted due to the shutdown of air and truck transportation networks (Sheffi, 2001 ). These high impact low probability disruptions have affected a large number of economic variables such as industrial production, international trade and logistics operations, thus revealing vulnerabilities in the global SCNs, which are traditionally left unaddressed (Tett, 2011 ). Therefore, the design of supply chains that can maintain their function in the face of perturbations, both expected and unexpected, is a key goal of contemporary supply chain management (Lee, 2004 ).
Until recently, the primary focus of supply chain management was on increasing efficiency and reliability by means of globalization, specialization and lean supply chain procedures. Although, these practices enable cost savings in daily operations, they have also made the SCNs more vulnerable to disruptions (World Economic Forum, 2013 ). Under a low probability high impact disruption, lean supply chains would shut down in a matter of hours, with global implications. Supply concentration and IT reliance make the supply chains vulnerable to targeted attacks. This is particularly evident in the SCNs with low levels of ‘buffer’ inventory (Jüttner et al., 2003 ).
A recent strand of publications, by both academic and industry communities, has revealed the importance of understanding and quantifying robustness in global SCNs. Increasing focus has been given to modelling SCNs as complex adaptive systems, in recent years, using network science methods to examine the robustness of various network topologies (Choi et al., 2001 ; Surana et al., 2005 ; Brintrup et al., 2016 ).
The aim of this paper is to present a critical assessment of the research published, mainly in the last decade, in the field of modelling the topology and robustness of SCNs using network science concepts. A novel perspective is then presented in relation to the way forward. The subsequent sections of this paper are structured as follows; From complex systems theory to network science section discusses the complex system nature of modern SCNs and introduces key network science concepts in the context of SCNs; Network science approach to modelling the topology and robustness of SCNs section presents the network science approach to modelling the topology and robustness of SCNs; Discussion of literature – Limitations and improved methodological directions section presents a discussion, including comparisons, critiques and potential methodological improvements, of the research reviewed, and Conclusions and future directions section provides conclusions and outlines possible directions for future research.
From complex systems theory to network science
Complex systems theory.
Complex systems theory is a field of science that is used to investigate how the individual components and their relationships give rise to the collective behaviour of a given system (Ladyman et al., 2013 ). In essence, complex systems possess collective properties that cannot easily be derived from their individual constituents. For example, social systems which comprise relationships between individuals, the nervous system which functions through individual neurones and connections, and life on Earth itself, can all be regarded as complex systems (Kasthurirathna, 2015 ).
Although complex systems do not have a formal definition, the following three key features broadly characterise such systems (Bar-Yam, 2002 );
Emergence : Macro level properties, which dynamically originate from the activities and behaviours of the individual agents of the system, cannot be easily explained at the agent level alone (Kaisler and Madey, 2009 ). Therefore, emergence is governed by micro level interactions that are ‘bottom-up’ rather than ‘top-down’ rules.
Interdependence : Individual components depend on each other to varying degrees (Buckley, 2008 ).
Self-organisation : This is the attribute that is most commonly shared by all complex systems, where large scale organisation manifests itself spontaneously without any central control, based on local feedback mechanisms that either amplify or dampen disturbances (Mina et al., 2006 ).
Complex system characteristics of modern supply chain networks
Traditionally, a focal firm is assumed to be responsible for shaping the structure of a given SCN by selecting different suppliers for various purposes, such as reduced cost, increased flexibility/redundancy, and so on. However, the ability of a single firm to shape its supply chain seems to significantly diminish as SCNs become more global and complex in nature. Therefore, the topological structure of a SCN can increasingly be considered as emergent. As such, in a global and a complex business landscape, an individual firm may benefit more from positioning itself within the SCN rather than attempting to shape the SCN’s overall topology (Xuan et al., 2011 ).
Choi et al. ( 2001 ) note the complex adaptive system nature of large scale SCNs, where an interconnected network of multiple entities exhibit adaptiveness in response to changes in both the environment and the system itself. System behaviour emerges as a result of the large number of activities made in parallel by interacting entities (Pathak et al., 2007 ). Therefore, from the point of view of a single firm, the overall SCN is a self-organising system, which consists of various entities engaging in localised decision-making. Given this distributed nature of decision making, the configuration of the final SCN is beyond the realm of control of one organisation. Indeed, individual firms may pursue their own goals with the SCN emerging over time (Choi and Hartley, 1996 ; Choi et al., 2001 ).
Network modelling of supply chains
Traditionally, supply chains have been modelled as multi agent (or agent based) systems, in order to represent explicit communications between the various entities involved (Gjerdrum et al., 2001 ; Julka et al., 2002 ; Nair and Vidal, 2011 ). The earliest example of such a model is Forrester’s supply chain model (Forrester, 1961 ; Forrester, 1973 ), which comprised four types of agents, representing various organisations involved in a supply chain (namely; retailers, wholesalers, distributors and manufacturers), interacting with each other. Such agent-based models (ABMs) provide autonomy to each entity involved and define behaviours in terms of observables accessible to each agent and its goals, norms and decision rules (Parunak et al., 1998 ; Rahmandad and Sterman, 2008 ). ABMs are a form of logical deduction, since, given a set of basic rules and initial conditions, the emergent outcomes are embedded in the rules, however surprising they may be (Epstein and Axtell, 1996 ; Berryman and Angus, 2010 ).
ABMs are considered to be micro-models, since they facilitate system level inference from explicitly programmed, micro-level rules in simulated agent populations over time and space in a given environment. While such a bottom-up approach maybe suitable for relatively small systems, the exponential increase in the number of connected entities that comprise modern global SCNs favour a top-down approach to system modelling (Pruteanu, 2013 ).
In this regard, the macro perspective offered by network models are particularly valuable. A recent surge in interest in the area of networks has paved the way for what is now known as ‘network science’. Starting from the mathematical field of graph theory (Bondy and Murty, 1976 ; West, 2001 ), network science has now matured into a separate field, borrowing concepts from other domains such as statistical mechanics (Albert and Barabasi, 2002 ; Newman et al., 2011 ).
Network models focus on how topological properties affect various system properties. Such models typically do not have an environment or coevolution of the environment with the system. Rather, they consist of an ensemble of nodes that behave coherently. This top-down approach considers the network as a single entity and in some models, the individual nodes may exchange information and update the state of the system based on global specifications, which makes the system less prone to unpredictable emergent behaviour (Pruteanu, 2013 ).
Basic network models
The series of papers published by Erdȍs and Rényi on random graphs, between 1950 and 1960, sparked initial interest in network science. However, since the introduction of small-world networks by Watts and Strogatz in 1998 , interest in the field of network science has surged, as evident in literature.
The following networks are now widely regarded as benchmarks;
Erdȍs-Rényi (ER) Random graphs:
Nodes are randomly connected to each other.
Modelled using the Erdös-Rényi model (Erdȍs and Rényi, 1959 ).
Small-world networks:
Most nodes are not neighbours of one another, but can be reached from every other node by a small number of steps.
Modelled using the Watts–Strogatz model (Watts and Strogatz, 1998 ).
Scale-free networks:
The degree distribution follows power-law, at least asymptotically.
Modelled using the Barabasi-Albert (BA) model (Barabási and Albert, 1999 ).
The key characteristics of the above mentioned network topologies are presented in Fig. 1 .
Comparison of random, small-world and scale free networks. Topological structure of benchmark network models. Random and Small-world network topologies do not include hub nodes. In contrast, scale-free topologies are characterised by the presence of small number of highly connected hub nodes and a high number of feebly connected nodes. Presence of distinct hubs in scale-free networks make them more vulnerable to targeted attacks, compared to random and small-world networks
Modelling network topology
A wide range of network models are available in network science literature. They can be broadly categorised into two distinct classes as follows;
Generative models – the aim of these models is to generate a snapshot of a topology. Among generative models, some are static (time independent topologies) while some use growth (and other mechanisms). Furthermore, some generative models include predefined global properties (such as degree distribution, hierarchy and modularity) while others predefine a local property (such as the attachment probability).
Evolving models – the aim of these models is to capture the microscopic mechanisms underlying the temporal evolution of a network topology. These models include growth and in some instances may include node deletion and link rewiring. For a comprehensive summary of mechanisms underlying various evolving network models, readers are referred to Albert and Barabasi ( 2002 ).
Figure 2 outlines the different perspectives offered by generative and evolving network models.
Modelling Perspectives obtained from Generative and Evolving Network Models
Based on the above classification, both ER random and small-world network models are static generative models as they imply a fixed number of nodes where links are placed between nodes using some random algorithm. These models are therefore less widely used to model dynamical open systems, such as SCNs. However, the ER random model is generally used by researchers as a null model to test whether a real network property is statistically significant or simply attributable to random connectivity (Kito et al., 2014 ).
It is noted that the result of any static generative model can also be obtained by an evolving model (for example, an ER random network can be conveniently generated using an evolving model with a specified growth process). In fact, any evolving model can be used for generative purposes. In this regard, the BA model, which is generally considered as an evolving network model, can also be used for generative purposes, depending on the study requirement.
An evolving network growth model governs the time evolution of networks by specifying the way in which the new nodes connect with the existing nodes in the network (Zhao et al., 2011a ). This process is referred to as ‘attachment’ and various network growth models comprise various ‘attachment rules’, which subsequently generate networks with distinct topologies as they evolve. For example, the mechanism underlying generation of scale free networks has been successfully captured by the growth (in terms of nodes) and preferential attachment mechanism presented in the BA model (Barabási and Albert, 1999 ). Under preferential attachment, the probability p i that a new node makes a connection to an existing node i with degree k i is given by:
where N is the set of nodes to which the new node could connect.
The BA model represents a ‘rich get richer’ process and the resulting scale-free network topology can be used to model many real world networks, such as the World Wide Web, power grids, metabolic networks and social networks (Surana et al., 2005 ). This concept explains the existence of ‘hubs’ (a few nodes with a large number of connections), which is a defining feature of scale-free networks.
The degree distribution P k of a scale free network is approximated with power-law as follows;
where k is the degree of the node and γ is the power-law exponent.
Many network properties depend on the value of the power-law exponent, γ (Barabasi, 2014 ). Therefore, it is important to accurately estimate the power-law exponent of the degree distribution of a given network topology, as this enables us to compare network topologies on a continuous spectrum. Newman ( 2005 ) presents a reliable methodology accurately estimating the power-law exponent of a given degree distribution, which involves plotting the complementary cumulative distribution function. This method does not require data binning and as a result eliminates the plateau observed in linear binning approach for high degree regime by extending the scaling region. Interested readers are referred to Clauset et al. ( 2009 ), for a comprehensive review of power-law distributions in empirical data.
Fitness based network models
In the BA model, it is assumed that a node’s growth rate (in terms of new link acquisition) is determined solely by its degree. Accordingly, it predicts that the oldest node always has the most links – this concept is often referred to as the first mover advantage in the economics literature. However, this approach does not take into consideration the intrinsic characteristics of the nodes which may influence the rate at which they acquire new links. For instance, in many real world networks such as Hollywood actor networks and global business networks, some nodes despite being latecomers, acquire links within a short timeframe whereas others are present within the network from early on but fail to acquire high numbers of links (Barabasi, 2014 ). As such, modelling SCN growth based on a growth model which views new link acquisitions from a purely topological perspective may not be suitable.
Therefore, rather than relying entirely on the node degree, the attachment probability and subsequent network growth should rely on a more basic factor, referred to as node ‘fitness’ (Caldarelli et al., 2002 ; Ghadge et al., 2010 ; Smolyarenko, 2014 ). The concept of ‘fitness’ can be thought of as the amalgamation of all the attributes of a given node that contribute to its propensity to attract links, which could also include the node degree.
In order to overcome the limitations mentioned above, a model was proposed by Bianconi and Barabasi ( 2001 ). This model is referred to as the Bianconi-Barabasi Model (hereinafter referred to as the BB model) and has the following characteristics;
Growth – At each time step, a new node j with m links and fitness ϕ j is added to the network. In generating an ensemble of networks, ϕ j is sampled from a fitness distribution. Once assigned, the fitness of a node remains constant.
Preferential Attachment – the probability of a new node connecting to node i is proportional to the product of node i ’s degree k i and its fitness ϕ i ;
As can be seen from the above formulation, between two nodes i and j with the same fitness ( ϕ i = ϕ j ), the one with the higher degree will have the higher probability of selection. Conversely, between two nodes i and j with the same degree ( k i = k j ) the node with the higher fitness will be selected with a higher probability, thus indicating that even a relatively new node, with only a few links, can acquire more new links rapidly, if it has a higher level of fitness. As such, consideration is given to both fitness and the degree of the nodes in the above growth model.
More recently, Ghadge et al. ( 2010 ) proposed a purely fitness based network growth model, which accounts for the various factors that contribute to the likelihood of a new node being attracted to an existing node within a network. Such fitness based attachment models can indeed be categorised as generative models. This type of models offers greater flexibility owing to their ability to reproduce network topologies with fixed global properties.
In the model proposed by Ghadge et al. ( 2010 ), the fitness ϕ i , which represents the propensity of node i to attract links, is formed from the product of relevant attributes { φ i 1 , …, φ iL };
Where each attribute, φi is represented as a real non-negative value. Subsequently, it is assumed that the number of attributes affecting a node’s attractiveness is sufficiently large and are statistically independent. Therefore, by a version of the Central Limit Theorem, the overall fitness ϕ i will tend to be lognormally distributed, regardless of the type of distribution of the individual factors (Nguyen and Tran, 2012 ). In a SCN context, the attributes, which contribute to fitness, could include cost, service or product quality, reliability, and so on. Finally, the probability of connecting a new node j to an existing node i is taken to be proportional to its fitness ϕ i , as follows;
The above attachment rule, named the ‘Lognormal Fitness Attachment’ (LNFA), differs from the BA model in that node fitness replaces node degree (Nguyen and Tran, 2012 ). Therefore, in LNFA, a new node which has a large fitness, despite being in the network for a short period of time, can make itself a preferential choice for new nodes entering the network.
Recent work by Bell et al. ( 2017 ) have investigated the evolutionary mechanisms that would give rise to a fitness-based attachment process. In particular, it is proven by analytical and numerical methods that in homogeneous networks, the minimisation of maximum exposure to unfitness by each node, leads to attachment probabilities that are proportional to fitness. This result is then extended to heterogeneous networks, with strictly tiered SCNs being used as examples.
Generating null models using configuration model
Similar to the LNFA model, the configuration model belongs to the wider class of network generative models. A generative model allows us to choose parameters and draw a single instance of a network. Since a single generative model can generate many instances of networks, the model itself corresponds to an ensemble of networks.
The configuration model is commonly used to generate networks with pre-defined degree sequences. It is particularly useful for generation of null models for the purposes of hypothesis testing. Comparison of properties of an empirical network with the properties of an ensemble of networks generated by the configuration model allows one to identify if the properties observed in the empirical network are unique and meaningful or whether they are common to all networks with that degree sequence (Fosdick et al., 2016 ). When data is available for an empirical network, a technique termed degree preserving randomisation (DPR) is often used in literature to generate random networks which correspond to the configuration model. DPR involves rewiring the original network, to generate an ensemble of null models, while preserving the degree vector (Maslov and Sneppen, 2002 ). At each time step, the DRP process randomly picks two connected node pairs and switch their link targets. This switching is repeatedly applied to the entire network until each link is rewired at least once. The resulting network represents a null model where each node still has the same degree, yet the paths through the network have been randomised.
For example, Becker et al. ( 2014 ) have constructed a manufacturing system network model from real world data (where nodes represent separate work stations and links represent material flows between work stations). By applying the DPR process to generate an ensemble of networks with the same degree distribution, the authors observe that nodes (work stations) with a particularly high betweenness centrality are over-represented in the manufacturing system studied. They concludes that the manufacturing system topology is therefore non-random and favours the existence of a few highly connected work stations.
Network science approach to modelling the topology and robustness of SCNs
So far, the published research in the area of modelling SCNs as complex networks have demonstrated that a network perspective can indeed be used to successfully represent a supply chain as nodes and links (Thadakamalla et al., 2004 ; Xuan et al., 2011 ; Zhao et al., 2011a ; Zhao et al., 2011b ; Wen and Guo, 2012 ; Li et al., 2013 ; Li, 2014 ; Mari et al., 2015 and Kim et al., 2015 ). A typical SCN model consists of nodes, which represent individual firms (such as suppliers, manufacturers, distributers and retailers), and links, which represent interactions between nodes (such as exchange of information, transportation of material, and financial transactions). Such abstractions can be beneficial in identifying the properties of various types of SCN, as representing too many details could be detrimental to identifying the network properties (Shen et al., 2006 ). On the other hand, important node or link information could be lost. The level of detail to be represented by a given complex network model is an important decision to be made by the network scientist (Kasthurirathna, 2015 ).
Given the ultimate goal of obtaining generalizable results for real world SCNs, the theoretical research in this area should be well informed by empirical studies. In particular, empirical studies should be used to establish the key characteristics that need to be represented in the network topologies being generated by a given growth mechanism. Figure 3 illustrates the general methodological framework of research on topological modelling and robustness analysis of SCNs.
General methodological framework of research on topology and robustness of SCNs
Modelling SCN topologies through growth models
In order to characterise the dynamical processes on complex SCNs, the first step is to construct realistic network growth models. Such models can be used to generate an ensemble of networks with the required topological properties, from which insights can be gained into the relationship between the topology and the dynamics of complex networks (Bianconi, 2016 ).
In the context of supply chains, the concept of growth describes how newcomer firms join existing firms in a SCN. As new entrants join the SCN, trading partners are assigned from within the network. In the above regard, the BA model, despite its simplicity and elegance, includes a number of known limitations, as listed in Table 1 against their respective implications for SCNs.
Indeed, firm partner selection is, in reality, a multi-objective problem and involves numerous factors, such as price, performance, quality, goodwill, transport cost (Jain et al., 2009 ; Li et al., 2013 ). However, it is not practical to consider all these factors, so researchers have adopted simple yet intuitive approaches which extend the basic BA model concept. These include specifying selection (attachment) rules based on basic topological properties, such as the node degree (i.e. the connectedness of a given firm), in conjunction with other parameters (such as the number of links entering the system with each type of new node, the rewiring probability, and the topological distance between source and target nodes). Examples of such customised attachment rules are summarised in Table 2 .
Each of the aforementioned attachment rules, over time, generate networks with distinct topologies. It is evident, that when constructing network topologies representative of SCNs, the attachment preference is generally governed by three factors;
The type of node entering the system (which determines the number of links that enter the system with addition of new nodes and to which existing nodes these links will be connected).
Type and degree of existing nodes (preference is given to existing high degree nodes over the low degree nodes, representing the market power and visibility of highly connected firms).
Type and topological or geographical distance of existing nodes (preference is given to closer nodes than farther away ones, in terms of either the topological or the geographical distance, representing the ‘relationship distance’ or the cost of goods movement, respectively).
Concept of SCN robustness
From the contemporary literature in the area of modelling SCN robustness, it is evident that the terms resilience and robustness have been used interchangeably by researchers. However, in the field of network science, the terms resilience and robustness have distinct meanings. For example, a system is called robust, if it can maintain its basic functions in the presence of internal and external perturbations. Hence, a robust SCN would include redundant or parallel components, which if needed can be relied upon to maintain the overall functionality.
In contrast, resilience is defined as the capability of a system to adapt to internal or external perturbations by changing mode of operation, without losing its ability to function (Barabasi, 2014 ). Therefore, a resilient supply chain should respond quickly and effectively to a given perturbation, such as a change in supply or demand, or to the failure of a component (such as a firm or a material transport route) within the system. The response mechanism of a resilient SCN is attributable to its flexibility to rewire the lost connections away from disrupted nodes (Sheffi and Rice, 2005 ). As opposed to resilience, the robustness of a SCN does not relate to response mechanisms – it merely reflects the extent to which a given SCN can withstand loss of its components, without losing its basic functions. It is worth noting that most studies have focussed mainly on the topological robustness of SCNs, rather than their resilience.
Analytical measurements of SCN topological robustness
Network science offers a rich set of tools for topological robustness analysis. Refer to Costa et al. ( 2007 ) and Rubinov and Sporns ( 2010 ) for a comprehensive range of measures used for the characterization of complex networks. Some key metrics used in network science, and their corresponding SCN implications at node and network level are presented in Appendix 1 and 2 , respectively.
As can be seen from the metrics presented in Appendix 1 and 2 , the analytical measures in network science can be used to gain important insights on network structure and robustness quickly and with low computational difficulty. However, the key limitation of using analytical measures is that they are unable to account for the heterogeneity of nodes, in terms of their functionality within a given SCN, since the metrics consider all nodes to be homogeneous in function. In order to overcome the above limitation, researchers have relied on simulations to analyse the topological robustness of SCNs. Furthermore, simulations allow flexibility in analysis through customised robustness metrics (see section below).
Using simulations to determine the topological robustness of SCNs
Node failures in networks can be categorised either as ‘random failures’ or ‘targeted attacks’. Random failures entail the same probability of failure across each node within a given network. In contrast, a ‘targeted attack’ refers to when an attacker selectively compromises the nodes with probabilities proportional to their degrees, where highly connected nodes are compromised with higher probability (Ruj and Pal, 2014 ).
In the network science literature, random failures and targeted attacks in networks are typically simulated as follows;
Random failure : Each robustness metric established for the network is recorded at each time step by randomly removing the nodes from the network.
Targeted attacks : Each robustness metric established for the network is recorded at each time step by sequentially removing the nodes, based on their degree, removing higher degree nodes first, from the network.
The robustness values recorded for each metric, for each network considered, are then compared. It has, so far, been established that the random networks respond similarly to both random failures and targeted attacks. In comparison, the scale-free networks are robust against random failures but are highly sensitive to targeted attacks (Albert et al., 2000 ). This is due to the presence of hubs (highly connected nodes) in scale-free networks, which are the nodes targeted by an attacker.
A number of researchers have modelled various SCN topologies under both random failures and targeted attacks, and attempted to establish an optimal topology which can withstand each type of failure without compromising the overall network functionality (Thadakamalla et al., 2004 ; Zhao et al., 2011a , Li and Du, 2016 ). Each study has established a set of robustness metrics, in order to assess and compare the robustness of each network topology simulated under random failures and targeted attacks. These robustness metrics are variations of the existing standard topological metrics from network science. The most commonly used network topology metrics in supply network research are;
Size of the largest connected component (LCC) of a network - As nodes are sequentially removed, the graph disintegrates into sub-graphs. The number of nodes in the LCC (or the largest sub-graph) of a fragmented network therefore provides insights into its overall connectivity.
Average or maximum path length in the LCC - The average or maximum shortest path length between any two nodes in the largest connected component of a network. This provides insights into the overall accessibility of the network.
The above metrics consider the roles of entities (nodes and links) within a distribution network to be homogeneous. Such an assumption is far from reality, since the entities within a real-world supply network play different roles with different characteristics – for example, the distance between two supply nodes or two demand nodes are not as important as that between a supply and a demand node (Zhao et al., 2011b ).
Therefore, various researchers (such as Thadakamalla et al., 2004 ; Zhao et al., 2011a and Zhao et al., 2011b , Xu et al., 2014 ) have developed new metrics, which realistically represent the heterogeneous roles of nodes within the network. For example, Zhao et al. ( 2011a ) have developed the following customised robustness metrics for distribution networks;
Supply availability rate is represented as the percentage of demand nodes that have access to supplies from at least one supply node.
The network connectivity is determined through the size of the largest functional sub-network (LFSN), namely the number of nodes in the LFSN in which there is a path between any pair of nodes and there exists at least one supply node.
Accessibility is determined by;
○ Average supply path lengths in the LFSN, i.e. the average shortest supply path length between all pairs of supply and demand nodes in the LFSN.
○ Maximum supply path lengths in the LFSN, i.e. the maximum shortest path length between any pair of supply and demand nodes in the LFSN.
Empirical studies on SCN topologies
A review of contemporary literature on SCN topologies reveals that only a limited number of data driven studies are available in this domain. This is mainly due to difficulty in obtaining specific information about supplier/customer relationships, which is often proprietary and confidential. Table 3 presents a summary of a number of empirical studies available to date. It is noted that these studies generally focus on overall topological character of SCNs rather than robustness.
Discussion of literature – Limitations and improved methodological directions
This section will critically discuss the existing methodologies in contemporary literature, on modelling SCN topologies and robustness.
Modelling SCN topologies
Insights revealed by empirical studies.
While SCNs in real world may not evolve through a single mechanism, it is possible to infer general growth and design principles from the global properties of existing SCNs. In this regard, empirical studies play a major role in pointing the theoretical research work on modelling SCN topologies in a meaningful direction.
A number of past theoretical studies have relied upon the BA model for SCN growth and/or benchmarking purposes (Thadakamalla et al., 2004 ; Xuan et al., 2011 ; Zhao et al., 2011a ). However, based on the results of empirical studies summarised in this paper, it is understood that the BA model (due to its minimal nature) cannot sufficiently represent the growth mechanism underlying SCNs, due to the following:
The BA model generates networks with a constant power-law exponent, γ = 3, as shown by both analytical and simulation methods in Barabasi ( 2014 ). The SCNs reported in empirical studies indicate topologies with γ ≈ 2 (it is noted that γ = 2 is the boundary between hub and spoke (γ < 2) and scale-free (γ > 2) network topologies).
The BA model cannot generate networks with pronounced community structure, which has been observed in real SCNs, since all nodes in the network belong to a single weakly connected component (Newman, 2003 ).
Assortative (or disassortative) mixing as observed in real SCNs, is not a feature of networks generated by the BA model - as shown analytically (in the limit of large network size) by Newman ( 2002 ).
As can be seen above, although some real world networks have been convincingly modelled by the preferential attachment mechanism presented in the BA model (Barabasi et al., 1999 , Albert et al., 1999 ), this is not so for SCNs. Therefore, a convincing network growth mechanism for SCNs is yet to be formulated.
Suitability of network models in literature for SCN modelling purposes
Considering the limitations of the BA model in representing the topologies of real SCNs (as discussed in the previous section), the generative models which predefine a global property (such as the degree distribution, hierarchy, modularity, etc.) are a good starting point for the researchers in the SCN domain, especially when the interest of research is directed towards understanding the role of network topology on its robustness. In particular, the SCNs in the real world may have evolved based on various non generalizable principles. Therefore, when aiming to study and understand the topological character of SCNs, researchers will benefit more from simply mimicking the observed topologies, than trying to understand the underlying growth mechanism – which may indeed be complex and non-generalizable, beyond the realm of a single mathematical algorithm.
Generative models allow the researchers to recreate a network topology, as observed at a single cross section in time, and undertake further investigations on various phenomena, such as topological efficiency, robustness etc. When information on the adjacency matrix is available for real-world networks, one can simply use the DPR to generate an ensemble of random networks (which correspond to the configuration model) to establish whether the degree distribution on its own is sufficient to describe the property observed in the network at hand. It is worth noting that in tiered SCNs, the DPR process should be restricted to each tier, in order to ensure that links are not swapped between non-compatible tiers.
In many cases, adjacency matrix information for real networks are not readily available. Such situations require the researcher to recreate the degree distribution of the SCN, using only the basic network metrics (such as the power-law exponent) or simply through qualitative descriptions. In this regard, fitness based generative models have recently gained prominence in theoretical research (Caldarelli et al., 2002 ; Bedogne et al., 2006 ; Smolyarenko, 2014 ; Perera et al., 2016a ). In fitness based models, the fitness distribution and the connection rules are given by a priori arbitrary functions, which enables considerable amount of tuning (Smolyarenko, 2014 ). Indeed, this tunability makes such models a useful and practical modelling tool.
For example, the LNFA includes a tunable parameter σ (the shape parameter of the lognormal distribution), which can be manipulated to generate a large spectrum of networks. At one extreme, when σ is zero, all nodes have the same fitness and therefore at the time a new node joins the network, it chooses any existing node as a neighbour with equal probability, thus replicating the random graph model. On the other hand, when σ is increased beyond a certain threshold, very few nodes will have very large levels of fitness while the overwhelming majority of nodes have extremely low levels of fitness. As a result, the majority of new connections will be made to a few nodes which have high levels of fitness. The resulting network therefore resembles a monopolistic/“winner-take-all” scenario, which can sometimes be observed in the real world (however, in some instances, it may be necessary to place a restriction on the highest degree achievable by a single node, in order to represent the ‘contractual capacity’ of firms). Between the above two extremes (random and monopolistic) lies a spectrum of power law networks which can closely represent many real networks (Ghadge et al., 2010 ). Figure 4 illustrates the spectrum of network topologies generated by the LNFA model.
Transitions from random to winner-take-all graphs observed as σ parameter is increased
Nguyen and Tran ( 2012 ) have illustrated that the LNFA model can indeed generate network topologies with γ ≈ 2, which represents many observed SCN topologies in empirical research work (Büttner et al., 2013 ; Orenstein, 2016 ). However, the ability of LNFA to generate modular and disassortative networks, as observed in SCNs, remains an open research question.
Directionality of links
The inter-firm relationships in SCNs are generally modelled using undirected links. However, the links between nodes in a SCN can include a direction, depending on the specific type of relationship being modelled. The inter-firm relationships in a SCN can be broadly categorised into three classes, namely; (1) material flows, (2) financial flows, and (3) information exchanges. Material flows are usually unidirectional from suppliers to retailers, while financial flows are unidirectional in the opposite direction. Both material and financial flows mostly occur vertically, across the functional tiers of a SCN (however, in some cases, two firms within the same tier, such as two suppliers, could also exchange material and finances) (Lazzarini et al., 2001 ). In contrast, information exchanges are bidirectional (i.e. undirected) and includes both vertical and horizontal connections (i.e. between firms across tiers and between firms within the same tier). Therefore, the same SCN can include different topologies based on the specific type of relationship denoted by the links in the model. For instance, unlike material and financial flows, SCN topology for information exchanges can exhibit shorter path lengths and high clustering due to relatively larger number of horizontal connections (Hearnshaw and Wilson, 2013 ).
Compared to undirected network representation, in directed networks, the adjacency matrix is no longer symmetric. As a result, the degree of a node in a directed network is characterised by both in-degree and out-degree. On this basis, the degree distribution of directed networks is analysed separately for in and out degrees. Also, unlike undirected networks, in directed networks the distance between node i and node j is not necessarily the same as the distance between node j and node i. In fact, in directed networks, the presence of a path from node i to node j does not necessarily imply the presence of a path from node j to node i (Barabasi, 2014 ) . This has implications on node centrality metrics, such as closeness and betweenness. In addition, it is noted that many dynamics, such as synchronizability and percolation, are different in directed networks compared to undirected networks (Schwartz et al., 2002 ; Park and Kim, 2006 ). Therefore, when modelling SCNs, it is important to first identify the specific type of relationship denoted by the links, so that network can be correctly represented as undirected or directed.
Additional considerations for robustness testing
From a SCN point of view, the position of an individual firm with respect to the others can influence both its strategy and behaviour (Borgatti and Li, 2009 ). Accordingly, analysis of each firm’s role and importance based on its position in the SCN can reveal important properties, such as its structural robustness. In this regard, future studies could simulate targeted attacks based on node centrality measures, such as betweenness and closeness centrality, rather than node degree. Such considerations will capture the critical nodes in various perspectives. Also, as Piraveenan et al. ( 2012 ) notes, when simulating targeted attacks on empirical networks, one could also rank nodes on the basis of non-topological attributes (such as firm size, output, and geographic location).
Depending on the structure of the overall SCN, disruptions can be experienced in various forms, such as; supply disruptions, logistics disruptions, coordination disruptions and demand disruptions (Yi et al., 2013 ). These various disruptions can be attributed to either nodes or links or both, for modelling purposes. So far, the focus of modelling has been on unweighted links in SCNs, which essentially indicate that all relationships are considered to be homogeneous in terms of their relative importance. However, real SCNs exhibit large levels of heterogeneity in the capacity and intensity of the connections (links) between the nodes. Rui and Ban ( 2012 ) state that empirical observations have shown the existence of nontrivial correlations and associations between link weights and topological quantities in complex networks. In the context of SCNs, the connections, be they physical flows or relationships between organisations, are heterogeneous in terms of the strength and importance. Therefore, the SCN can be better reflected and understood in terms of weighted networks, where weights reflect volume, frequency or the criticality of flows (Hearnshaw and Wilson, 2013 ). If such information is available, targeted attacks could also be simulated by link removal on the basis of link weights.
Conclusions and future directions
This paper has presented a comprehensive and critical review of the research undertaken on the use of network science techniques to model the topology and robustness of SCNs. The key challenge in this research is the tailoring of network science principles to SCNs, by identifying the fundamental SCN features. Although network science offers a rich conceptual representation of SCNs, a number of potential improvements to the existing modelling approach have been identified and are proposed for future research.
From the literature reviewed, it is evident that most of the previous research undertaken in the field of modelling SCNs as complex networks have given primary consideration to network topology. Based on the empirical studies, it is evident that most real world SCNs tend to have power-law exponents which fluctuate around 2. It is noted that γ = 2 is the boundary between hub and spoke (γ < 2) and scale-free (γ > 2) network topologies. Also, most SCN topologies indicate disassortative mixing and modularity (the presence of communities). The well-known BA model is not able to generate network topologies with the above mentioned features. Therefore, researchers are advised to focus on generative models to mimic the SCN topologies observed in empirical studies. This approach is deemed more effective and reliable than the existing methodology of investigating the mechanisms underlying SCN evolution, particularly since the overarching goal of research in this area is to understand the role of network topology on properties such as robustness. It is emphasised that future theoretical work on development of SCN growth models should ideally aim to reflect the above outlined topological features in the network topologies obtained from generative models. A natural extension of this work would be to investigate the ability of fitness based growth models, coupled with node and link heterogeneity, to mimic the topological features, such as modularity and disassortativity, of real world SCNs.
So far, empirical studies have investigated a cross sectional view of real SCNs at a given point in time. However, databases such as Bloomberg offer rich data sets to investigate the evolution of SCNs across time. Therefore, researchers could investigate the evolution of SCNs, using temporal data. Such empirical tests can validate the theoretical network growth models developed so far in the literature.
Albert R, Barabási AL (2002) Statistical mechanics of complex networks. Rev Mod Phys 74(1):47
Article ADS MathSciNet MATH Google Scholar
Albert R, Jeong H, Barabási AL (1999) Internet: diameter of the world-wide web. Nature 401(6749):130–131
Article ADS Google Scholar
Albert R, Jeong H, Barabási AL (2000) Error and attack tolerance of complex networks. Nature 406:378–382
Barabási, A. L. (2014). Network science book. Boston : Center for Complex Network, Northeastern University . Available online at: http://barabasi.com/networksciencebook
Barabási A-L, Albert R (1999) Emergence of scaling in random networks. Science 286(5439):509–512
Bartos S (2012) Resilience in the Australian food supply chain. Department of agriculture Australian government, fisheries and forestry, Commonwealth of Australia, Canberra Retrieved from http://www.tisn.gov.au/Documents/Resilience%20in%20the%20Australian%20food%20supply%20chain%20-%20PDF%20copy%20for%20web.PDF
Google Scholar
Bar-Yam Y (2002) General features of complex systems. Encyclopedia of Life Support Systems (EOLSS), UNESCO, EOLSS Publishers, Oxford
MATH Google Scholar
Becker T, Meyer M, Windt K (2014) A manufacturing systems network model for the evaluation of complex manufacturing systems. Int J Product Perform Manag 63(3):324–340
Article Google Scholar
Bedogne C, Rodgers GJ (2006) Complex growing networks with intrinsic vertex fitness. Phys Rev E 74(4):046115
Bell M, Perera S, Piraveenan M, Bliemer M, Latty T, Reid C (2017) Network growth models: a behavioural basis for attachment proportional to fitness. Sci Rep 2017:7
Berryman MJ, Angus SD (2010) Tutorials on agent-based modelling with NetLogo and network analysis with Pajek, Complex physical, biophysical and Econophysical systems, pp 351–375
Bianconi G, Barabási AL (2001) Competition and multiscaling in evolving networks. EPL (Europhysics Letters) 54(4):436
Bianconi, Ginestra. "Processes on networks". 2016. Lecture
Bondy JA, Murty USR (1976) Graph theory with applications, vol 290. Macmillan, London
Book MATH Google Scholar
Borgatti SP, Li X (2009) On social network analysis in a supply chain context*. J Supply Chain Manag 45(2):5–22
Brintrup, A., Wang, Y., & Tiwari, A. (2015). Supply networks as complex systems: a network-science-based characterization
Brintrup A, Ledwoch A, Barros J (2016) Topological robustness of the global automotive industry. Logist Res 9(1):1–17
Buckley W (2008) Society as a complex adaptive system. Emergence: Complexity and Organization 10(3):8
Büttner K, Krieter J, Traulsen A, Traulsen I (2013) Static network analysis of a pork supply chain in northern Germany—characterisation of the potential spread of infectious diseases via animal movements. Preventive veterinary medicine 110(3):418–428
Caldarelli G, Capocci A, De Los Rios P, Munoz MA (2002) Scale-free networks from varying vertex intrinsic fitness. Phys Rev Lett 89(25):258702
Canis, Bill. (2011). Motor vehicle supply chain: effects of the Japanese earthquake and tsunami: DIANE publishing
Choi TY, Hartley JL (1996) An exploration of supplier selection practices across the supply chain. J Oper Manag 14(4):333–343
Choi TY, Hong Y (2002) Unveiling the structure of supply networks: case studies in Honda, Acura, and DaimlerChrysler. J Oper Manag 20(5):469–493
Choi TY, Dooley KJ, Rungtusanatham M (2001) Supply networks and complex adaptive systems: control versus emergence. J Oper Manag 19(3):351–366
Clauset A, Shalizi CR, Newman ME (2009) Power-law distributions in empirical data. SIAM Rev 51(4):661–703
Cohen R, Erez K, Ben-Avraham D, Havlin S (2000) Resilience of the internet to random breakdowns. Phys Rev Lett 85(21):4626
Costa LDF, Rodrigues FA, Travieso G, Villas Boas PR (2007) Characterization of complex networks: a survey of measurements. Adv Phys 56(1):167–242
Epstein JM, Axtell R (1996) Growing artificial societies: social science from the bottom up. Brookings Institution Press, Massachusetts
Erdős P, Rényi A (1959) On random graphs. I. Publ Math 6:290–297
MathSciNet MATH Google Scholar
Forrester JW (1961) Industrial dynamics. MIT Press, Cambridge
Forrester JW (1973) World dynamics. MIT Press, Cambridge
Fosdick, B. K., Larremore, D. B., Nishimura, J., & Ugander, J. (2016). Configuring random graph models with fixed degree sequences. arXiv preprint arXiv:1608.00607
Freeman LC (1977) A set of measures of centrality based on betweenness. Sociometry 1:35–41
Gang Z, Ying-Bao Y, Xu B, Qi-Yuan P (2015) On the topological properties of urban complex supply chain network of agricultural products in mainland China. Transportation Letters 7(4):188–195
Ghadge S, Killingback T, Sundaram B, Tran DA (2010) A statistical construction of power-law networks. International Journal of Parallel, Emergent and Distributed Systems 25(3):223–235
Article MathSciNet MATH Google Scholar
Gjerdrum J, Shah N, Papageorgiou LG (2001) A combined optimization and agent-based approach to supply chain modelling and performance assessment. Prod Plan Control 12(1):81–88
Hearnshaw EJ, Wilson MM (2013) A complex network approach to supply chain network theory. Int J Oper Prod Manag 33(4):442–469
Jain V, Wadhwa S, Deshmukh SG (2009) Select supplier-related issues in modelling a dynamic supply chain: potential, challenges and direction for future research. Int J Prod Res 47(11):3013–3039
Article MATH Google Scholar
Julka N, Srinivasan R, Karimi I (2002) Agent-based supply chain management—1: framework. Comput Chem Eng 26(12):1755–1769
Jüttner U, Peck H, Christopher M (2003) Supply chain risk management: outlining an agenda for future research. Int J Log Res Appl 6(4):197–210
Kaisler S, Madey G (2009) Complex adaptive systems: emergence and self-organization. Presentation, University of Notre Dame, Notre Dame
Kasthurirathna D (2015) The influence of topology and information diffusion on networked game dynamics (ph.D). University of Sydney, Sydney
Keqiang W, Zhaofeng Z, Dongchuan S (2008) Structure analysis of supply chain networks based on complex network theory, In Semantics, Knowledge and Grid, 2008. SKG'08. Fourth International Conference on . IEEE, USA, pp 493–494
Kim Y, Choi TY, Yan T, Dooley K (2011) Structural investigation of supply networks: a social network analysis approach. J Oper Manag 29(3):194–211
Kim Y, Chen YS, Linderman K (2015) Supply network disruption and resilience: a network structural perspective. J Oper Manag 33:43–59
Kito T, Brintrup A, New S, Reed-Tsochas F (2014) The structure of the Toyota supply network: an empirical analysis. Saïd Business School WP 2014:3
Ladyman J, Lambert J, Wiesner K (2013) What is a complex system?. European . Journal for Philosophy of Science 3(1):33–67
Lazzarini S, Chaddad F, Cook M (2001) Integrating supply chain and network analyses: the study of netchains. Journal on chain and network science 1(1):7–22
Lee HL (2004) The triple-a supply chain. Harv Bus Rev 82(10):102–113
Li Y (2014) Networked analysis approach of supply chain network. Journal of Networks 9(3):777–784
Li Y, Du ZP (2016) Agri-food supply chain network robustness research based on complex network, In proceedings of the 6th international Asia conference on industrial engineering and management innovation. Atlantis Press, Paris, pp 929–938
Li G, Gu YG, Song ZH (2013) Evolution of cooperation on heterogeneous supply networks. Int J Prod Res 51(13):3894–3902
Manuj I, Mentzer JT (2008) Global supply chain risk management strategies. Int J Phys Distrib Logist Manag 38(3):192–223
Mari SI, Lee YH, Memon MS, Park YS, Kim M (2015) Adaptivity of complex network topologies for designing resilient supply chain networks. International Journal of Industrial Engineering 22(1):102–116
Maslov S, Sneppen K (2002) Specificity and stability in topology of protein networks. Science 296(5569):910–913
Mina AA, Braha D, Bar-Yam Y (2006) Complex engineered systems: a new paradigm, In complex engineered systems. Springer, Heidelberg, pp 1–21
Book Google Scholar
Nair A, Vidal JM (2011) Supply network topology and robustness against disruptions–an investigation using multi-agent model. Int J Prod Res 49(5):1391–1404
Newman ME (2002) Assortative mixing in networks. Phys Rev Lett 89(20):208701
Newman ME (2003) The structure and function of complex networks. SIAM Rev 45(2):167–256
Newman ME (2005) Power laws, Pareto distributions and Zipf's law. Contemp Phys 46(5):323–351
Newman ME, Girvan M (2004) Finding and evaluating community structure in networks. Phys Rev E 69(2):026113
Newman M, Barabasi AL, Watts DJ (2011) The structure and dynamics of networks. Princeton University Press, Princeton
Nguyen K, Tran DA (2012) Fitness-based generative models for power-law networks, In handbook of optimization in complex networks. Springer US, New Mexico, pp 39–53
Noldus R, Van Mieghem P (2015) Assortativity in complex networks. Journal of . Complex Networks 3(4):507–542
Article MathSciNet Google Scholar
Orenstein P (2016) How does supply network evolution and its topological structure impact supply chain performance? In 2016 Second International Symposium on Stochastic Models in Reliability Engineering, Life Science and Operations Management (SMRLO) . IEEE, USA, pp 562–569
Parhi M (2008) Impact of the changing facets of inter-firm interactions on manufacturing excellence: a social network perspective of the Indian automotive industry. Asian Journal of Technology Innovation 16(1):117–141
Park SM, Kim BJ (2006) Dynamic behaviors in directed networks. Phys Rev E 74(2):026114
Parunak HVD, Savit R, Riolo RL (1998) Agent-based modeling vs. equation-based modeling: a case study and users’ guide, In Multi-agent systems and agent-based simulation . Springer, Heidelberg, pp 10–25
Pathak SD, Day JM, Nair A, Sawaya WJ, Kristal MM (2007) Complexity and adaptivity in supply networks: building supply network theory using a complex adaptive systems perspective*. Decis Sci 38(4):547–580
Perera S, Bell MGH, Bliemer MCJ (2016a) Resilience characteristics of supply network topologies generated by fitness based growth models. 95th Annual Meeting of the Transportation Research Board TRB, Washington, D.C. United States, 14th January 2016
Perera S, Bell M, Piraveenan M, Bliemer M (2016b) Empirical investigation of supply chain structures using network theory. 6th International Conference on Logistics and Maritime Systems, Sydney Retrieved from http://logms2016.org/authors-information/
Piraveenan M, Uddin S, Chung KSK (2012, August) Measuring topological robustness of networks under sustained targeted attacks, In Advances in Social Networks Analysis and Mining (ASONAM), 2012 IEEE/ACM International Conference on . IEEE, USA, pp 38–45
Pruteanu, A. (2013). Mastering emergent behavior in large-scale networks
Rahmandad H, Sterman J (2008) Heterogeneity and network structure in the dynamics of diffusion: comparing agent-based and differential equation models. Manag Sci 54(5):998–1014
Ravasz E, Somera AL, Mongru DA, Oltvai ZN, Barabási AL (2002) Hierarchical organization of modularity in metabolic networks. Science 297(5586):1551–1555
Rubinov M, Sporns O (2010) Complex network measures of brain connectivity: uses and interpretations. NeuroImage 52(3):1059–1069
Ruhnau B (2000) Eigenvector-centrality—a node-centrality? Soc Networks 22(4):357–365
Rui Y, Ban Y (2012) Nonlinear growth in weighted networks with neighborhood preferential attachment. Physica A: Statistical Mechanics and its Applications 391(20):4790–4797
Ruj S, Pal A (2014, May) Analyzing cascading failures in smart grids under random and targeted attacks, In Advanced Information Networking and Applications (AINA), 2014 IEEE 28th International Conference on . IEEE, USA, pp 226–233
Sabidussi G (1966) The centrality index of a graph. Psychometrika 31(4):581–603
Schwartz N, Cohen R, Ben-Avraham D, Barabási AL, Havlin S (2002) Percolation in directed scale-free networks. Phys Rev E 66(1):015104
Article ADS MathSciNet Google Scholar
Sheffi Y (2001) Supply chain management under the threat of international terrorism. The International Journal of logistics management 12(2):1–11
Sheffi Y, Rice JB Jr (2005) A supply chain view of the resilient enterprise. MIT Sloan Manag Rev 47(1):41
Shen Z, Ma KL, Eliassi-Rad T (2006) Visual analysis of large heterogeneous social networks by semantic and structural abstraction. Visualization and . Computer Graphics, IEEE Transactions on 12(6):1427–1439
Smolyarenko IE (2014) Fitness-based network growth with dynamic feedback. Phys Rev E 89(4):042814
Song C, Havlin S, Makse HA (2006) Origins of fractality in the growth of complex networks. Nat Phys 2(4):275–281
Sun JY, Tang JM, Fu WP, Wu BY (2017) Hybrid modeling and empirical analysis of automobile supply chain network. Physica A: Statistical Mechanics and its Applications 473:377–389
Surana A, Kumara S, Greaves M, Raghavan UN (2005) Supply-chain networks: a complex adaptive systems perspective. Int J Prod Res 43(20):4235–4265
Tett G. (2011). Japan supply chain risk reverberates globally - financial Times.Com . Retrieved from https://www.ft.com/content/fc3936a6-4f2f-11e0-9038-00144feab49a
Thadakamalla HP, Raghavan UN, Kumara S, Albert R (2004) Survivability of multiagent-based supply networks: a topological perspective. Intelligent Systems, IEEE 19(5):24–31
Vázquez A (2003) Growing network with local rules: preferential attachment, clustering hierarchy, and degree correlations. Phys Rev E 67(5):056104
Vespignani A (2010) Complex networks: the fragility of interdependency. Nature 464(7291):984–985
Watts DJ, Strogatz SH (1998) Collective dynamics of ‘small-world’ networks. Nature 393(6684):440–442
Article ADS MATH Google Scholar
Wen L, Guo M (2012) Statistic characteristics analysis of directed supply chain complex network. International Journal of Advancements in Computing Technology 4(21)
West DB (2001) Introduction to graph theory, vol 2. Prentice hall, Upper Saddle River
Willems, SP (2008) Data set—Real-world multiechelon supply chains used for inventory optimization. Manufacturing & Service Operations Management 10(1):19–23.
World Economic Forum. (2013). Building resilience in supply chains. Retrieved from http://www3.weforum.org/docs/WEF_RRN_MO_BuildingResilienceSupplyChains_Report_2013.pdf
Xu M, Wang X, Zhao L (2014) Predicted supply chain resilience based on structural evolution against random supply disruptions. International Journal of Systems Science: Operations & Logistics 1(2):105–117
Xu NR, Liu JB, Li DX, Wang J (2016) Research on evolutionary mechanism of agile supply chain network via complex network theory. Math Probl Eng 2016:9
Xuan Q, Du F, Li Y, Wu TJ (2011) A framework to model the topological structure of supply networks. Automation Science and Engineering, IEEE Transactions on 8(2):442–446
Yi CQ, Meng SD, Zhang DM, Li JD (2013) Managing disruption risks in supply chain based on complex networks. Journal of Convergence Information Technology 8(5):175–184
Zhang Y, Xiong J, Feng C (2012) Robustness analysis of supply chain network based on complex networks. Computer Simulation 11:087
Zhao K, Kumar A, Yen J (2011a) Achieving high robustness in supply distribution networks by rewiring. Engineering Management, IEEE Transactions 58(2):347–362
Zhao K, Kumar A, Harrison TP, Yen J (2011b) Analyzing the resilience of complex supply network topologies against random and targeted disruptions. Systems Journal, IEEE 5(1):28–39
Download references
The authors would like to acknowledge the Australian Research Council (ARC) for funding this work under grant DP140103643.
Availability of data and materials
Not applicable.
Author information
Authors and affiliations.
Institute of Transport and Logistics (ITLS), University of Sydney Business School, Darlington, NSW, 2006, Australia
Supun Perera, Michael G.H. Bell & Michiel C.J. Bliemer
You can also search for this author in PubMed Google Scholar
Contributions
SP carried out the initial review of literature and prepared the draft manuscript. M.G.H. Bell and M.C.J. Bliemer participated in discussions where limitations in existing research methods were identified and improvements proposed. SP and M.G.H. Bell prepared the final manuscript. All authors read and approved the final manuscript.
Corresponding author
Correspondence to Supun Perera .
Ethics declarations
Ethics approval and consent to participate, consent for publication, publisher’s note.
Springer Nature remains neutral with regard to jurisdictional claims in published maps and institutional affiliations.
LIST OF NETWORK LEVEL METRICS AND THEIR SCN IMPLICATIONS.
LIST OF NODE LEVEL METRICS AND THEIR SCN IMPLICATIONS.
Rights and permissions
Open Access This article is distributed under the terms of the Creative Commons Attribution 4.0 International License ( http://creativecommons.org/licenses/by/4.0/ ), which permits unrestricted use, distribution, and reproduction in any medium, provided you give appropriate credit to the original author(s) and the source, provide a link to the Creative Commons license, and indicate if changes were made.
Reprints and permissions
About this article
Cite this article.
Perera, S., Bell, M.G. & Bliemer, M.C. Network science approach to modelling the topology and robustness of supply chain networks: a review and perspective. Appl Netw Sci 2 , 33 (2017). https://doi.org/10.1007/s41109-017-0053-0
Download citation
Received : 01 June 2017
Accepted : 29 August 2017
Published : 10 October 2017
DOI : https://doi.org/10.1007/s41109-017-0053-0
Share this article
Anyone you share the following link with will be able to read this content:
Sorry, a shareable link is not currently available for this article.
Provided by the Springer Nature SharedIt content-sharing initiative
- Network science
- Supply chain network modelling
- Supply network topology and robustness
- Fitness based attachment

An official website of the United States government
The .gov means it’s official. Federal government websites often end in .gov or .mil. Before sharing sensitive information, make sure you’re on a federal government site.
The site is secure. The https:// ensures that you are connecting to the official website and that any information you provide is encrypted and transmitted securely.
- Publications
- Account settings
- My Bibliography
- Collections
- Citation manager
Save citation to file
Email citation, add to collections.
- Create a new collection
- Add to an existing collection
Add to My Bibliography
Your saved search, create a file for external citation management software, your rss feed.
- Search in PubMed
- Search in NLM Catalog
- Add to Search
Network science approach to modelling the topology and robustness of supply chain networks: a review and perspective
Affiliation.
- 1 Institute of Transport and Logistics (ITLS), University of Sydney Business School, Darlington, NSW 2006 Australia.
- PMID: 30443587
- PMCID: PMC6214257
- DOI: 10.1007/s41109-017-0053-0
Due to the increasingly complex and interconnected nature of global supply chain networks (SCNs), a recent strand of research has applied network science methods to model SCN growth and subsequently analyse various topological features, such as robustness. This paper provides: (1) a comprehensive review of the methodologies adopted in literature for modelling the topology and robustness of SCNs; (2) a summary of topological features of the real world SCNs, as reported in various data driven studies; and (3) a discussion on the limitations of existing network growth models to realistically represent the observed topological characteristics of SCNs. Finally, a novel perspective is proposed to mimic the SCN topologies reported in empirical studies, through fitness based generative network models.
Keywords: Fitness based attachment; Network science; Supply chain network modelling; Supply network topology and robustness.
PubMed Disclaimer
Conflict of interest statement
Not applicable.Not applicable.Springer Nature remains neutral with regard to jurisdictional claims in published maps and institutional affiliations.
Comparison of random, small-world and…
Comparison of random, small-world and scale free networks. Topological structure of benchmark network…
Modelling Perspectives obtained from Generative…
Modelling Perspectives obtained from Generative and Evolving Network Models
General methodological framework of research…
General methodological framework of research on topology and robustness of SCNs
Transitions from random to winner-take-all…
Transitions from random to winner-take-all graphs observed as σ parameter is increased
Similar articles
- Resilience of supply-chain systems under perturbations: A network approach. Zhou W, Zhang Q. Zhou W, et al. Chaos. 2022 Sep;32(9):093123. doi: 10.1063/5.0096983. Chaos. 2022. PMID: 36182389
- Topological motifs populate complex networks through grouped attachment. Choi J, Lee D. Choi J, et al. Sci Rep. 2018 Aug 23;8(1):12670. doi: 10.1038/s41598-018-30845-4. Sci Rep. 2018. PMID: 30140017 Free PMC article.
- Detecting differences in the topology of scale-free networks grown under time-dynamic topological fitness. Tsiotas D. Tsiotas D. Sci Rep. 2020 Jun 30;10(1):10630. doi: 10.1038/s41598-020-67156-6. Sci Rep. 2020. PMID: 32606368 Free PMC article.
- Communication dynamics in complex brain networks. Avena-Koenigsberger A, Misic B, Sporns O. Avena-Koenigsberger A, et al. Nat Rev Neurosci. 2017 Dec 14;19(1):17-33. doi: 10.1038/nrn.2017.149. Nat Rev Neurosci. 2017. PMID: 29238085 Review.
- Serous Neoplasms of the Pancreas: A Clinicopathologic Analysis of 193 Cases and Literature Review With New Insights on Macrocystic and Solid Variants and Critical Reappraisal of So-called "Serous Cystadenocarcinoma". Reid MD, Choi HJ, Memis B, Krasinskas AM, Jang KT, Akkas G, Maithel SK, Sarmiento JM, Kooby DA, Basturk O, Adsay V. Reid MD, et al. Am J Surg Pathol. 2015 Dec;39(12):1597-610. doi: 10.1097/PAS.0000000000000559. Am J Surg Pathol. 2015. PMID: 26559376 Review.
- Assessing the impacts of vaccination and viral evolution in contact networks. Blanco-Rodríguez R, Tetteh JNA, Hernández-Vargas E. Blanco-Rodríguez R, et al. Sci Rep. 2024 Jul 8;14(1):15753. doi: 10.1038/s41598-024-66070-5. Sci Rep. 2024. PMID: 38977773 Free PMC article.
- Analysis of network disruption evolution of Chinese fresh cold chain under COVID-19. Chen H, Chen G, Zhang Q, Zhang X. Chen H, et al. PLoS One. 2023 Jan 26;18(1):e0278697. doi: 10.1371/journal.pone.0278697. eCollection 2023. PLoS One. 2023. PMID: 36701281 Free PMC article.
- Local topological features of robust supply networks. Lyutov A, Uygun Y, Hütt MT. Lyutov A, et al. Appl Netw Sci. 2022;7(1):33. doi: 10.1007/s41109-022-00470-2. Epub 2022 May 20. Appl Netw Sci. 2022. PMID: 35615080 Free PMC article.
- Niche differentiation drives microbial community assembly and succession in full-scale activated sludge bioreactors. de Celis M, Duque J, Marquina D, Salvadó H, Serrano S, Arregui L, Santos A, Belda I. de Celis M, et al. NPJ Biofilms Microbiomes. 2022 Apr 11;8(1):23. doi: 10.1038/s41522-022-00291-2. NPJ Biofilms Microbiomes. 2022. PMID: 35411053 Free PMC article.
- Testing "efficient supply chain propositions" using topological characterization of the global supply chain network. Chakraborty A, Ikeda Y. Chakraborty A, et al. PLoS One. 2020 Oct 1;15(10):e0239669. doi: 10.1371/journal.pone.0239669. eCollection 2020. PLoS One. 2020. PMID: 33002029 Free PMC article.
- Albert R, Barabási AL. Statistical mechanics of complex networks. Rev Mod Phys. 2002;74(1):47. doi: 10.1103/RevModPhys.74.47. - DOI
- Albert R, Jeong H, Barabási AL. Internet: diameter of the world-wide web. Nature. 1999;401(6749):130–131. doi: 10.1038/43601. - DOI
- Albert R, Jeong H, Barabási AL. Error and attack tolerance of complex networks. Nature. 2000;406:378–382. doi: 10.1038/35019019. - DOI - PubMed
- Barabási, A. L. (2014). Network science book. Boston: Center for Complex Network, Northeastern University. Available online at: http://barabasi.com/networksciencebook
- Barabási A-L, Albert R. Emergence of scaling in random networks. Science. 1999;286(5439):509–512. doi: 10.1126/science.286.5439.509. - DOI - PubMed
Publication types
- Search in MeSH
Related information
Linkout - more resources, full text sources.
- Europe PubMed Central
- PubMed Central
- Citation Manager
NCBI Literature Resources
MeSH PMC Bookshelf Disclaimer
The PubMed wordmark and PubMed logo are registered trademarks of the U.S. Department of Health and Human Services (HHS). Unauthorized use of these marks is strictly prohibited.
Thank you for visiting nature.com. You are using a browser version with limited support for CSS. To obtain the best experience, we recommend you use a more up to date browser (or turn off compatibility mode in Internet Explorer). In the meantime, to ensure continued support, we are displaying the site without styles and JavaScript.
- View all journals
- Explore content
- About the journal
- Publish with us
- Sign up for alerts
- Published: 19 August 2021
Network analysis of multivariate data in psychological science
- Denny Borsboom ORCID: orcid.org/0000-0001-9720-4162 1 ,
- Marie K. Deserno 2 ,
- Mijke Rhemtulla 3 ,
- Sacha Epskamp 1 , 4 ,
- Eiko I. Fried 5 ,
- Richard J. McNally 6 ,
- Donald J. Robinaugh 7 ,
- Marco Perugini ORCID: orcid.org/0000-0002-4864-6623 8 ,
- Jonas Dalege 9 ,
- Giulio Costantini 8 ,
- Adela-Maria Isvoranu ORCID: orcid.org/0000-0001-7981-9198 1 ,
- Anna C. Wysocki 3 ,
- Claudia D. van Borkulo 1 , 4 ,
- Riet van Bork ORCID: orcid.org/0000-0002-4772-8862 10 &
- Lourens J. Waldorp 1
Nature Reviews Methods Primers volume 1 , Article number: 58 ( 2021 ) Cite this article
85k Accesses
421 Citations
398 Altmetric
Metrics details
- Scientific data
An Author Correction to this article was published on 21 February 2022
This article has been updated
In recent years, network analysis has been applied to identify and analyse patterns of statistical association in multivariate psychological data. In these approaches, network nodes represent variables in a data set, and edges represent pairwise conditional associations between variables in the data, while conditioning on the remaining variables. This Primer provides an anatomy of these techniques, describes the current state of the art and discusses open problems. We identify relevant data structures in which network analysis may be applied: cross-sectional data, repeated measures and intensive longitudinal data. We then discuss the estimation of network structures in each of these cases, as well as assessment techniques to evaluate network robustness and replicability. Successful applications of the technique in different research areas are highlighted. Finally, we discuss limitations and challenges for future research.
Similar content being viewed by others
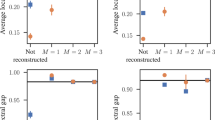
Statistical inference links data and theory in network science
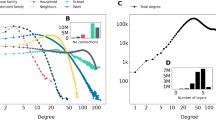
The anatomy of a population-scale social network
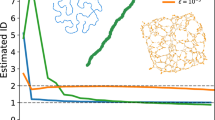
Intrinsic dimension as a multi-scale summary statistics in network modeling
Introduction.
In many scientific fields, researchers study phenomena best characterized at the systems level 1 . To understand such phenomena, it is often insufficient to focus on the way individual components of a system operate. Instead, one must also study the organization of the system’s components, which can be represented in a network 2 . The value of analysing the structure of a system in this way has been underscored by the advent of network science, which has delivered important insights into diverse sets of phenomena studied across the sciences 3 , 4 . This Primer discusses methodology to apply this line of reasoning to the statistical analysis of multivariate data.
Network approaches involve the identification of system components (network nodes) and the relations among them (links between nodes). Well-known examples include semantic networks (in which concepts are connected through shared meanings 5 ), social networks (in which people are connected through acquaintance 6 ) and neural networks (in which neurons are connected through axons 7 ). After nodes and links are identified, and a network has been constructed, one can study its topology using descriptive tools of network science 8 . For instance, one can describe the global topology of a network (such as a small-world network or random graph 9 ) or the position of individual nodes within the network (for example, by assessing node centrality 10 ). These analyses are often carried out with the goal of relating structural features of the network to system dynamics 4 , 11 .
Network representations have a long history as research tools in statistics, where they encode important information concerning the joint probability distribution of a set of variables 12 . For instance, in graphical models, unconnected nodes are conditionally independent given all or a subset of other nodes in the network 12 ; in causal models, graphical criteria are used to determine whether parameters in an estimated causal model are identified 13 ; and in structural equation models, path-tracing rules on network representations are used to determine the value of empirical correlations implied by the model 14 .
In this Primer, we present network analysis of multivariate data as a method that combines both multivariate statistics and network science to investigate the structure of relationships in multivariate data. This approach identifies network nodes with variables and links between nodes and describes them with statistical parameters that connect these variables (for example, partial correlations). Statistical models are used to assess the parameters that define the links in the network, in a process known as network structure estimation . Then, using a process of network description , the resulting network is characterized using the tools of network science 15 , 16 , 17 . Here, we refer to this combined procedure of network structure estimation and network description as psychometric network analysis (Fig. 1 ).

Joint probability distribution of multivariate data characterized in terms of conditional associations and independencies. Conditional independencies translate into disconnected nodes; conditional associations translate into links between nodes, typically weighted by the strength of the association. The resulting structure is subsequently described and analysed as a network.
Network approaches to multivariate data can be used to advance several different goals. First, they can be used to explore the structure of high-dimensional data in the absence of strong prior theory on how variables are related. In these analyses, psychometric network analysis complements existing techniques for the exploratory analysis of psychological data, such as exploratory factor analysis (which aims to represent shared variance due to a small number of latent variables) and multidimensional scaling (which aims to represent similarity relations between objects in a low-dimensional metric space). The unique focus of psychometric network analysis is on the patterns of pairwise conditional dependencies that are present in the data. Second, network representations can be used to communicate multivariate patterns of dependency effectively, because they offer powerful visualizations of patterns of statistical association. Third, network models can be used to generate causal hypotheses, as they represent statistical structures that may offer clues to causal dynamics; for instance, networks that represent conditional independence relations form a gateway that connects correlations to causal relations 13 , 18 , 19 .
Here, we review these functions of network analysis in the context of three types of application in psychological science, illustrating them with examples taken from personality, attitude research and mental health.
Experimentation
The schematic workflow of psychometric network analysis as discussed in this paper is represented in Fig. 2 . Typically, one starts with a research question that dictates a data collection scheme, which includes cross-sectional designs, time-series designs and panel designs. Psychometric network analysis begins with node selection , a choice primarily driven by substantive rather than methodological considerations. The core of the psychometric network analysis methodology then lies in the steps of network structure estimation, network description and network stability analysis . Importantly, inferences drawn from the output of network analytic methods require both substantive domain knowledge and general methodological considerations regarding the stability and robustness of the estimated network in order to optimally inform scientific inference.
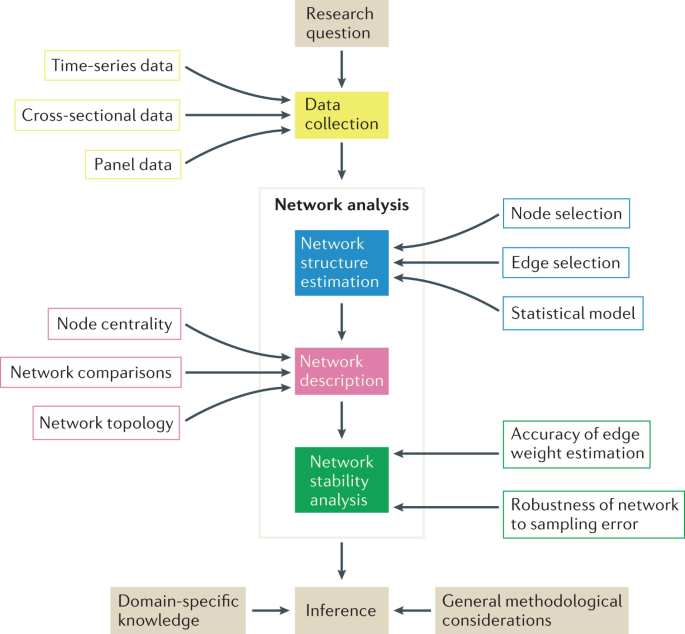
The heart of the psychometric network analysis methodology described lies in the steps of network structure estimation (to construct the network), network description (to characterize the network) and network stability analysis (to assess the robustness of results). These steps are informed by substantive research questions and data collection procedures. Output of the network approaches combines with general methodological considerations and domain-specific knowledge to support scientific inference.
Network approaches to multivariate data are based on generic statistical procedures and thus invite applications to many types of data. The approaches discussed in this paper, however, have been developed and typically used in the context of psychometric variables such as responses to questionnaire items, symptom ratings and cognitive test scores 20 , possibly extended with background variables such as age and gender 21 , genetic information 22 , physiological markers 23 , medical conditions 24 , experimental interventions 25 and anticipated downstream effects 26 . Accordingly, the nodes we discuss will ordinarily represent items and tests.
The majority of network modelling approaches use conditional associations to define the network structure prevalent in a set of variables 20 , 27 . A conditional association between two variables holds when these variables are probabilistically dependent, conditional on all other variables in the data. Which measure of conditional association to use depends on the structure of the data; for instance, for multivariate normal data, partial correlations would be indicated, whereas for binary data, logistic regression coefficients may be used. The strength of this conditional association is typically represented in the network as an edge weight that describes the connection between two nodes. If the association between two variables can be explained by other variables in the network, so that their conditional association vanishes when these other variables are controlled for, then the corresponding nodes are disconnected in the network representation.
The description of the joint probability distribution of a set of variables in terms of pairwise statistical interactions is a graphical model 12 known as the pairwise Markov random field (PMRF) 27 . Versions of the PMRF are known under several other names as well in the statistical literature; see refs 28 , 29 for an overview of the relations between relevant statistical models. Many network modelling approaches attempt to estimate the PMRF, typically using existing statistical methodologies such as significance testing 30 , cross-validation 31 , information filtering 32 and regularized estimation 16 , 33 , 34 , 35 , 36 . Because of its prominence in the literature, this Primer is limited to network approaches that use the PMRF, although it should be noted that other approaches to the analysis of multivariate data exist, including models based on zero-order associations 37 , self-reported causal relations between variables 38 , 39 and relative importance of variables 40 .
Because, in typical multivariate data, a substantive subset of associations between variables vanishes upon conditioning, applications of network modelling generally return non-trivial topological structures and the description of such structures is an important goal of psychometric network analysis. For instance, the extent to which network nodes are connected and the network’s general topology are of interest, as well as the position of individual nodes in that structure. Thus, psychometric network analysis typically involves interpreting the output of statistical estimation procedures, for example an estimated PMRF, as the input for network description techniques taken from network science (Fig. 1 ).
Types of data
Network models always operate on associations among sets of variables, but such associations can be extracted from many different experimental and quasi-experimental designs. We focus on three designs that represent typical data environments in social science where psychometric network analysis can be relevant: cross-sectional networks, longitudinal networks of panel data and time-series networks (Fig. 3 ).
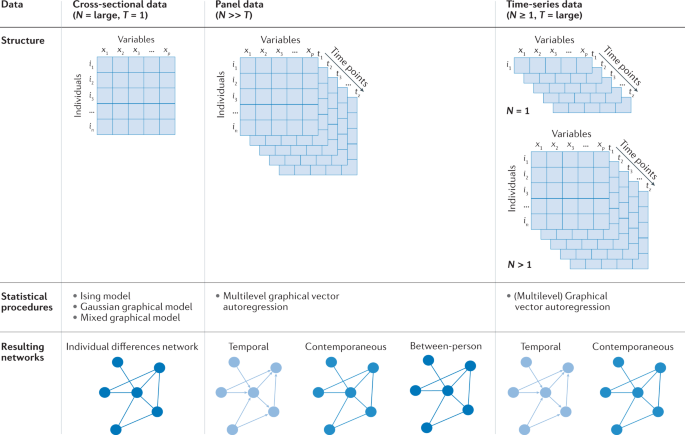
Typical data types include cross-sectional, panel and time-series data.
Cross-sectional data
In applications to cross-sectional data, networks are representations of the conditional associations between variables measured at a single time point in a large sample ( T = 1, N = large). In this case, the associations between variables are driven by individual differences, which renders such networks useful for studying the psychometric structure of psychological tests 29 . In the cross-sectional data example used here, we are interested in the empirical relations among personality and personal goals. We analyse a data set in which three levels of personality structure are assessed via questionnaires, using network models to investigate empirical relations among these elements and personal goals. Our illustrative personality data set features 432 observations and 39 variables of interest 41 .
We represent network structures as they arise at different levels of aggregation 42 at which personality can be described. These can be higher-order traits, such as conscientiousness ; facets , such as orderliness, industriousness and impulse control 43 ; or even specific single items, such as prudent, reflective and disciplined (items of impulse control 44 ) that allow for a finer distinction of personality characteristics below facets (see ref. 45 for an example). The objective of psychometric network analysis, in this case, would be to offer insight into the multivariate pattern of conditional dependencies that characterize the joint distribution of these variables at these different levels of aggregation (Box 1 ).
When cross-sectional data are analysed through network estimation and interpreted via network description, is it important to keep in mind that resulting topologies represent structures that describe differences between individuals, and that these are not necessarily isomorphic to processes or mechanisms that characterize the individuals who make up the data. That is, inter-individual differences do not necessarily translate to intra-individual processes 46 , 47 . If one is interested solely in the structure of individual differences, cross-sectional data are adequate, but research into intra-individual dynamics ideally complements such data sources with panel data or time series.
Box 1 Psychometric structure of personality test scores
A substantial part of the literature on human personality is concerned with the psychometric structure of personality tests. Research has shown that people’s self-ratings on adjectives (such as outgoing, punctual and nervous) or responses to items that characterize them (I make friends easily, I get stressed out easily; see the International Personality Item Pool for an overview of psychometric items) show systematic patterns of correlations. These patterns of correlations are often described by a low-dimensional factor model; most often, solutions with five factors known as the Five Factor Model 142 or with six factors known as HEXACO 143 are proposed. The factors in the Five Factor Model are often interpreted as latent variables that cause the correlations between the item scores. However, attempts to ground these latent variables in psychological or biological theories of human functioning have met with limited success, and correlations between personality items may have other causes that include content overlap and the presence of direct relations between properties measured by these items 69 . Such hypotheses are consistent with the finding that items in personality scales typically either load on several factors simultaneously or feature correlated residuals, suggesting that the latent variable model does not fully account for the correlations between item scores. Recently, network models have been proposed as an alternative representation of the psychometric structure of personality tests that does not require a priori commitment to a particular generating model (such as a latent variable model) and may serve to identify alternative mechanisms that lead to correlations between items 44 , 144 . An exploratory factor model and a network model are visualized in the figure using IPIP-Big Five Factor Markers open data 145 .
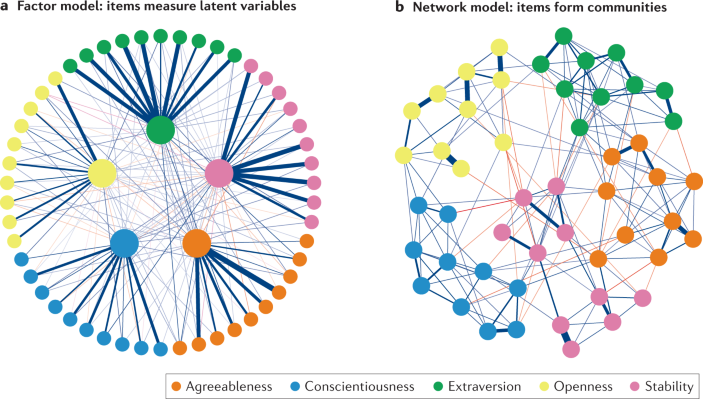
In network applications to longitudinal data (also referred to as panel data), a limited set of repeated measurements characterize both the association structure of variables at a given time point and the way these conditional dependencies’ change over time ( N > T ). Such measures can illuminate the structure of individual differences and intra-individual change in parallel.
In our example for network approaches to panel data, we use repeated assessments of emotions and beliefs towards Bill Clinton as represented in longitudinal panel data of the American National Election Studies (ANES) between 1992 and 1996. We aim to model consistency, stability and extremity of attitudes towards Bill Clinton during the time that he transitioned from governor of Arkansas to president of the United States. The network theory of attitudes (Box 2 ) formalizes changes in attitude importance as network temperature , for example, increasing or decreasing interdependence between attitude elements. In the panel data example, network analyses can assist in modelling temperature changes in the interdependence of attitude elements towards BillClinton.
Box 2 Causal attitude network model and attitudinal entropy
The network theory of attitudes holds that attitudes are higher-level properties emerging from lower-level beliefs, feelings and behaviours 111 . A negative attitude towards a politician might emerge from negative beliefs (that the politician is incompetent and bad for the future of the country), feelings (anger and frustration towards the politician) and behaviours (voting behaviour and making jokes about the politician). These different attitude elements can be modelled as nodes in a network, in which edges between attitude elements represent potentially bidirectional interactions between the elements. The network theory of attitudes relies on the central principle that interdependence between attitude elements increases when the attitude is important to the person and when an individual directs attention to the attitude object 111 . This theory uses analogical modelling of statistical mechanics and the effect of attitude importance, and attention is formalized as a decrease in temperature. The effect of decreasing network temperature is that the entropy of a multivariate system decreases by making (attitude) elements in the system more interdependent. In the case of attitudes, this effect translates to heightened consistency and stability of the attitude when it is important, because the different attitude elements rein each other in under low temperature compared with high temperature (see the figure, parts a and b ). Low temperature leads to low variance of the overall attitude within an individual, and hence higher stability. By contrast, a group of individuals with low-temperature attitude networks have higher variance than a high-temperature group, because the pressure of attitude elements to align leads to higher extremity of the overall attitude, creating a bimodal distribution. As this bimodal distribution only occurs in a low-temperature/high-importance scenario, the network model offers a potential explanation for polarization: higher importance leads to more strongly connected networks, which in turn produces polarized attitudes.
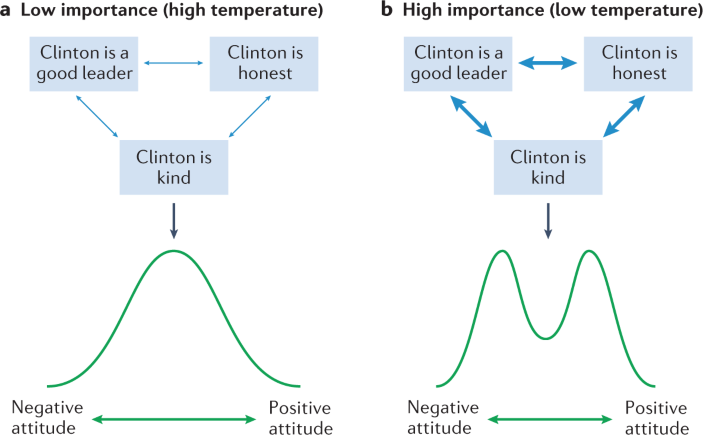
Time-series data
Networks as applied to time-series data of one or multiple persons characterize multivariate dependencies between time series of variables that are assessed intra-individually ( T = large, N ≥ 1). Such networks are most often applied in situations where one seeks insight into the dynamic structure of systems. For instance, in the social and clinical sciences, recent years have witnessed a surge of daily diary studies and ecological momentary assessment , conducted via smartphones and designed to study such dynamic structures. Studies typically measure experiences — such as mood states, symptoms, cognitions and behaviours — at the moment they occur 48 , 49 . In such cases, network analyses can assist in interpreting intensive longitudinal data by offering insightful characterizations of the multivariate pattern of dynamics.
In the time-series data example used here, we leverage data gathered during the onset of the COVID-19 pandemic to investigate the impact of reduced social contact due to lockdown measures on the mental health of students enrolled at Leiden University in the Netherlands. In this ecological momentary assessment study, students were followed daily for 2 weeks, assessing momentary social contact as well as current stress, anxiety and depression 4 times per day via a smartphone application 50 . In this situation, a network model can be fitted to these data to investigate to what degree social contact variables influence mental health variables over the course of hours and days. Because, in this case, multiple individuals were assessed multiple times, the design is mixed; in such situations, it is often profitable to use a statistical multilevel approach 27 , 51 , in which the repeated observations are treated as nested in the individuals. This explicitly separates individual differences from time dynamics 52 .
In a PMRF, the joint likelihood of multivariate data is modelled through the use of pairwise conditional associations, leading to a network representation that is undirected. There are several benefits to the PMRF that make this particular network representation important. First, the PMRF encodes conditional independence relations (in terms of absent links between nodes), which form an important gateway to identify candidate data-generating mechanisms 29 , 53 , 54 . However, the PMRF does not require an a priori commitment to any particular data-generating mechanism (unlike directed acyclic graph estimation or latent variable modelling, for example). Because PMRFs do not place strong assumptions on the structure of the generating model but do hold clues to causal structure through conditional independencies, they are well suited to exploratory analyses (see also Limitations and optimizations). In addition, estimated PMRFs often describe the data successfully with only a subset of the possible parameters (for example, using sparse network structures), which leads to more insightful network visualizations. Finally, a priori commitments invariably lead to problems of underdetermination , because many structurally different models will produce indistinguishable data, which is known as statistical equivalence. By contrast, the PMRF is uniquely identified, so there are no two equivalent PMRFs with different parameters that fit the data equally well.
If data are continuous, a popular type of PMRF is the Gaussian graphical model (also known as a partial correlation network) in which edges are parameterized as partial correlation coefficients 55 , 56 . If data are binary, a popular PMRF developed to estimate the Ising model can be used, in which edges are parameterized as log-linear relationships 16 , 29 , 36 . The Ising model and the Gaussian graphical model can be combined in mixed graphical models , in which edges are parameterized as regression coefficients from generalized linear regression models 57 . Mixed graphical models represent the most general approach to PMRF estimation and also allow for the inclusion of categorical and count variables.
The PMRF can readily be estimated from cross-sectional data, in which case it can be reasonably assumed that all cases or rows in the data set — which usually represent people — are independent. This assumption is violated, however, in panel data and time-series designs, in which an individual case is not a person but, rather, a single measurement moment of one of the persons in the sample. In this case, violations of independence occur in two ways: temporal dependencies are introduced owing to the temporal aspect of data gathering (for example, a person who feels sad at 12:00 might still feel sad at 15:00), and responses from the same person may correlate more strongly with one another than responses between different persons (for example, a person might feel, on average, very sad in all responses). Thus, whereas cross-sectional data can use independence assumptions that allow for the application of population-sample logic, time-series data require a model to deal with the dependence between data points.
To address time dependencies, PMRFs may be extended with temporal effects that represent regressions on the previous time point in a single-person case. These temporal effects may, for instance, be estimated through the application of a vector autoregressive model. The structure of the associations that remain after taking temporal effects into account can also be represented in a PMRF. This network is typically designated as the contemporaneous network . Thus, in contrast to the case of cross-sectional networks, the application of network modelling to multivariate time series returns separate network structures to characterize the dependence relation describing associations that link variables through time, and associations that link variables after these temporal effects have been taken into account. These networks have a distinct function in the interpretation of results. The temporal network can be read in terms of carry-over effects at the timescale defined by the spacing between repeated measures, where the temporal ordering can also assist causal interpretation. The contemporaneous network will include associations that are due to effects that occur at different timescales rather than those defined by the spacing between repeated measurements. Note that, just as cross-sectional networks, time-series networks almost always represent correlational data; interpretation of such networks in causal terms is never straightforward.
In panel data or N >1 time-series settings, multilevel modelling can differentiate between within-person and between-person variance In addition to the temporal and contemporaneous networks (both of which represent within-person information), one then obtains a third structure of associations that can be characterized as a PMRF. This third structure represents the conditional associations between the long-term averages of the time series between people. This structure, similar to that of cross-sectional networks, represents associations driven by individual differences and is known as the between-persons network. Thus, in the cross-sectional case one obtains one network (the PMRF of the association between individual differences), in the time-series case one obtains two networks (the directed temporal network of vector autoregressive coefficients and the undirected contemporaneous network of the regression residuals) and for multiple time series and panel data one obtains three networks (temporal and contemporaneous networks driven by intra-individual processes and the between-persons network driven by individual differences). In addition, one may use multiple time series to identify network structures that are (in)variant over individuals 58 or that define subgroups 59 .
Edge selection
Methods of edge selection are based on general statistical theory as applied to the estimation of conditional associations. Three methods are featured in the literature. First, approaches based on model selection through fit indices can be used. For example, regularized estimation procedures 16 , 33 lead to models that balance parsimony and fit, in the sense that they aim to only include edges that improve the fit of the network model to data (for instance, by minimizing the extended Bayesian information criterion 35 ). Second, null hypothesis testing procedures are used to evaluate each individual edge for statistical significance 30 ; if desired, this process can be specialized to deal with multiple testing, through Bonferroni correction or false discovery rate approaches, for example. Last, cross-validation approaches can be used. In these approaches, the network model is chosen based on its performance in out of sample prediction, such as in k -fold cross-validation 31 .
Network description
Once a network structure is estimated, network description tools from network science can be applied to investigate the topology of PMRF networks 3 , 60 .
Global topologies that are particularly important revolve around the distinction between sparse versus dense networks. In sparse networks, few (if any) edges are present relative to the total number of possible edges. In dense networks, the converse holds, and relatively many edges are present. This distinction is important for two reasons. First, optimal estimation procedures may depend on sparsity, for example regularization-based approaches can be expected to perform well if data are generated from a sparse network, but may not work well in dense networks. Second, in sparse networks the importance of individual nodes is typically more pronounced, because in dense networks all nodes tend to feature a similar large number of edges. Further analyses can be used to investigate the global topology of the network structure in greater detail; for example, Dalege et al. 61 investigate small-world features 9 of attitude networks and Blanken et al. 62 use clique percolation methodology to assess the structure of psychopathology networks. Although network visualizations are typically based on aesthetic principles — for example, by using force-based algorithms 63 — recently, techniques have been proposed to visualize networks based on multidimensional scaling 64 . These techniques allow node placement to mirror the strength of conditional associations in the PRMF, so that more strongly connected nodes are placed in closer vicinity to each other.
Local topological properties of networks feature attributes of particular nodes or sets of nodes. For example, measures of centrality can be used to investigate the position of nodes in the network. The most commonly used centrality metrics are node strength, which sums the absolute edge weights of edges per node; closeness, which quantifies the distance between the node and all other nodes by averaging the shortest path lengths to all other nodes; and betweenness, which quantifies how often a node lies on the shortest path connecting any two other nodes 65 . These metrics are directly adapted from social network analysis, and can be used to assess the position of variables in the network representation constructed by the researchers. Strength conveys how strongly the relevant variable is conditionally associated with other variables in the network, on average. However, note that closeness and betweenness treat association as distances, which can be problematic. More recently, new measures have been introduced, specifically designed for the analysis of PMRF structures. Expected influence is a measure of centrality that takes the sign of edge weights into account 66 ; this can be appropriate when variables have a non-arbitrary coding, such as when the high values of all variables indicate more psychopathology. Predictability quantifies how much variance in a node is explained by its neighbours 54 , which can be used to assess the extent to which the network structure predicts node states. Further extensions to the characterization of networks and nodes in terms of network science involve participation coefficients, minimal spanning trees and clique percolation as proposed by Letina et al. 67 and Blanken et al. 62 . Finally, the shortest paths between nodes may yield insight into the strongest predictive pathways, and clustering in the network may yield insight into potential underlying unobserved causes and the dimensionality of the system 68 .
Applications
Although network approaches as discussed here draw on insights from statistics and network theory, the specific combination of techniques discussed in this paper has its roots in psychometric modelling in psychological contexts. This section discusses three areas in which this approach has been particularly successful. First, the domain of personality research, where network models have been applied to describe the interaction between stable behavioural patterns that characterize an individual. Second, the domain of attitude research, in which networks have been designed to model the interaction between attitude elements (feelings, thoughts and behaviours) to explain phenomena such as polarization . Last, the domain of mental health research, where networks have been used to represent disorders as systems of interacting symptoms and to represent key concepts such as vulnerability and resilience.
Personality research
Personality researchers are interested in examining the processes characterizing personality traits 69 . One type of these processes is motivational: research shows that traits such as conscientiousness or extraversion can be considered as means to achieve specific goals, for example getting tasks done and having fun, which have been identified as goals relevant for conscientiousness and extraversion, respectively 70 . Psychometric network analysis of personality traits and motivational goals combined offers a novel way to explore relations among relatively stable dispositions. Personality networks can represent personality at different levels of abstraction, from higher-order traits to facets to specific items. One could wonder which abstraction level should be preferred. The answer requires balancing simplicity and accuracy of predictions and of explanations. Focusing on a level that is too abstract might result in losing important details, whereas adding elements beyond necessary could result in noisy estimates and, thus, faulty conclusions. An approach that can help is out of sample predictability 71 . We illustrate this by reanalysing data from Costantini et al. 41 (Study 3) that include 9 goals identified as relevant for conscientiousness and 30 items from an adjective-based measure of conscientiousness that assess three main facets: industriousness, impulse control and orderliness 44 .
Data and analysis
In this sample ( N = 432) we explored how well we could predict goals using a tenfold cross-validation approach 72 . The networks depicted in Fig. 4 represent Gaussian graphical models estimated with the qgraph R package 15 , using graphical lasso regularization. The lambda parameter for graphical lasso was selected through the extended Bayesian information criterion (γ = 0.5 (ref. 33 )). We varied the level of representation of the personality dimensions from general (single trait) to specific (3 facets) to molecular (30 items) and explored the relationships between personality and 9 goal scores.
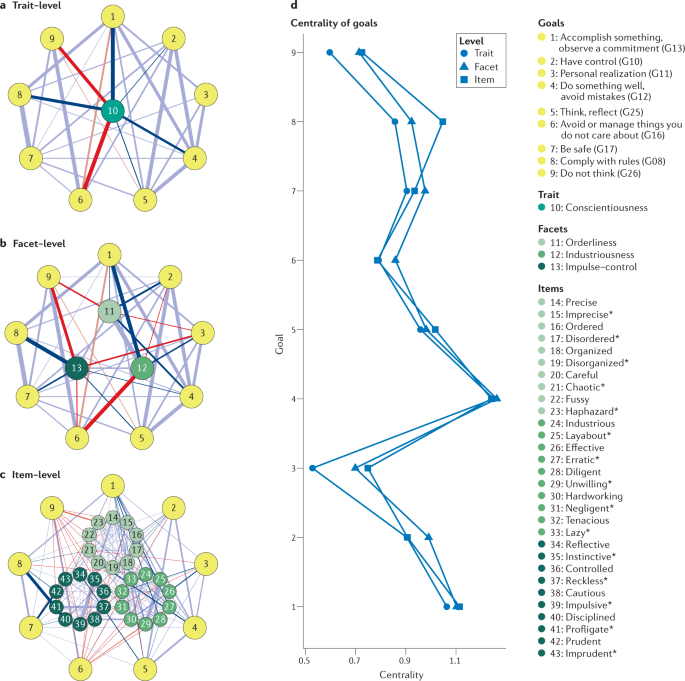
Network of relationships between motivational goals (yellow) and conscientiousness at the level of the trait (panel a ), its facets (panel b ) and items (panel c ). Blue edges represent positive connections and red edges represent negative connections; thicker edges represent stronger relationships. Relationships between personality and goals are emphasized with saturated colours. *Items reverse-scored before entering network estimation. d | Strength centrality for each goal in each network.
The results depicted in Fig. 4a suggest that some goals are positively associated and some negatively associated with an overall conscientiousness score. Two goals, personal realization (node 3) and be safe (node 7), do not show direct connections to the trait. However, this network does not consider several ways in which one can be conscientious. Some people can be more organized, others can be more controlled and yet others can be more industrious 43 . The facet-level network (Fig. 4b ) shows that most goals are related to a specific subset of one or two of the three facets, thus characterizing more clearly specific portions of the trait. At this level, personal realization (node 3) is positively related to industriousness but negatively connected to the remaining facets, something that would not have been apparent had we considered the trait level exclusively. At the item level (Fig. 4c ), connections appear generally consistent with those emerging at the facet level, albeit with some exceptions. For example, avoid or manage things you do not care about (node 6) shows relations with items of orderliness, whereas no such connection emerged at the facet level.
Figure 4d shows strength centrality estimates for all nodes in the three networks. Irrespective of the abstraction level considered, the most central goal was do something well, avoid mistakes (node 4). The centrality of node 4 is due to connections to other goals, rather than to its connections to conscientiousness. Such connections suggest that node 4 might serve as a means for several other goals. For example, one could speculate that doing things well might be important in the pursuit of more abstract goals, such as personal realization (node 3) or having control (node 2) (see ref. 72 for a discussion of the abstractness of these goals).
Results show that the trait level is never the best level for prediction and that some goals are best predicted at the item level and others at the facet level (Table 1 ), albeit in one case (goal 16) the trait level performed better than the item level. In general, specific levels might be useful if one is mainly interested in examining which elements of the personality system drive the association with a criterion 73 or if one is purely interested in prediction. In our example, the item level performed, on average, slightly better than the facet level in terms of prediction, although this was not the case for all goals (see also ref. 74 ). A preference for more abstract levels sometimes amounts to sacrificing a small portion of prediction in exchange for a noticeable gain in theoretical simplicity. Furthermore, using abstract predictors can sometimes assuage multicollinearity. At the same time, abstracting too much can lump together concepts that are better understood separately. There is no ultimate answer to the selection of the best abstraction level in personality as it heavily depends on the questions being asked and the data available. In general, the facet level might often provide a good balance between specificity and simplicity 75 , 76 .
Attitude research
Social psychologists are interested in how beliefs and attitudes can change over time. We illustrate the use of networks to improve our understanding of these processes with a study of attitudes towards Bill Clinton in the United States in the early 1990s. Based on the network theory of attitudes (Box 2 ) one expects that temperature should decrease throughout the years, because Bill Clinton was probably more on individuals’ minds when he was president than before he was president. We investigate changes in the network structure of these attitudes in the years before and during his presidency and whether the temperature of the attitude network changes. In this example, we estimate temperature using variations in how strongly correlated the attitude elements are at the different time points. Temperature of attitude networks can, however, also be measured by several proxies, such as how much attention individuals direct towards a given issue and how important they judge the issue.
We use data from the open access repository of the ANES between 1992 and 1996 including beliefs and emotions towards Bill Clinton. For this example, the presented data have been previously reported 77 , 78 . Beliefs were assessed using a four-point scale ranging from describes Bill Clinton extremely well to not at all. Emotions were assessed using a dichotomous scale with answer options of yes, have felt and no, never felt. Dichotomizing the belief questions, we fit an Ising model with increasing constraints representing their hypotheses to this longitudinal assessment of beliefs and emotions in the American electorate. We investigate the impact on the fit of the model of constraining edges between nodes to be equal across time points, constraining the external fields to be equal across time points and constraining the temperature (the entropy of the system) to be equal across time points. Additionally, we tested whether a dense network (all nodes are connected) or a sparse network (at least some edges are absent) fits the data best. After estimating the network, we applied the walktrap algorithm to the network to detect different communities, such as, for example, sets of highly interconnected nodes 68 , 79 . The walktrap algorithm makes use of random walks to detect communities. If random walks between two nodes are sufficiently short, these two nodes are assigned to the same community.
The results show a sparse network with a stable network structure, where edges do not differ between time points (Fig. 5 ). The model with varying external information and temperature fitted the data best. Figure 5a shows the estimated network at the four time points. The attitude network is connected: every attitude element is at least indirectly connected to every other attitude element. As can be seen, negative emotions of feeling afraid and angry are strongly connected to each other, as are positive emotions of feeling hope and pride. Within the beliefs, believing that Bill Clinton gets things done and provides strong leadership are closely connected. The belief that he cares about people is closely connected to the positive emotions. The walktrap algorithm detected two communities: one large community that contains all beliefs and the positive emotions; and one smaller community that contains the negative emotions. This indicates that positive emotions are more closely related to (positive) beliefs than positive and negative emotions are related to each other.
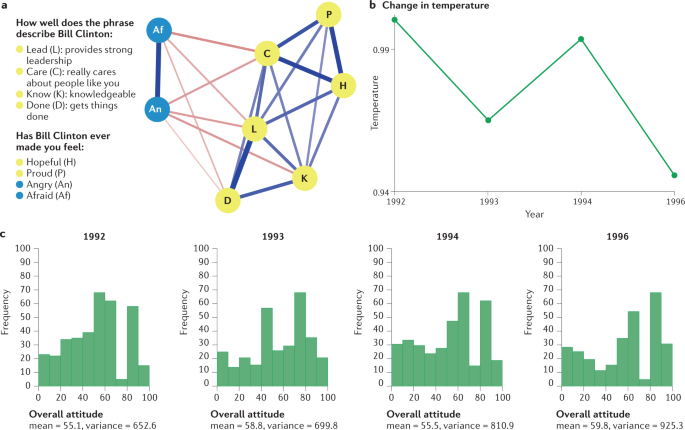
a | Estimated attitude network towards Bill Clinton. Colour of nodes corresponds to communities detected by the walktrap algorithm. Blue edges indicate positive connections between attitude elements and red edges indicate negative connections; width of the edges corresponds to strength of connection. b | Change in temperature throughout time. c | Histograms for overall attitude towards Bill Clinton in each year.
Figure 5b shows changes in temperature throughout the years. As can be expected from the network theory of attitudes (Box 2 ), the temperature of the attitude network generally decreased throughout the years, with the sharpest drop before the election in 1996 revealing an increase in the specificity of respondents’ attitudes towards Clinton. This implies that attitude elements became more consistent over time, resulting in more polarized attitudes. The increase in temperature between 1993 and 1994, however, is somewhat surprising.
Figure 5c shows the distribution of the overall attitude, separately measured on a scale ranging from 0 to 100, with higher numbers indicating more favourable attitudes. Based on the decreasing temperature of the attitude networks, a corresponding increase in the extremity of these distributions is to be expected. This is exactly what was found; the variance of the distributions increased in a somewhat similar fashion as the temperature of the attitude network decreased. The increase in the variance between 1993 and 1994 was the only exception.
Mental health research
Mental health research and practice rest on reportable symptoms and observable signs. Therapists interviewing patients will ask questions about subjective symptoms as well as assess signs of behavioural distress (such as agitated hand-wringing and crying). The challenge for both mental health researchers and therapists is to determine the cause of the person’s constellation of signs and symptoms. Therapists, moreover, have the additional charge of using this information to devise an appropriate course of treatment. The network theory of psychopathology 80 , 81 suggests that mental disorders are best understood as clusters of symptoms sufficiently unified by causal relations among those symptoms that support induction, explanation, prediction and control 82 , 83 (Box 3 ). Signs and symptoms are constitutive of disorder, not the result of an unobservable common cause. We illustrate this with an example study of social interaction and its relations to mental health variables in a student sample during the COVID-19 pandemic.
Box 3 Disease models versus network structures in mental health
Symptoms and signs associated with mental illness do not co-occur randomly. For example, recurrent obsessive thoughts about potential contamination co-occur more often with compulsive handwashing than with paranoid delusions. The tendency for some symptoms to co-occur may be owing to a common underlying cause. For example, consider a patient complaining of fatigue, pain upon swallowing, a fever and white patches in the throat. A physician may posit the Streptococcus bacterium as the common cause of the co-occurrence of the patient’s signs and symptoms 86 , 87 , and can eliminate the patient’s illness by therapeutically targeting the bacteria rather than the resulting symptoms. This bacterial model of disease became firmly entrenched early in psychiatry’s history, shaping the field’s methods and motivating researchers to identify the common underlying cause of regularly co-occurring signs and symptoms 81 (see the figure, part a ). Despite the widespread and often implicit influence of the bacterial model of disease, failures to discover biomarkers of putative underlying entities have continued to mount during the past century 146 . The network theory of psychopathology provides an alternative account of why some symptoms tend to co-occur 37 , 80 . Rather than being the independent, functionally unrelated consequences of an underlying common cause, the network theory of psychopathology posits that symptoms co-occur owing to causal interactions among the signs and symptoms themselves 81 , 147 (see the figure, part b ). Indeed, the Diagnostic and Statistical Manual of Mental Disorders criteria often specify functional relations among symptoms. For example, compulsive rituals diminish the distress provoked by obsessions and avoidance behaviour in panic disorder arises as a consequence of recurrent panic attacks. This simple idea forms the foundation of the network approach to psychopathology and motivates the effort to investigate the structure of relationships among symptoms using psychometric network analysis.
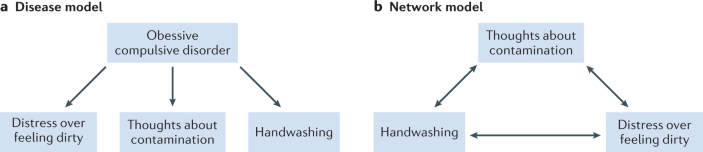
Researchers have devised an ecological momentary assessment study following 80 students (mean age = 20.38 years, standard deviation = 3.68, range = 18–48 years; n = 60 female, n = 19 male, n = 1 other) from Leiden University for 2 weeks in their daily lives 50 . With 19 different nationalities represented, this sample is highly international. Most students are single ( n = 50), one–third of the students are currently employed and about 1 in 5 students report prior mental health problems. In this study, participants are asked about the extent of their worry, sadness, irritability and other subjective phenomenological experiences four times per day via a smartphone application. We use multilevel vector autoregressive modelling to assess the contemporaneous and temporal associations among problems related to generalized anxiety and depression. As a reminder, the contemporaneous network covers relations within the same 3-h assessment window, and the temporal network lag – 1 relations between one 3-h window and the next.
The resulting networks can be used to inform our understanding of how the modelled variables evolve over time (Fig. 6 ). In this application, the model suggests that the cognitive symptom worry and the affective symptom nervous exhibit a strong contemporaneous association but do not exhibit a conditional dependence relation in temporal analyses, indicating that the relation between these items may be limited to a 3-h time interval. Similarly, we can clarify the paths by which external factors, such as social interaction, predict and are predicted by mental health. For example, the contemporaneous association between offline social interaction (nodes 8) and worry (node 3) occurs via feelings of loneliness (node 7), information which could be used in the generation of hypotheses about the causal relationships among these symptoms. It is also notable that different types of social interaction are differentially associated with loneliness. Offline social interaction is conditionally associated with lower levels of loneliness, whereas online social interaction is associated with higher levels of loneliness. The temporal associations further inform our understanding of these relationships. Difficulty envisioning the future and difficulty relaxing predict online social interaction, and online social interaction predicts subsequent difficulty relaxing. This illustrates how psychometric network analysis of time series naturally leads to more detailed hypotheses about the system under study; do note that this use of network analysis is exploratory and that generated hypotheses require independent testing, ideally through research that utilizes experimental interventions.
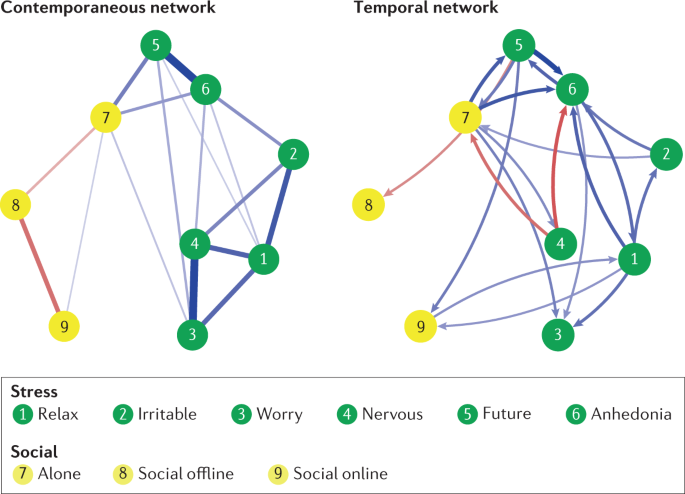
Contemporaneous network (left) of conditional associations between variables obtained after controlling for temporal effects in the temporal network (right); latter represents carry-over effects from one time point to the next. Blue edges indicate positive connections and red edges indicate negative connections; width of edges corresponds to strength of connection.
Network analyses not only equip researchers to investigate the associations among symptoms but also provide a novel framework for conceptualizing treatment. There are at least two potential ways one can intervene on a system, such as that depicted in Fig. 6 . First, we can lower the mean level of a node by diminishing its frequency or severity. For example, we could intervene on the online social interaction node, hoping, based on the contemporaneous relations, that it might promote offline social interaction, alleviate loneliness and, in turn, foster less worry, more optimism and greater interest and pleasure. However, even if initially successful, merely intervening on a node may be insufficient, leaving the person vulnerable to relapse, as the structure of the network remains intact. If pessimism and an inability to relax are, indeed, encouraging online social interaction, then when our intervention on this node ceases, the problem may return, erasing our treatment gains. Accordingly, instead of targeting a specific node (or symptom), we may target the link between symptoms, thereby changing the structure of the network. For example, rather than aiming to reduce online social interaction in general, we could specifically target the tendency to engage in online social interaction when the person experiences pessimism or difficulty relaxing, thereby eliminating the temporal association between these symptoms and online social interaction and disrupting the network.
Reproducibility and data deposition
A challenge posed by the estimation of PMRFs from multivariate data is that estimation error and sampling variation need to be taken into account when interpreting the network model. For example, networks estimated from two different groups of people may look different visually but this difference may be due to sampling variation. Several statistical methods have been proposed for assessing the stability and accuracy of estimated parameters as well as to compare network models of different groups. For many statistical estimators, data resampling techniques such as bootstrapping and permutation tests have been developed for this purpose 17 , 84 .
Standard approaches to robustness analyses involve three targets: individual edge weight estimates, differences between edges in the network and topological metrics defined on the network structure, such as node centrality. The robustness of edge weight estimates can be assessed by constructing intervals that reflect the sensitivity of edge weight estimates to sampling error, such as confidence intervals, credibility intervals and bootstrapped intervals (Fig. 7a ). The robustness of differences between edge weights can be assessed by investigating to what degree the bootstrapped intervals for the relevant coefficients overlap (Fig. 7b ). The robustness of network properties such as node centrality can be investigated through a case-dropping bootstrap, in which progressively fewer cases are sampled from the original data set to obtain subsamples; the correlation between centrality measures in these subsamples and the total sample is plotted as a function of the size of the subsamples (Fig. 7c ). Various approaches are available to assess these forms of robustness, including approaches based on bootstrapping 17 and Bayesian statistics 85 .
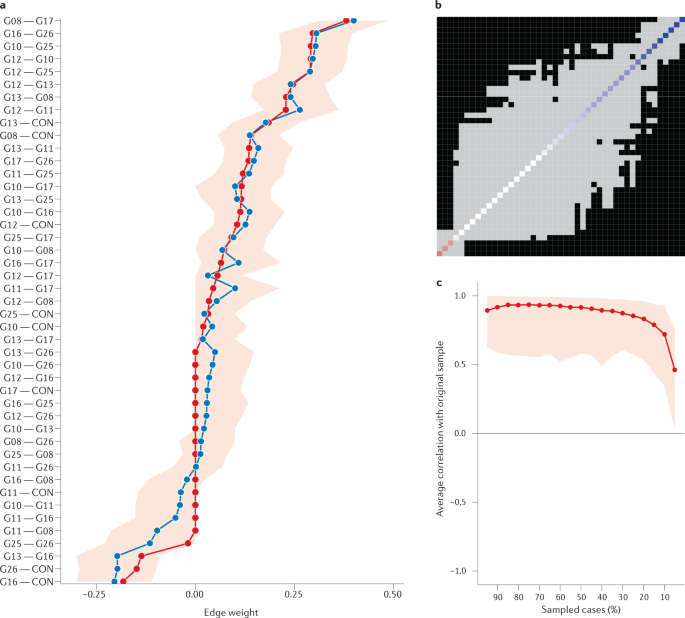
a | Sample value (red line), bootstrapped 95% intervals (shaded area) and average bootstrapped value (blue line) of edge weights. b | Whether the 95% bootstrapped interval of the differences between any two edges includes the value zero (grey squares) or not (dark squares) gives an indication of whether two edges are different from each other 17 . Diagonal visualizes magnitude of original edge; red indicates negative values, blue indicates positive values and colour saturation indicates absolute values (more saturated the colour, stronger the edge). c | Results of case-dropping bootstrap analysis showing average correlation between strength centrality estimated in the full sample and strength estimated on a random subsample, retaining only a certain portion of cases (from 90% to 10%). Shaded area indicates 95% bootstrapped confidence intervals of correlation estimates. Higher values indicate better stability of centrality estimates 17 .
The generalizability of network structures can be assessed by comparing results in different samples. This is typically assessed by examining the similarity of network structures across samples. A formal test for the invariance of networks has been developed to assess the null hypothesis that the networks are identical at the level of the population from which individuals have been sampled 84 and Bayesian analyses 86 can also be used to assess invariance of networks. Finally, moderated network analysis 87 and multi-group analysis have been introduced as methods for statistically comparing groups 88 . To gain more insight into the degree to which pairwise associations correspond across networks, the correlation between edge weights in different groups can be inspected.
It should be emphasized that, owing to sampling variability, one should not ordinarily expect to reproduce the network completely, and that the degree to which the network structure replicates depends on several factors, including the network architecture itself 80 , 89 . For this reason, network analysts have developed tools to compute the expected reproducibility of network structure estimation results 27 . Figure 8 displays the expected replicability of one of the personality networks reported above that one should expect, if the estimated networks were the true networks, using different sample sizes. For instance, from this analysis it is apparent that the item-level network should be expected to replicate less strongly than the facet-level and trait-level networks.
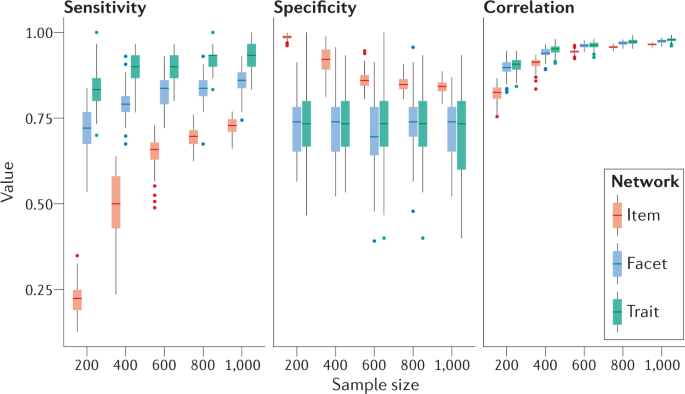
ReplicationSimulator generates multiple data sets from an estimated network to assess expected sensitivity (probability of including edges given that they are, in fact, present in the generating network) and specificity (probability of leaving out edges given that they are, in fact, absent in the generating network) as well as expected correlation between edge weights for two replication data sets generated from the network.
In addition to sampling variability, network structures can be affected by random measurement error. The effects of measurement error differ depending on the type of network estimated. In cross-sectional networks, ignoring measurement error typically leads to an underestimation of network density. If the strength of edges is associated with the network structure itself, this may lead to an artificial magnification of network structure. In longitudinal and time-series networks, however, measurement error can also lead to spurious edges 90 . One way to deal with measurement error is to utilize latent variable modelling; in this case, the network model is augmented with a measurement model that relates multiple observables to a single latent node, and the PMRF is estimated at the level of these latent nodes 27 .
To improve standardization and reproducibility, recent research explicates minimal shared norms in reporting psychological network analyses 91 . For methods sections of scientific papers, such norms include information on subsample and variable selection, the presence of deterministic relations between variables and skip structures that may distort the network, the estimation methods used as well as any additional specifications (such as thresholding, regularization, parameter settings), how the accuracy and stability of edge estimates were assessed and, finally, the statistical software and packages used, including their versions (Table 2 ).
In terms of results, current norms recommend reporting the final sample size after handling missing data, plotting and visualization choices and the accuracy and stability checks of any network model, in light of the research question of the researcher. If the research questions concern centrality estimates, case-drop bootstrap results would be reported, for example. Many reporting routines are dependent on the specific research goals of the researcher and different analysis routines result in different reporting choices. Burger et al. 91 elaborate on these routines and further discuss important considerations for network analysis and potential sources of misinterpretation of network structures.
Limitations and optimizations
Network structure estimation.
Although many network structures are now estimable through standard software, some limitations still remain. First, although treatments of dichotomous, unordered categorical and continuous data and their combinations are well developed 57 , treatments of ordinal data are still suboptimal. Ongoing research is developing approaches for such data, which are common in the social sciences 92 , 93 . Second, estimation routines have traditionally used nodewise regularized regression 16 or the graphical lasso 33 . Although these techniques return visually attractive networks, statistically they are most appropriate when networks can be expected to be sparse 35 , 36 . Non-regularized estimation approaches based on model selection provide an important alternative, as research suggests that they can outperform regularized approaches in several situations 94 , 95 . Third, many network modelling techniques handle missing data suboptimally, for example through list-wise deletion. Emerging estimation frameworks use alternative approaches, which allow for better missing data handling, for instance through full-information maximum likelihood 88 , 96 .
Interpretation
The fact that, in psychometric network models, edges are not observed but estimated necessitates the evaluation of sampling variance, which requires extensions. First, current techniques for edge selection do not guarantee that unselected edges are statistically indistinguishable from zero or that evidence for their absence is strong. Relatedly, many current estimation methods do not produce standard errors or confidence intervals around edge weight estimates, as the sampling distributions of regularized regression coefficients are unwieldy. This limits the interpretation of individual edges. In non-regularized networks, significance tests can be used, but this practice is not based on model selection and therefore inherits problems inherent in significance testing. New Bayesian approaches address these challenges, as they can quantify evidence for or against edge inclusion 97 .
Second, network structures depend on which variables are included. Nodes that are highly central in one network may therefore be peripheral in another. In addition, if important nodes are missing, this can affect the structure of the network; for instance, it may lead to increased edge strengths among nodes that represent effects of an omitted common cause 98 . If nodes are essentially duplicates of each other — for example, if two nodes have topological overlap — this will influence the network architecture as well 99 , 100 . Thus, network interpretation depends on a judicious choice of which variables to include in the network, and more research is needed to develop theoretical frameworks to guide these choices.
Third, centrality metrics have been suggested to reflect the importance of nodes to the system that the network represents 33 and early literature interpreted nodes with high centrality as more plausible targets for intervention 101 . However, recent work has highlighted situations where centrality is not a good proxy for causal influence 102 , 103 , and for certain networks, peripheral nodes may be more important in determining system behaviour 104 . In addition, in some areas such as psychopathology, interactions may occur at different timescales, which complicates the relation between association structure and causal dynamics. This has rendered the use of centrality measures a topic of debate, with some papers arguing that, because psychometric network models do not specify dynamics or flow, centrality metrics should not be interpreted in terms of causal dynamics at all. In addition, centrality metrics that concatenate paths between nodes (such as closeness and betweenness) are based on (absolute) conditional associations; these do not represent physical distances — they violate transitivity — and should not be interpreted as such. Finally, although network software indexes many types of centrality, including closeness, betweenness, degree, strength, eigenvalue and expected influence, there are no clear guidelines on which interpretations are licensed by each of these 105 , so more research is needed to investigate the relation between theoretical properties of possible generating models and empirical estimates of centrality 106 .
Causal inference
The constituent parts of PMRFs are purely statistical associations, so that direct causal inference based on network structures is not justified. Although the PMRF itself is typically unique — there are no alternative PMRFs that will generate the same set of joint probability distributions — the correspondence between the PMRF and generative causal systems is one to many: edges between nodes may arise owing to directed causal effects or feedback loops, but also owing to unobserved common causes 107 , conditioning on common effects 102 , 108 and various other structures (Fig. 9 ). As is the case for causal inference in general, causal inference based on PMRFs requires the statistical structure to be augmented by substantively backed assumptions 53 . This motivates the articulation of strong network theories in addition to the development of network models, as for instance have been devised for intelligence 109 , 110 , attitudes 61 , 111 and certain mental disorders 112 .
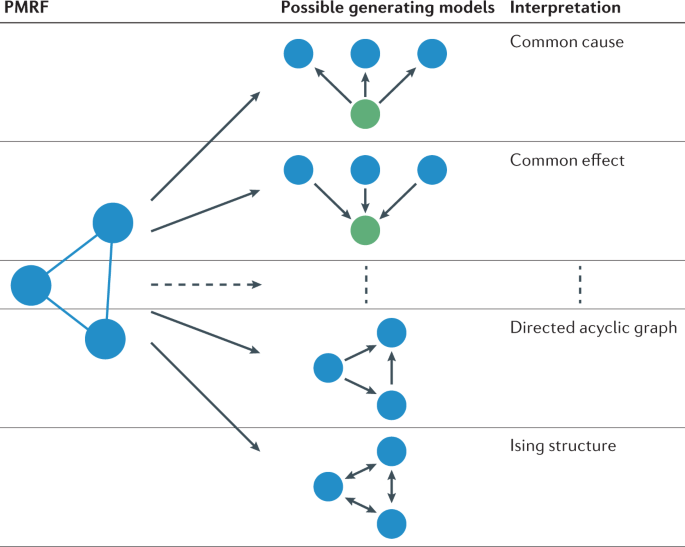
Pairwise Markov random field (PMRF) (left) can be generated by alternative models (middle) that have different interpretations (right). Dashed lines represent range of models and interpretations not captured here.
Current directions in network estimation may assist in causal inference by developing better methodologies. For example, causal search algorithms may be effective in identifying a particular causal model in certain cases 18 , 113 , 114 , 115 . In addition, inclusion of interventions in network structures may facilitate causal interpretation 25 , 116 , 117 . Alternatively, researchers may revert to non-causal interpretation of network structures. In such cases, marginal associations can be preferred over conditional associations if the goal is purely to describe the patterns of association. For example, Schwaba et al. 118 opted to model a network of correlations rather than partial correlations, because of the descriptive nature of their goal.
Confirmatory testing
Most applications of network analysis use exploratory techniques to estimate network structures 20 . However, advances in network estimation allow one to constrain parameters (such as edge weights) to a specific value, constrain edges to have the same edge weight as each other or constrain edge weights to be equal across different groups 88 , 119 . The ability to test these constraints adds confirmatory data analysis approaches to the network analytic toolbox 120 . The psychonetrics R package 121 is an example of an implementation that allows for confirmatory testing of network constraints. There are also Bayesian implementations available for testing constraints in networks that can be used to test whether an edge is positive, negative or null, and to test order constraints on edge weights 85 .
One way of arriving at network hypotheses is on the basis of exploratory network analyses. For example, an initial data set may be used to estimate a network model exploratively. In the next step, all of the estimated zeros are included as constraints in a network model that is fitted to a new data set 122 . Similarly, one can use an exploratively estimated network to formulate different hypotheses about the order of the strengths of edge weights and test these hypotheses against each other using Bayes factors 123 . A second way of arriving at network hypotheses is from substantive theory about the phenomena being modelled, from which network structures implied by the theory can be deduced 124 . To test substantive hypotheses, future methodological research should provide tools that can help researchers express substantive hypotheses in constraints on network structures, which can subsequently be tested using confirmatory models.
Network models are suited to estimate and represent patterns of conditional associations without requiring strong a priori assumptions on the generating model, which renders them well suited to exploratory data analysis and visualization of dependency patterns in multivariate data. As statistical analysis methods, the software routines for estimating, visualizing and analysing networks enhance existing exploratory data analysis methods, as they focus specifically on the patterns of pairwise conditional associations between variables. The resulting network representation of conditional associations between variables, as encoded in the PMRF, may be of interest in its own right, but can also function as a gateway that allows the researcher to assess the plausibility of different generating models that may produce the relevant conditional associations. This assessment may include latent variable models 29 and directed acyclic graphs 115 in addition to explanations based on network theories 80 , 123 .
Because network models for multivariate data explicitly represent pairwise interactions between components in a system, they form a natural bridge from data analysis to theory formation based on network science principles 3 . In this respect, networks not only accommodate the multivariate architecture of systems but also offer a toolbox to develop formal theories of the dynamical processes that form and maintain them 61 , 124 . One successful example of such an approach is the mutualism model of intelligence 125 , which proposes an explanation of the positive correlations between intelligence tests based on network concepts. This explanation quantifies how the structure of the cognitive network impacts the dynamic processes taking place in it. This model has been extended to explain various empirical phenomena reported in the intelligence literature 126 , 127 . Similar developments have taken place in clinical psychology 112 , 128 and attitude research 78 , as featured in the current paper.
The combination of network representations in data analytics and theory formation is remarkably fruitful in forging connections between different fields and research programmes. One important connection is that between the study of inter-individual differences and intra-individual mechanisms. More than half a century ago, Cronbach famously diagnosed psychological science to be a deeply divided discipline 129 . With one camp of psychological scientists concerned with mechanistic explanations and another camp primarily focused on the study of individual differences, that dichotomy is still prevailing. Some argue that in order to overcome this division, psychological scientists should rethink their widespread practice of detaching statistical practice from substantive theory 130 , 131 , 132 . One reason for this detachment, however, has been the long-standing lack of an intuitive modelling framework that facilitates both theory construction and process-based computations and simulation, so that it can connect the two disciplines 129 . But this gap is exactly what makes network approaches fall on fertile soil. Networks readily accommodate the multivariate architecture of psychological systems and also offer a toolbox to develop formal theories of the dynamical processes that act on them. In this manner, models of intra-individual dynamics can serve as explanations of systems of inter-individual differences, bridging the gap between intra-individual and inter-individual modelling 129 .
Network models are not only useful to create bridges from data analysis to theory formation but also to connect different scientific disciplines to each other. In recent years, network science and associated complex systems approaches have led to an active interdisciplinary research area in which researchers from many fields collaborate. Network approaches in psychology, as discussed here, have similarly broadened the horizon of relevant candidate methodologies relevant to psychological research questions; for instance, it is remarkable that the first network model fitted to psychopathology data 16 was based on modelling approaches developed to study atomic spins 133 , 134 , whereas subsequent studies into the research dynamics of psychopathology 135 investigated sudden transitions using methodology developed in ecology 136 and, finally, recent studies of interventions in such networks are based on control theory 137 . Clearly, network representations create a situation where scientists with different disciplinary backgrounds find a common vocabulary.
This common vocabulary creates tantalizing possibilities for building bridges between research areas — particularly in cases where the systems studied are plausibly constituted by networks operating at different levels, such as human behaviour. For instance, largely independent of one another, neuroscience and psychology have both developed research traditions rooted in network science. With network models of the brain based on neuroimaging studies and network models of psychological responses, the bigger picture might no longer be obstructed by disciplinary fences 138 , 139 . This promise is by no means limited to psychology and its subdisciplines; the network fever is spanning many disciplines, such as physics, ecology and biology. In fact, the best cited network papers are concerned with universal network characteristics that can advance interdisciplinary theory and modelling 9 , 140 . We have only begun to chart the connections between disciplines that deal with complex networks, and we hope that network approaches to multivariate data can play a productive role in this respect.
Code availability
Code and data used in sample analyses are available from https://github.com/DennyBorsboom/NatureMethodsPrimer_NetworkAnalysis .
Change history
21 february 2022.
A Correction to this paper has been published: https://doi.org/10.1038/s43586-022-00101-1
Meadows, D. H. Thinking in Systems: A Primer (Chelsea Green, 2008). This text is the most convincing to motivate systems thinking throughout the sciences .
Barabási, A. L. The network takeover. Nat. Phys. 8 , 14–16 (2012).
Article Google Scholar
Newman, M. E. J. Networks: An Introduction (Oxford University Press, 2010). This text is an ideal introduction to network science and the associated mathematical modelling techniques .
Newman, M. E. J., Barabási, A. L. E. & Watts, D. J. The Structure and Dynamics of Networks (Princeton University Press, 2006).
Richens, R. H. Preprogramming for mechanical translation. Mech. Transl. Comput. Ling. 3 , 20–25 (1956).
Google Scholar
Milgram, S. The small world problem. Psychol. Today 2 , 60–67 (1967).
Ramón y Cajal, S. The Croonian Lecture: la fine structure des centres nerveux. Proc. R. Soc. Lond. 55 , 444–468 (1894).
Newman, M. E. & Clauset, A. Structure and inference in annotated networks. Nat.Commun. 7 , 1–11 (2016).
Watts, D. J. & Strogatz, S. H. Collective dynamics of ‘small-world’ networks. Nature 393 , 440–442 (1998). This article kickstarts the growth of network science in the past few decades .
Article ADS MATH Google Scholar
Bavelas, A. A mathematical model for group structures. Appl. Anthropol. 7 , 16–30 (1948).
Kolaczyk, E. D. Statistical Analysis of Network Data: Methods and Models (Springer, 2009). This text is an authoritative overview of statistical models for network analysis .
Cox, D. R. & Wermuth, N. Multivariate Dependencies: Models, Analysis and Interpretation Vol. 67 (CRC, 1996).
Pearl, J. Causality: Models, Reasoning, and Inference (Cambridge University Press, 2000). This crucial book makes the connection between conditional independence patterns and causal structures .
Wright, S. Correlation and causation. J. Agric. Res. 20 , 557–585 (1921).
Epskamp, S., Cramer, A. O., Waldorp, L. J., Schmittmann, V. D. & Borsboom, D. qgraph: network visualizations of relationships in psychometric data. J. Stat. Softw. 48 , 1–18 (2012).
Van Borkulo, C. D. et al. A new method for constructing networks from binary data. Sci. Rep. 4 , 1–10 (2014). This paper is the first application of regularized network modelling in psychopathology .
Epskamp, S., Borsboom, D. & Fried, E. I. Estimating psychological networks and their accuracy: a tutorial paper. Behav. Res. Methods 50 , 195–212 (2018). This article introduces robustness analysis for network modelling .
Spirtes, P., Glymour, C. N., Scheines, R. & Heckerman, D. Causation, prediction, and search (MIT Press, 2000).
Haslbeck, J., Ryan, O., Robinaugh, D., Waldorp, L. & Borsboom, D. Modeling psychopathology: from data models to formal theories. Psychol. Methods https://doi.org/10.31234/osf.io/jgm7f (2021).
Robinaugh, D. J., Hoekstra, R. H., Toner, E. R. & Borsboom, D. The network approach to psychopathology: a review of the literature 2008–2018 and an agenda for future research. Psychol. Med. 50 , 353–366 (2020).
Deserno, M. K., Borsboom, D., Begeer, S. & Geurts, H. M. Multicausal systems ask for multicausal approaches: a network perspective on subjective well-being in individuals with autism spectrum disorder. Autism 21 , 960–971 (2017).
Isvoranu, A. M. et al. Toward incorporating genetic risk scores into symptom networks of psychosis. Psychol. Med. 50 , 636–643 (2020).
Fried, E. et al. Using network analysis to examine links between individual depressive symptoms, inflammatory markers, and covariates. Psychol. Med. 16 , 2682–2690 (2019).
Isvoranu, A. M. et al. Extended network analysis: from psychopathology to chronic illness. BMC Psychiatry 21 , 1–9 (2021).
Blanken, T. F. et al. Introducing network intervention analysis to investigate sequential, symptom-specific treatment effects: a demonstration in co-occurring insomnia and depression. Psychother. Psychosom. 88 , 52–54 (2019).
Blanken, T. F., Borsboom, D., Penninx, B. W. & Van Someren, E. J. Network outcome analysis identifies difficulty initiating sleep as a primary target for prevention of depression: a 6-year prospective study. Sleep 43 , zsz288 (2020).
Epskamp, S. Psychometric network models from time series and panel data. Psychometrika 85 , 206–231 (2020). This article systematizes psychometric network models for longitudinal data .
Article MathSciNet MATH Google Scholar
Kindermann, R. P. & Snell, J. L. On the relation between Markov random fields and social networks. J. Math. Sociol. 7 , 1–13 (1980).
Marsman, M. et al. An introduction to network psychometrics: relating Ising network models to item response theory models. Multivar. Behav. Res. 53 , 15–35 (2018). This article establishes systematic links between network models and latent variable analysis .
Williams, D. R. & Rast, P. Back to the basics: rethinking partial correlation network methodology. Br. J. Math. Stat. Psychol. 73 , 187–212 (2020).
Haslbeck, J. M. & Waldorp, L. J. How well do network models predict observations? On the importance of predictability in network models. Behav. Res. Methods 50 , 853–861 (2018).
Christensen, A. P., Kenett, Y. N., Aste, T., Silvia, P. J. & Kwapil, T. R. Network structure of the Wisconsin Schizotypy Scales — short forms: examining psychometric network filtering approaches. Behav. Res. Methods 50 , 2531–2550 (2018).
Epskamp, S. & Fried, E. I. A tutorial on regularized partial correlation networks. Psychol. Med. 23 , 617 (2018).
Costantini, G. et al. Stability and variability of personality networks. A tutorial on recent developments in network psychometrics. Pers. Individ. Differ. 136 , 68–78 (2019).
Barber, R. F. & Drton, M. High-dimensional Ising model selection with Bayesian information criteria. Electron. J. Stat. 9 , 567–607 (2015).
Ravikumar, P., Wainwright, M. J., Raskutti, G. & Yu, B. High-dimensional covariance estimation by minimizing ℓ1-penalized log-determinant divergence. Electron. J. Stat. 5 , 935–980 (2011). This seminal article presents regularized estimation of network structure .
Borsboom, D. & Cramer, A. O. Network analysis: an integrative approach to the structure of psychopathology. Annu. Rev. Clin. Psychol. 9 , 91–121 (2013).
Frewen, P. A., Allen, S. L., Lanius, R. A. & Neufeld, R. W. Perceived causal relations: novel methodology for assessing client attributions about causal associations between variables including symptoms and functional impairment. Assessment 19 , 480–493 (2012).
Deserno, M. K. et al. Highways to happiness for autistic adults? Perceived causal relations among clinicians. PLoS ONE 15 , e0243298 (2020).
Robinaugh, D. J., LeBlanc, N. J., Vuletich, H. A. & McNally, R. J. Network analysis of persistent complex bereavement disorder in conjugally bereaved adults. J. Abnorm. Psychol. 123 , 510–522 (2014).
Costantini, G., Saraulli, D. & Perugini, M. Uncovering the motivational core of traits: the case of conscientiousness. Eur. J. Pers. 34 , 1073–1094 (2020).
Deserno, M. K., Borsboom, D., Begeer, S. & Geurts, H. M. Relating ASD symptoms to well-being: moving across different construct levels. Psychol. Med. 48 , 1179–1189 (2018).
Roberts, B. W., Lejuez, C., Krueger, R. F., Richards, J. M. & Hill, P. L. What is conscientiousness and how can it be assessed? Dev. Psychol. 50 , 1315–1330 (2014).
Costantini, G. et al. Development of indirect measures of conscientiousness: combining a facets approach and network analysis. Eur. J. Pers. 29 , 548–567 (2015).
Mõttus, R., Kandler, C., Bleidorn, W., Riemann, R. & McCrae, R. R. Personality traits below facets: the consensual validity, longitudinal stability, heritability, and utility of personality nuances. J. Pers. Soc. Psychol. 112 , 474–490 (2017).
Molenaar, P. C. A manifesto on psychology as idiographic science: bringing the person back into scientific psychology, this time forever. Measurement 2 , 201–218 (2004). This article establishes the need for time-series modelling of psychometric data .
Hamaker, E. L., Kuiper, R. M. & Grasman, R. P. A critique of the cross-lagged panel model. Psychol. Methods 20 , 102–116 (2015). This article demonstrates the need to separate between-subject from within-subject structures in the analysis of longitudinal data .
aan het Rot, M., Hogenelst, K. & Schoevers, R. A. Mood disorders in everyday life: a systematic review of experience sampling and ecological momentary assessment studies. Clin. Psychol. Rev. 32 , 510–523 (2012).
Moskowitz, D. S. & Young, S. N. Ecological momentary assessment: what it is and why it is a method of the future in clinical psychopharmacology. J. Psychiatry Neurosci. 31 , 13 (2006).
Fried, E. I., Papanikolaou, F. & Epskamp, S. (2021). Mental health and social contact during the COVID-19 pandemic: an ecological momentary assessment study. Clin. Psychol. Sci. https://doi.org/10.1177/21677026211017839 (2021).
Bringmann, L. F. et al. A network approach to psychopathology: new insights into clinical longitudinal data. PLoS ONE 8 , e60188 (2013). This article introduces multilevel time-series modelling in the context of psychopathology networks .
Article ADS Google Scholar
Hamaker, E. L., Ceulemans, E., Grasman, R. P. P. P. & Tuerlinckx, F. Modeling affect dynamics: state of the art and future challenges. Emot. Rev. 7 , 316–322 (2015).
Pearl, J. Causal inference. Causality: objectives and assessment. Proc. Mac. Learn. Res. 6 , 39–58 (2010).
Spirtes, P., Glymour, C. N., Scheines, R. & Heckerman, D. Causation, Prediction, and Search (MIT Press, 2000).
Chen, B., Pearl, J. & Kline, R. Graphical tools for linear path models. Psychometrika 4 , R432 (2018).
Roverato, A. & Castelo, R. The networked partial correlation and its application to the analysis of genetic interactions. J. R. Stat. Soc. 66 , 647–665 (2017).
Article MathSciNet Google Scholar
Haslbeck, J. & Waldorp, L. J. mgm: estimating time-varying mixed graphical models in high-dimensional data. Preprint at https://arxiv.org/abs/1510.06871 (2020). This article generalizes the network model to mixed data types .
Gates, K. M. & Molenaar, P. C. M. Group search algorithm recovers effective connectivity maps for individuals in homogeneous and heterogeneous samples. NeuroImage 63 , 310–319 (2012).
Gates, K. M., Lane, S. T., Varangis, E., Giovanello, K. & Guskiewicz, K. Unsupervised classification during time-series model building. Multivar. Behav. Res. 52 , 129–148 (2017).
Barabasi, A. L. Network Science (Cambridge University Press, 2018). This text is an authoritative overview of network science .
Dalege, J. et al. Toward a formalized account of attitudes: the causal attitude network (CAN) model. Psychol. Rev. 123 , 2 (2016).
Blanken, T. F. et al. The role of stabilizing and communicating symptoms given overlapping communities in psychopathology networks. Sci. Rep. 8 , 1–8 (2018).
Fruchterman, T. M. & Reingold, E. M. Graph drawing by force-directed placement. Software Pract. Exper. 21 , 1129–1164 (1991).
Jones, P. J., Mair, P. & McNally, R. J. Visualizing psychological networks: a tutorial in R. Front. Psychol. 9 , 1742 (2018).
Opsahl, T., Agneessens, F. & Skvoretz, J. Node centrality in weighted networks: generalizing degree and shortest paths. Soc. Netw. 32 , 245–251 (2010). This article generalizes network metrics to weighted networks as intensively used in current network approaches to multivariate data .
Robinaugh, D. J., Millner, A. J. & McNally, R. J. Identifying highly influential nodes in the complicated grief network. J. Abnorm. Psychol. 125 , 747 (2016).
Letina, S., Blanken, T. F., Deserno, M. K. & Borsboom, D. Expanding network analysis tools in psychological networks: minimal spanning trees, participation coefficients, and motif analysis applied to a network of 26 psychological attributes. Complexity https://doi.org/10.1155/2019/9424605 (2019).
Golino, H. F. & Epskamp, S. Exploratory graph analysis: a new approach for estimating the number of dimensions in psychological research. PLoS ONE 12 , e0174035 (2017).
Baumert, A. et al. Integrating personality structure, personality process, and personality development. Eur. J. Pers. 31 , 503–528 (2017).
McCabe, K. O. & Fleeson, W. Are traits useful? Explaining trait manifestations as tools in the pursuit of goals. J. Pers. Soc. Psychol. 110 , 287–301 (2016).
Yarkoni, T. & Westfall, J. Choosing prediction over explanation in psychology: lessons from machine learning. Perspect. Psychol. Sci. 12 , 1100–1122 (2017).
Costantini, G., Perugini, M. & Mõttus, R. A framework for testing causality in personality research. Eur. J. Pers. 32 , 254–268 (2018).
Mõttus, R. Towards more rigorous personality trait—outcome research. Eur. J. Pers. 30 , 292–303 (2016).
Mõttus, R. et al. Descriptive, predictive and explanatory personality research: different goals, different approaches, but a shared need to move beyond the Big Few traits. Eur. J. Pers. 34 , 1175–1201 (2020).
Paunonen, S. V. & Ashton, M. C. Big five factors and facets and the prediction of behavior. J. Pers. Soc. Psychol. 81 , 524 (2001).
Paunonen, S. V. & Ashton, M. C. On the prediction of academic performance with personality traits: a replication study. J. Res. Pers. 47 , 778–781 (2013).
Dalege, J., Borsboom, D., van Harreveld, F., Waldorp, L. J. & van der Maas, H. L. Network structure explains the impact of attitudes on voting decisions. Sci. Rep. 7 , 1–11 (2017).
Dalege, J., Borsboom, D., van Harreveld, F. & van der Maas, H. L. A network perspective on attitude strength: testing the connectivity hypothesis. Soc. Psychol. Personal. Sci. 10 , 746–756 (2019).
Pons, P. & Latapy, M. Computing communities in large networks using random walks. J. Graph. Algorithms Appl. 10 , 191–218 (2006).
Borsboom, D. A network theory of mental disorders. World Psychiatry 16 , 5–13 (2017).
McNally, R. Network analysis of psychopathology: controversies and challenges. Annu. Rev. Clin. Psychol. 17 , 31–53 (2020). This paper is a state-of-the-art overview of the status of network analysis in psychopathology .
Kendler, K. S., Zachar, P. & Craver, C. What kinds of things are psychiatric disorders? Psychol. Med. 41 , 1143–1150 (2011).
Held, B. S. The distinction between psychological kinds and natural kinds revisited: can updated natural-kind theory help clinical psychological science and beyond meet psychology’s philosophical challenges? Rev. Gen. Psychol. 21 , 82–94 (2017).
Van Borkulo, C. D., et al. Comparing network structures on three aspects: a permutation test (preprint). https://doi.org/10.13140/RG.2.2.29455.38569 (2017).
Williams, D. R. & Mulder, J. Bayesian hypothesis testing for Gaussian graphical models: conditional independence and order constraints. J. Math. Psychol. 99 , 102441 (2020). This article introduces Bayesian approaches to hypothesis testing in network models .
Williams, D. R., Piironen, J., Vehtari, A. & Rast, P. Bayesian estimation of Gaussian graphical models with predictive covariance selection. Preprint at https://arxiv.org/abs/1801.05725 (2018).
Haslbeck, J. M., Borsboom, D. & Waldorp, L. J. Moderated network models. Multivariate Behav. Res. 56 , 256–287 (2019).
Epskamp, S., Isvoranu, A. M. & Cheung, M. Meta-analytic Gaussian network aggregation. Psychometrika https://doi.org/10.1007/s11336-021-09764-3 (2021).
Article MATH Google Scholar
Williams, D. R. Learning to live with sampling variability: expected replicability in partial correlation networks. (preprint). PsyArXiv https://doi.org/10.31234/osf.io/fb4sa (2020).
Schuurman, N. K. & Hamaker, E. L. Measurement error and person-specific reliability in multilevel autoregressive modeling. Psych. Methods 24 , 70 (2019).
Burger, J. et al. Reporting standards for psychological network analyses in cross-sectional data. (preprint). PsyArXiv https://doi.org/10.31234/osf.io/4y9nz (2020).
Isvoranu, A. & Epskamp, S. Continuous and ordered categorical data in network psychometrics: which estimation method to choose? deriving guidelines for applied researchers. (preprint). PsyArXiv https://doi.org/10.31234/osf.io/mbycn (2021).
Johal, S. K. & Rhemtulla, M. Comparing estimation methods for psychometric networks with ordinal data. (preprint). PsyArXiv https://doi.org/10.31234/osf.io/ej2gn (2021).
Williams, D. R., Rhemtulla, M., Wysocki, A. C. & Rast, P. On nonregularized estimation of psychological networks. Multivariate Behav. Res. 54 , 719–750 (2019).
Wysocki, A. C. & Rhemtulla, M. On penalty parameter selection for estimating network models. Multivariate Behav. Res. 56 , 288–302 (2019).
Mansueto, A. C., Wiers, R., van Weert, J., Schouten, B. C. & Epskamp, S. Investigating the feasibility of idiographic network models. (preprint). PsyArXiv https://doi.org/10.31234/osf.io/hgcz6 (2020).
Williams, D. R., Briganti, G., Linkowski, P. & Mulder, J. On accepting the null hypothesis of conditional independence in partial correlation networks: a Bayesian analysis. (preprint). PsyArXiv https://doi.org/10.31234/osf.io/7uhx8 (2021).
Hallquist, M. N., Wright, A. G. & Molenaar, P. C. Problems with centrality measures in psychopathology symptom networks: why network psychometrics cannot escape psychometric theory. Multivariate Behav. Res. 56 , 199–223 (2019).
Christensen, A. P., Golino, H. & Silvia, P. J. A psychometric network perspective on the validity and validation of personality trait questionnaires. Eur. J. Pers. 34 , 1095–1108 (2020).
Fried, E. I. & Cramer, A. O. Moving forward: challenges and directions for psychopathological network theory and methodology. Perspect. Psychol. Sci. 12 , 999–1020 (2017).
Rhemtulla, M. et al. Network analysis of substance abuse and dependence symptoms. Drug Alcohol. Depend. 161 , 230–237 (2016).
Dablander, F. & Hinne, M. Node centrality measures are a poor substitute for causal inference. Sci. Rep. 9 , 1–13 (2019).
Spiller, T. R. et al. On the validity of the centrality hypothesis in cross-sectional between-subject networks of psychopathology. BMC Med. 18 , 1–14 (2020).
Quax, R., Apolloni, A. & Sloot, P. M. The diminishing role of hubs in dynamical processes on complex networks. J. R. Soc. Interface 10 , 20130568 (2013).
Bringmann, L. F. et al. What do centrality measures measure in psychological networks? J. Abnorm. Psychol. 128 , 892 (2019).
Borgatti, S. P. Centrality and network flow. Soc. Netw. 27 , 55–71 (2005).
Rohrer, J. M. Thinking clearly about correlations and causation: graphical causal models for observational data. Adv. Methods Pract. Psychol. Sci. 1 , 27–42 (2018).
de Ron, J., Fried, E. I. & Epskamp, S. Psychological networks in clinical populations: investigating the consequences of Berkson’s bias. Psychol. Med. 51 , 168–176 (2021).
Kan, K. J., van der Maas, H. L. & Levine, S. Z. Extending psychometric network analysis: empirical evidence against g in favor of mutualism? Intelligence 73 , 52–62 (2019).
Kievit, R. A. et al. Mutualistic coupling between vocabulary and reasoning supports cognitive development during late adolescence and early adulthood. Psychol. Sci. 28 , 1419–1431 (2017).
Dalege, J., Borsboom, D., van Harreveld, F. & van der Maas, H. L. The attitudinal entropy (AE) framework as a general theory of individual attitudes. Psychol. Inq. 29 , 175–193 (2018). This article develops the network theory of attitudes .
Robinaugh, D. et al. Advancing the network theory of mental disorders: a computational model of panic disorder. (preprint). PsyArXiv https://doi.org/10.31234/osf.io/km37w (2020). This article is the first to augment symptom network models with substantively plausible formalized theory .
Scutari, M. Learning Bayesian networks with the bnlearn R package. J. Stat. Softw. 35 , 1–22 (2010).
Colombo, D. & Maathuis, M. H. Order-independent constraint-based causal structure learning. J. Mach. Learn. Res. 15 , 3741–3782 (2014).
MathSciNet MATH Google Scholar
Ryan, O., Bringmann, L. F. & Schuurman, N. K. The challenge of generating causal hypotheses using network models. (preprint). PsyArXiv https://doi.org/10.31234/osf.io/ryg69 (2020).
Kossakowski, J. J., Gordijn, M. C. M., Harriette, R. & Waldorp, L. J. Applying a dynamical systems model and network theory to major depressive disorder. Front. Psychol. 10 , 1762 (2019).
Mooij, J. M., Magliacane, S. & Claassen, T. Joint causal inference from multiple contexts. J. Mach. Learn. Res. 21 , 1–108 (2020).
Schwaba, T., Rhemtulla, M., Hopwood, C. J. & Bleidorn, W. A facet atlas: visualizing networks that describe the blends, cores, and peripheries of personality structure. PLoS ONE 15 , e0236893 (2020).
Drton, M. & Richardson, T. S. in Proceedings of the 20th Conference on Uncertainty in Artificial Intelligence (UAI ‘04) 130–137 (AUAI Press, 2004).
Epskamp, S., Rhemtulla, M. & Borsboom, D. Generalized network psychometrics: combining network and latent variable models. Psychometrika 82 , 904–927 (2017).
Epskamp, S. Psychonetrics: structural equation modeling and confirmatory network analysis. Psychonetrics http://psychonetrics.org/ (2020).
Kan, K. J., de Jonge, H., van der Maas, H. L., Levine, S. Z. & Epskamp, S. How to compare psychometric factor and network models. J. Intell. 8 , 35 (2020).
Rodriguez, J. E., Williams, D. R., Rast, P. & Mulder, J. On formalizing theoretical expectations: Bayesian testing of central structures in psychological networks. (preprint). PsyArXiv https://doi.org/10.31234/osf.io/zw7pf (2020).
Cramer, A. O., Waldorp, L. J., Van Der Maas, H. L. & Borsboom, D. Comorbidity: a network perspective. Behav. Brain Sci. 33 , 137 (2010).
Van Der Maas, H. L. et al. A dynamical model of general intelligence: the positive manifold of intelligence by mutualism. Psychol. Rev. 113 , 842 (2006). This article contains the first articulation of a network model to account for patterns of individual differences in psychology .
Savi, A. O., Marsman, M., van der Maas, H. L. & Maris, G. K. The wiring of intelligence. Perspect. Psychol. Sci. 14 , 1034–1061 (2019).
Van Der Maas, H. L., Kan, K. J., Marsman, M. & Stevenson, C. E. Network models for cognitive development and intelligence. J. Intell. 5 , 16 (2017).
Cramer, A. O. et al. Major depression as a complex dynamic system. PloS ONE 11 , e0167490 (2019).
Cronbach, L. J. [1957]. The two disciplines of scientific psychology. Am. Psychol. 12 , 671 (2016).
Gigerenzer, G. Personal reflections on theory and psychology. Theor. Psychol. 20 , 733–743 (2010).
Wood, D., Gardner, M. H. & Harms, P. D. How functionalist and process approaches to behavior can explain trait covariation. Psychol. Rev. 122 , 84 (2015).
Borsboom, D., van der Maas, H. L., Dalege, J., Kievit, R. A. & Haig, B. D. Theory construction methodology: a practical framework for building theories in psychology. Perspect. Psychol. Sci. 16 , 756–766 (2021).
Lenz, W. Beitrag zum Verständnis der magnetischen Erscheinungen in festen Körpern [German]. Physikalische Z. 21 , 613–615 (1920).
Ising, E. Beitrag zur theorie des ferromagnetismus [German]. Z. für Phys. 31 , 253–258 (1925).
Wichers, M., Groot, P. C. & Psychosystems, E. S. M., EWS Group. Critical slowing down as a personalized early warning signal for depression. Psychother. Psychosom. 85 , 114–116 (2016). This article is the first to investigate early warnings in psychopathology transitions .
Scheffer, M. et al. Early-warning signals for critical transitions. Nature 461 , 53–59 (2009). This crucial article articulates the link between complex systems, sudden transitions and early warning signals in time series .
Henry, T. R., Robinaugh, D. & Fried, E. I. On the control of psychological networks. (preprint). PsyArXiv https://doi.org/10.31234/osf.io/7vpz2 (2021).
Brooks, D. et al. The multilayer network approach in the study of personality neuroscience. Brain Sci. 10 , 915 (2020).
Bathelt, J., Geurts, H. M. & Borsboom, D. More than the sum of its parts: merging network psychometrics and network neuroscience with application in autism. Preprint at bioRxiv https://doi.org/10.1101/2020.11.17.386276 (2020).
Liljeros, F., Edling, C. R., Amaral, L. A. N., Stanley, H. E. & Åberg, Y. The web of human sexual contacts. Nature 411 , 907–908 (2001).
R Core Team. R: A Language and Environment for Statistical Computing (R Foundation for Statistical Computing, 2020).
McCrae, R. R. & Costa, P. T. Jr. in Sage Handbook of Personality Theory and Assessment Vol. 1 273–294 (Sage, 2008).
Ashton, M. C. & Lee, K. Objections to the HEXACO model of personality structure—and why those objections fail. Eur. J. Pers. 34 , 492–510 (2020).
Cramer, A. O. J. et al. Dimensions of normal personality as networks in search of equilibrium: you can’t like parties if you don’t like people. Eur. J. Pers. 26 , 414–431 (2012).
Goldberg, L. R. et al. The international personality item pool and the future of public-domain personality measures. J. Res. Pers. 40 , 84–96 (2006).
McNally, R. J. What is Mental Illness? (Belknap Press of Harvard University Press, 2011).
Borsboom, D. Psychometric perspectives on diagnostic systems. J. Clin. Psychol. 64 , 1089–1108 (2008).
Download references
Acknowledgements
D.J.R.’s work on this manuscript was supported by a National Institute of Mental Health (NIMH) Career Development Award (K23-MH113805). M.K.D.’s work was supported by a Rubicon fellowship of the Netherlands Organization for Scientific Research (NWO) (no. 019.191SG.005). D.B.’s work was supported by European Research Council Consolidator Grant 647209. M.P. and G.C.’s work was supported by European Union’s Horizon 2020 research and innovation programme (grant no. 952464). E.I.F. is supported by funding from the European Research Council (ERC) under the European Union’s Horizon 2020 research and innovation programme (grant no. 949059). S.E. is supported by NWO Veni (grant number 016-195-261). C.D.v.B.’s work was supported by European Research Council Consolidator Grant 647209, granted to D.B. J.D.’s work was supported by an EU Horizon 2020 Marie Curie Global Fellowship (no. 889682). The content is solely the responsibility of the authors and does not necessarily represent the views of the funding agencies.
Author information
Authors and affiliations.
Department of Psychology, University of Amsterdam, Amsterdam, Netherlands
Denny Borsboom, Sacha Epskamp, Adela-Maria Isvoranu, Claudia D. van Borkulo & Lourens J. Waldorp
Center for Lifespan Psychology, Max Planck Institute for Human Development, Berlin, Germany
Marie K. Deserno
Department of Psychology, University of California, Davis, CA, USA
Mijke Rhemtulla & Anna C. Wysocki
University of Amsterdam, Centre for Urban Mental Health, Amsterdam, Netherlands
Sacha Epskamp & Claudia D. van Borkulo
Department of Clinical Psychology, Leiden University, Leiden, Netherlands
Eiko I. Fried
Department of Psychology, Harvard University, Cambridge, MA, USA
Richard J. McNally
Department of Psychiatry, Massachusetts General Hospital & Harvard Medical School, Boston, MA, USA
Donald J. Robinaugh
Department of Psychology, University of Milan Bicocca, Milan, Italy
Marco Perugini & Giulio Costantini
Santa Fe Institute, Santa Fe, NM, USA
Jonas Dalege
Center for Philosophy of Science, University of Pittsburgh, Pittsburgh, PA, USA
Riet van Bork
You can also search for this author in PubMed Google Scholar
Contributions
Introduction (D.B. and M.K.D.); Experimentation (D.B., M.K.D., E.I.F. and C.D.v.B.); Results (D.B., M.K.D., S.E., A.-M.I. and L.J.W.); Applications (D.B., M.K.D., E.I.F., R.J.M., D.J.R., M.P., J.D. and G.C.); Reproducibility and data deposition (D.B., M.K.D. and G.C.); Limitations and optimizations (D.B., M.K.D., M.R., R.v.B. and A.C.W.); Outlook (D.B. and M.K.D.); Overview of the Primer (D.B. and M.K.D.).
Corresponding author
Correspondence to Denny Borsboom .
Ethics declarations
Competing interests.
The authors declare no competing interests.
Additional information
Peer review information.
Nature Reviews Methods Primers thanks D. Hevey, S. Letina, M. Southward and the other, anonymous, reviewer(s) for their contribution to the peer review of this work.
Publisher’s note
Springer Nature remains neutral with regard to jurisdictional claims in published maps and institutional affiliations.
Related links
International Personality Item Pool: https://ipip.ori.org/
IPIP-Big Five Factor Markers open data: https://openpsychometrics.org/_rawdata/IPIP-FFM-data-8Nov2018.zip
A generic term that subsumes a family of measures that aim to assess how central a node is in a network topology, such as node strength, betweenness and closeness.
The application of statistical models to assess the structure of pairwise (conditional) associations in multivariate data.
Characterization of the global network topology and the position of individual nodes in that topology.
The analysis of multivariate psychometric data using network structure estimation and network description.
The choice of which variables will function as nodes in the network model.
The assessment of estimation precision and robustness to sampling error of psychometric networks.
A statistical association between two variables that does not vanish when taking into account other variables that may explain the association.
In psychometric network analysis, edge weights typically are parameter estimates that represent the strength of the conditional association between nodes.
(PMRF). An undirected network that represents variables as nodes and conditional associations as edges, in which unconnected nodes are conditionally independent.
A generic term to characterize networks in terms of their global topology, for instance in terms of density or architecture.
A term used in personality research to designate the propensity to be self-controlled, responsible, hardworking and orderly and to follow rules. In most models of human personality, conscientiousness is considered a high-order factor.
Specific traits subsumed by a factor in hierarchically organized models of personality. For instance, orderliness and industriousness are facets of conscientiousness.
A parameter of network models that controls the entropy of node state patterns. A network with low temperature will allow only node states that align, such that positively connected nodes must be in the same state and negatively connected nodes must be in the opposite state, whereas a network with high temperature will allow more random patterns of activation.
Daily diary methodology to measure psychological states and behaviours in the moment, for instance by using ambulatory assessment devices such as mobile phones to administer questionnaires that probe how the person feels or what the person does at that specific point in time.
The problem that explanatory models often are not identifiable from the data.
Models for relations between variables of continuous and discrete type based on conditional associations.
A network that represents within-person conditional associations between variables within the same time point. Contemporaneous networks are often estimated after conditioning on effects of the previous time point, as expressed in a time-series model.
A method to determine which edges of a mixed graphical model are to be included and excluded.
A social process that leads to higher prevalence of more extreme attitudes in a population, leading to a bimodal population distribution, with only strong supporters and opponents, rather than a normal distribution in which most people obtain a middle position.
A regularization parameter to determine edge inclusion/exclusion that obtains a nominal false positive rate.
The amount by which an estimate differs from the target value.
An algorithm to obtain a network in which each node, in turn, is used as the dependent variable in a penalized regression function to identify which other nodes are connected to the relevant node.
Approaches that do not use a penalized likelihood function in network structure estimation but rely on different methodologies for edge selection, such as null hypothesis testing or Bayesian approaches.
A concept that expresses the degree to which two nodes have the same position in the network topology. Two nodes with high topological overlap have very similar connections to other nodes.
Rights and permissions
Springer Nature or its licensor (e.g. a society or other partner) holds exclusive rights to this article under a publishing agreement with the author(s) or other rightsholder(s); author self-archiving of the accepted manuscript version of this article is solely governed by the terms of such publishing agreement and applicable law.
Reprints and permissions
About this article
Cite this article.
Borsboom, D., Deserno, M.K., Rhemtulla, M. et al. Network analysis of multivariate data in psychological science. Nat Rev Methods Primers 1 , 58 (2021). https://doi.org/10.1038/s43586-021-00055-w
Download citation
Accepted : 12 July 2021
Published : 19 August 2021
DOI : https://doi.org/10.1038/s43586-021-00055-w
Share this article
Anyone you share the following link with will be able to read this content:
Sorry, a shareable link is not currently available for this article.
Provided by the Springer Nature SharedIt content-sharing initiative
This article is cited by
Differences in the depression and burnout networks between doctors and nurses: evidence from a network analysis.
- Zheng Zhang
- Jiansong Zhou
BMC Public Health (2024)
New psychometric evidence from the Revised Mental Health Inventory (R-MHI-5) in Peruvian adolescents from a network psychometrics approach
- Estefany Rojas-Mendoza
- Vaneryn Alania-Marin
- Aaron Travezaño-Cabrera
BMC Psychology (2024)
Network analysis of Tourette syndrome and attention-deficit/hyperactivity disorder symptoms in children and adolescents
- Wenyan Zhang
- Zhongliang Jiang
- Tianyuan Lei
Child and Adolescent Psychiatry and Mental Health (2024)
Pathways to experienced coercion during psychiatric admission: a network analysis
- Benedetta Silva
- Stéphane Morandi
- Philippe Golay
BMC Psychiatry (2024)
eHealth tools use and mental health: a cross-sectional network analysis in a representative sample
- Dominika Ochnik
- Marta Cholewa-Wiktor
- Magdalena Pataj
Scientific Reports (2024)
Quick links
- Explore articles by subject
- Guide to authors
- Editorial policies
Sign up for the Nature Briefing: AI and Robotics newsletter — what matters in AI and robotics research, free to your inbox weekly.

Our systems are now restored following recent technical disruption, and we’re working hard to catch up on publishing. We apologise for the inconvenience caused. Find out more: https://www.cambridge.org/universitypress/about-us/news-and-blogs/cambridge-university-press-publishing-update-following-technical-disruption
We use cookies to distinguish you from other users and to provide you with a better experience on our websites. Close this message to accept cookies or find out how to manage your cookie settings .
Login Alert
- < Back to search results
- Network Science
Network Science
- Submit your article
- Announcements
This journal utilises an Online Peer Review Service (OPRS) for submissions. By clicking "Continue" you will be taken to our partner site https://mc.manuscriptcentral.com/nws . Please be aware that your Cambridge account is not valid for this OPRS and registration is required. We strongly advise you to read all "Author instructions" in the "Journal information" area prior to submitting.
- Information
- Journal home
- Journal information
- FirstView articles
- Latest issue
- Open access articles
We’re delighted to announce that all articles accepted for publication in Network Science from 10th November 2023 will be ‘open access’; published with a Creative Commons licence and freely available to read online (see the journal’s Open Access Options page for available licence options). The costs of open access publication will be covered through agreements between the publisher and the author’s institution , payment of APCs by funding bodies, or else waived entirely, ensuring every author can publish and enjoy the benefits of OA.
Please see the journal's Open Access Options page for instructions on how to request an APC waiver.
See this FAQ for more information.
- You have access: full Access: Full
- Open access
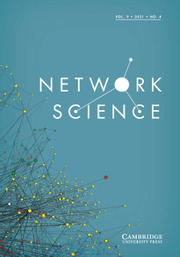
- ISSN: 2050-1242 (Print) , 2050-1250 (Online)
- Editor: Martin Everett University of Manchester, UK
- Editorial board
Recently published papers
Guiding prevention initiatives by applying network analysis to systems maps of adverse childhood experiences and adolescent suicide.
- Benjamin D. Maldonado , Ryan Schuerkamp , Cassidy M. Martin , Ketra L. Rice , Nisha Nataraj , Margaret M. Brown , Christopher R. Harper , Curtis Florence , Philippe J. Giabbanelli
- Network Science , First View
The latent cognitive structures of social networks
- Izabel Aguiar , Johan Ugander
When can networks be inferred from observed groups?
- Zachary P. Neal
- Network Science , Volume 12 , Issue 2
Algorithmic aspects of temporal betweenness
- Sebastian Buß , Hendrik Molter , Rolf Niedermeier , Maciej Rymar
Generating preferential attachment graphs via a Pólya urn with expanding colors
- Somya Singh , Fady Alajaji , Bahman Gharesifard
Automated detection of edge clusters via an overfitted mixture prior – CORRIGENDUM
- Hanh T. D. Pham , Daniel K. Sewell

A generalized hypothesis test for community structure in networks
- Eric Yanchenko , Srijan Sengupta
Methodological moderators of average outdegree centrality: A meta-analysis of child and adolescent friendship networks
- Jennifer Watling Neal
Elisa Bellotti, University of Manchester, UK Noshir Contractor, Northwestern University, USA Martin Everett, University of Manchester, UK Laura Koehly, National Human Genome Research Institute, USA Matteo Magnani , Uppsala University, Sweden Ann McCranie , Indiana University, USA Filippo Menczer, Indiana University, USA Nicola Perra, University of Greenwich, UK Paolo Pin, University of Siena, Italy Christoph Stadtfeld , ETH Zürich, Switzerland Fernando Vega-Redondo , Bocconi University, Italy Alessandro Vespignani, Northeastern University, USA Stanley Wasserman, Indiana University, USA
Managing Editor
Vee Everett, London, UK
Full Editorial Board
Explore related books from Cambridge
2023 Journal Citation Reports © Clarivate Analytics
What is network science?
- ULRIK BRANDES , GARRY ROBINS , ANN McCRANIE , STANLEY WASSERMAN
- Network Science , Volume 1 , Issue 1
Network Topology - Science topic

- Recruit researchers
- Join for free
- Login Email Tip: Most researchers use their institutional email address as their ResearchGate login Password Forgot password? Keep me logged in Log in or Continue with Google Welcome back! Please log in. Email · Hint Tip: Most researchers use their institutional email address as their ResearchGate login Password Forgot password? Keep me logged in Log in or Continue with Google No account? Sign up

An official website of the United States government
The .gov means it’s official. Federal government websites often end in .gov or .mil. Before sharing sensitive information, make sure you’re on a federal government site.
The site is secure. The https:// ensures that you are connecting to the official website and that any information you provide is encrypted and transmitted securely.
- Publications
- Account settings
The PMC website is updating on October 15, 2024. Learn More or Try it out now .
- Advanced Search
- Journal List
- Springer Nature - PMC COVID-19 Collection

Influential nodes identification in complex networks: a comprehensive literature review
Khaoula ait rai.
1 Computer System and Vision Laboratory, Faculty of Sciences Agadir BP8106, Ibn Zohr University, Agadir, Morocco
Mustapha Machkour
Jilali antari.
2 Laboratory of Computer Systems Engineering, Mathematics and Applications, Polydisciplinary Faculty of Taroudant, Ibn Zohr University, B.P. 8106, Agadir, Morocco
Associated Data
The datasets used and/or analyzed during the current study are available from the corresponding author on reasonable request.
Researchers have paid a lot of attention to complex networks in recent decades. Due to their rapid evolution, they turn into a major scientific and innovative field. Several studies on complex networks are carried out, and other subjects are evolving every day such as the challenge of detecting influential nodes. In this study, we provide a brief overview of complex networks, as well as several concepts key related to measurements, the structure of complex network and social influence, an important state of the art on complex networks including basic metrics on complex networks, the evolution of their topology over the years as well as the dynamic of networks. A detailed literature about influential finding approaches is also provided to indicate their strength and shortcomings. We aim that our contribution of literature can be an interesting base of information for beginners’ scientists in this field. At the end of this paper, some conclusions are drawn and some future perspectives are mentioned to be studied as new directions in the future. More detailed references are provided to go further and deep in this area.
The study of complex networks has been the subject of great attention from the scientific community and has proved useful in many fields such as physics, biology, telecommunications, computer science, sociology and epidemiology. Complex networks (CN) become a major scientific research field. In our daily life, there are several examples of complex networks. For instance, the world wide web is a real network composed of web pages connected by hypertext links; internet is a network of computers and routers attached by optical fibers; metabolic networks is a network of interaction between metabolites; neural networks represent simple neurons in brain linked to form a complex system. Such CN and others can be modeled as graphs composed of nodes that interact with each others, and the interaction between nodes is presented by links or edges. Graph theory is a powerful tool that has been employed in a variety of complex network studies [ 1 , 2 ]. The modeling of these systems allowed us to explore them, to understand their mathematical description, to understand their various behavior and to predict it. The modeling consists of creating coherent models that reflect the properties of real networks as much as possible. In real networks, while local interactions are well known such as the communication between routers and the protein–protein interaction, the overall result of all the interactions is still poorly understood (emergence property). For a better understanding of the characteristics of networks, we will need a formalism that encompasses the structure of the network (static approach) and its function (dynamic approach) [ 3 ]. The analysis of complex networks relies on knowing some fundamental concept such as network measurements, network structure, and social influence.
Models and real networks can be compared using network measurements. These measurements can express the most suitable topological features and can be an efficient source for networks investigation. Clustering coefficient, average path length, and degree distribution are some statistical measurements that can define the structure and the behavior of networks. An overview about these measurements is provided in Sect. 2 . The structure of a network means the way each node is arranged. It is the underlying layer of network’s dynamics [ 4 , 5 ]. Analyzing the dynamic of networks allows us to find out different behaviors of networks either in static or variable state.
With the study of network structure, the identification of influential nodes and the detection of community are an important issues that have recently been dealt by the scientific community. The detection of community is addressed in a range of methods. Each method has its own characteristics. The second issue is determining which nodes in networks are important; different approaches are proposed to fix this challenge. These approaches are divided into four categories: structured approaches (local, semi local, global and hybrid methods), Eigen vector-based approaches which rely on the quantity of neighbors and their influences, multi-criteria decision making (MCDM)-based approaches and machine learning-based approaches. Each method has its limitations. There are methods that consider local network information or methods that consider global information or methods that rely on feature engineering and the selection of this features. We give later a detailed comparison summary table of some used approaches to extract similarities and differences between them.
The main contributions of this paper are the presentation of a relevant state-of-the-art review on complex networks, and all concepts related to them like measurements, structure, social influence, and especially the influential node approach. A comprehensive review and categorization of different approaches used in influential node findings are presented to highlight their main advantages and weaknesses. Hoping that this paper will help scientists with the analysis in this field.
The rest of this paper is organized as follows: Sect. 2 provides the main text of the manuscript, a quick review of our subject's fundamental concepts is provided in Sect. 2.1 . An interesting literature review about complex networks is highlighted in Sect. 2.2 . The third sub-section discusses methods for detecting influential nodes. Section 4 is a summary of the classification of several papers. In Sect. 3 , we draw conclusions and some perspectives.
Fundamental concepts
In this section, we present some basic concepts and definitions that will be used in this article.
Complex network
In the context of network theory, CN is a network of interactions between entities whose overall behavior is not deductible from the individual behaviors of the said entities, hence the emergence of new properties. It refers to all entities that are linked to each other in some way. In other word, CN is a graph (network) with nontrivial topological features, features that do not occur in simple networks such as random networks, but often occur in networks representing real systems. The study of complex networks is a young and active field of scientific research largely inspired by the empirical findings of real-world networks such as:
- Social networks A social network, such as Facebook or Twitter, is a collection of social actors, such as persons or groups, connected by social interactions. It is a set of vertices and edges that describes a dynamic community.
- Biological networks for example, metabolic networks with proteins as nodes and chemical interactions as links.
- Infrastructure networks for example, transport networks whose nodes are airports and the links are air links as well as electricity networks (cables between places of production and consumption).
Most social, biological, and technological networks exhibit substantial non-trivial topological features, with connection patterns between their elements that are neither purely regular nor purely random [ 6 ]. These characteristics include a heavy tail in the degree distribution, a high clustering coefficient, assortativity or dissortativity between vertices, community structure, and hierarchical structure. In the case of directed networks, these characteristics also include reciprocity, triad importance profile, and other characteristics [ 7 ]. In contrast, many mathematical models of networks that have been studied in the past, such as networks and random graphs, do not exhibit these characteristics. The most of complex structures can be realized by networks with an average number of interactions. It is often possible to predict the functionality or understand the behavior of a complex system if we can verify certain "good properties" by analyzing the underlying network [ 8 ]. For example, if we detect clusters of vertices with the same topological characteristics of the network, we can obtain information about the particular roles played by each vertex (e.g., hubs, outliers) or how whole clusters describe or affect the general behavior of the CN [ 9 ]. The use of graph theory to model networks as graphs makes it easier to examine and understand their structure. Graphs are used to model this, with nodes representing entities and links representing relationships. A graph G is a couple V , E where: V = v 1 , v 2 , ⋯ , v n such as n = v is a set of vertices or nodes. E = e 1 , e 2 , ⋯ . , e m such as m = e is a set of edges or links. If each edge E is an unordered pair of nodes, the edge is undirected and the network is an undirected network. Otherwise, if each edge is an ordered pair of nodes, the edge is directed from node to other and the network is a directed or oriented network. In this case, an ordered pair of nodes ( u , v ) is a directed edge from node u to node v . If each edge has an associated numeric value called a weight, the edge is weighted and the network is a weighted network [ 10 ]. Figure 1 shows three examples of networks including undirected, directed and weighted network (undirected).

Examples of networks
Two well-known and much-studied classes of complex networks are scale-free networks [ 11 , 12 ] and small-world networks [ 13 , 14 ], whose discovery and definition are canonical case studies in the field. Both are characterized by specific structural features: power-law degree distributions for the first class [ 15 ], short path lengths and high clustering for the second class. Examples of these classes are presented in Fig. 2 . The random network is virtually homogeneous and follows the Poisson distribution. Nearly all nodes have the same number of links. Road network is an example of this class. scale-free network: An inhomogeneous network that exhibits power-law behavior. The majority of nodes have one or two links, but a few densely connected nodes, or "hubs," have many links. Airline networks is an example of this class. However, as the study of complex networks has continued to grow in importance and popularity, many other aspects of network structures have also attracted attention [ 16 , 17 ]. Section 3 presents these classes with their characteristics.

The random network and scale free network a the random network is virtually homogeneous and follows the Poisson distribution. Nearly all nodes have the same number of links. b Scale-free network: An inhomogeneous network that exhibits power-law behavior. The majority of nodes have one or two links, but a few densely connected nodes, or "hubs," have many links.in the scale-free network, the largest hubs are highlighted with dark circles, nodes are presented with white circles [ 18 ]
Recently, the study of complex networks has been extended to networks of networks. If these networks are interdependent, they become significantly more vulnerable than single networks to random failures and targeted attacks and exhibit cascading failures and first-order percolation transitions [ 19 ]. In addition, the collective behavior of a network in the presence of node failure and recovery has been studied. It has been found that such a network can have spontaneous failures and spontaneous recoveries [ 20 ].
The field continues to grow at a rapid pace and has brought together researchers from many fields, including mathematics, physics, biology, computer science, sociology, epidemiology, and others. Ideas and tools from network science and engineering were applied to the analysis of metabolic and genetic regulatory networks; the study of the stability and robustness of ecosystems; clinical science; modeling and design of scalable communication networks such as generation and visualization of complex wireless networks; the development of vaccination strategies for disease control; and a wide range of other practical issues [ 21 ]. In addition, network theory has recently proven useful in identifying bottlenecks in urban traffic. Network science is the subject of many conferences in a variety of different fields [ 22 ].
Network measurements
In the field of complex network, measurements can demonstrate the most relevant topological features, especially after the representation of the network structure, the analysis of the topological characteristics of the obtained representation carried out in the form of a set of informative measurements. During the modeling process, some respective measurements are used for comparing models with real networks. That is why it is an essential resource in many network investigation [ 23 ].
Thereafter, some measurements that can be used to measure significant properties of complex systems. We consider then a graph G with G V , E . V is a set of nodes, and E is a set of edges.
Density the density d of a graph G is the proportion of links existing in G compared to the total number of possible links: G = 2 m / n n - 1 . If m is of the order of n , the graph is said to be sparse (as opposed to dense graphs). Indeed, this measure is sensitive to the number of vertices, so the density equal to 0 corresponds to the graph where all the vertices are isolated, and equal to 1 in the case of a complete graph. In a graph resulting from empirical observations, the more the number of vertices increases, the more the density tends to decrease.
Shortest path it is the length of the shortest path connecting two nodes in the network. One of the algorithms for calculating the distance between two nodes in a graph is: Dijkstra's algorithm [ 24 ]. The average distance between two pairs of nodes makes it possible to evaluate the transmission time required between two “any” individuals.
Diameter the diameter of a network is formally the longest of the shortest paths between two entities, or nodes, of the network, via its connections. It allows for example to know the maximum time to transmit the disease.
Degree the degree d i of a node is the number of edges incident to node i , in other words, the number of neighboring nodes of i .
Degree distribution perhaps calculated as follows [ 25 ]:
δ v denotes the number of vertices of the network G having degree k and N : denotes the size of G (number of nodes). The above equation represents the proportion of vertices of G having degree k . The degree k i of node i is the number of links connected to node i . The distribution of degrees allows the understanding of the distribution of connectivity and the structure of the network.
Clustering coefficient is the probability that two neighbors of a node are also neighbors to each other. It can be interpreted as the probability that two nearest neighbors of i are connected to each other.
The average clustering coefficient of the graph G is the average of the clustering coefficient of all its vertices (nodes). In the literature, there are two definitions of the clustering coefficient: global clustering coefficient (also called transitive) and local clustering coefficient on average [ 26 ]:
The global clustering coefficient is defined as:
A triangle is a complete subgraph with three nodes;
A connected triple is a set of three vertices with at least two links between them.
Closeness centrality indicates if a node is located close to the all other nodes of the graph and if it can quickly interact with them. It is written formally [ 27 ]:
With d G u , v is the distance between nodes u and v .
Betweenness centrality is one of the most important concepts. It measures the usefulness of the node in the transmission of information within the network. The node plays a central role if many shortest paths between two nodes have to go through this node [ 27 ]. Formally, we express it as:
with σ ij v the number of paths between i and j that go cross v .
Vulnerability A node’s vulnerability is defined as the decrease in performance that occurs when the node and all of its edges are removed from the network.
where E is the original network's global efficiency and E i is the global efficiency after omitting node i and all its edges.
Complex network structure
The way in which the nodes are arranged is another aspect in the study of the complex networks or the study of their structure. The structure refers to the real-world network modeling research that has been done. Several models, however, appear to explain how small world networks and scale-free features emerge in the real world: Watts and Strogatz proposed a model [ 26 ] to explain how the two characteristics of small world networks, a high clustering coefficient and a low average path length, expound in networks. Barabàsi and Albert offered a model [ 28 ] to show how networks with power-law degree distribution emerge in networks.
Usually, models of networks can help us to understand the meaning of these properties, we can classify these models in two categories:
- Evolving models explains the evolution of the complex network as a function of time in order to show how these networks behavior develop and to determine the laws governing the evolution of physical systems. ex: Barabási and Albert for scale free networks [ 28 ].
- Static models show how networks are structured and how some properties of complex networks are present. The Watts and Strogatz model it is an example that explains the appearance of high clustering coefficient and low average path length in networks according to time [ 26 ].
There are several aspects in terms of the structure of a network that can be useful for predicting the overall behavior of a complex network, in terms of clusters how are interconnected, how to communicate with each other, how identify influential nodes in complex networks, how network structure affect the dynamics of social systems.
Social influence
The social influence is the most important topic in the field of complex system especially social network. We cannot talk about these type of networks without talking about spreading idea and information and the impact of this information on our society. Interactions between actors of social networks are the means by which information spreads. The maximizing of social influence is one of the issues concerning information propagation. It is essential to find a group of the most influential individuals in a social network so that they can extend their influence to the largest scale (influencers). In other words, the activation of these nodes can cause the propagation of information in the whole network. The problem of maximizing social influence has been an important research topic for many years because it has a considerable impact on the society. Some parties are interested by the progress in this area in order to optimize the spreading of information and new ideas through social network. Viral marketing is one of its application. The principle is that to promote a new service for all potential customers. The brand can target a limited group of clients who will subsequently tell their friends and acquaintances about the service. Other application of spreading information is the political company via social networks [ 29 ].
Literature review on complex networks
In past decades, CN have gotten much consideration from researchers and nowadays, they have become a subject key in many areas of science. Studies on CN show that the modeling of these systems the complexity reduces to a level that we can manage them in a practical way [ 30 ]. The graphical properties produced by this modeling are similar to the real system [ 30 ]. There are various examples of complex networks in our daily life. Despite the fact that various measures have been suggested by researchers about complex networks, there are three basic metrics that can describe the characteristics of complex networks. These metrics are average path length [ 4 ], clustering coefficient and degree distribution. Degree distribution is a probabilistic distribution of the degrees of each node of the network [ 31 ]. Clustering coefficient evaluates the level of local or global transitivity of a graph. In other words, we study the links at the level of the triads (relations between three nodes) and we check whether, when there is a link between the nodes ab and bc, there is also a link between the nodes a and c. The average path length is the average length of shortest path between any two vertices [ 32 ].
Formerly, researches on complex networks focus on the topological structure of the network and its characteristics as well as its dynamics. The objective of studying and analyzing complex networks is not only to understand different real systems but also to achieve an effective control of these networks. In fact, to predict and control such a complex system or network an understanding of the mathematical description of these systems is necessary [ 30 ]. According to the dynamic process of complex networks, networks can be divided into two classes: static and temporal. The study of complex networks began with this class where the presence of nodes and links is unrelated from any idea of time. The static network contains nodes and edges altered gradually over times or fixed permanently. It is widely studied and suitable for analytical traceability [ 30 ]. In the dynamic class, the concept of time is relevant and the existence of links and nodes is time-sensitive, they are not always granted to exist. This kind of network is more realistic. Links between nodes in these networks may appear or disappear over time, the scientific collaboration network as an example [ 30 ]. There is a lot of sub-classes under these two classes (static-temporal). Networks can be distinguished according to their distribution degree, the average distance and other metrics. Models are developed through the year from simple lattices until improved models. Lattices are the simple models of networks. They are suitable for solving analytic problems [ 30 ] such as Ising Model [ 33 ] and Voter Model [ 34 ]. They have a simplified structure but are unrealistic in comparison with real-world systems [ 30 ], this is why the historical evolution of the models knows more improvement by taking into account more real characteristics. Afterward, in 1959 Erdos and Rényi explored another basic network mockup is the random regular network [ 35 ]. Watts and Strogatz [ 26 ] proposed the small-world model. It is more realistic and social network-inspired. Barabasi and Albert [ 13 ] developed a preferential attachment model that might be used to reproduce the time growth features of many real networks. Nodes are added in this model at each step by creating links with the already existing nodes with a proportional probability of their degrees at that moment. A model close to the BA (Barabasi and Albert) network was proposed by Bianconi and Barabasi [ 14 ] (Fitness Model). This model relies in addition to degree, on the fitness of each node for realizing new links. A new idea in BA models is introduced by Almeida et al. [ 13 ]. This idea is homophily, and models are christened homophilic model. Homophilic models rely on degree, fitness and also similarity between nodes for example similarity of jobs or similarity of interests, etc. Catanzaro et al. provide an algorithm for creating uncorrelated random networks (URN), despite the fact that this model is uncommon in real networks. URN is created in order to reach a theoretical solution for the behavior of dynamical systems. Waxman [ 36 ] suggested a generalization model of the Erdos–Renyi graph in 1988 (spatial Waxman Model). The challenge of building longer connections between nodes is fully considered in this model. Rozenfeld et al. [ 11 ] proposed the scale free on lattice. When creating new links, this model considers the Euclidean distance among nodes. Perra et al. [ 37 ] propose the activity driven model as an example of temporal social network. Actor activity drives relationships in this model. Afterward, the Adaptive networks model is appeared to give the same importance between the topology and the dynamical process [ 38 ]. Metapopulation model [ 39 ] also is presented as network constituted by collection of networks describing interconnected populations. Two level characterizing this model, the first is interpopulation that contain set of individuals and each individual constitutes the intrapopulation level. Multilayer model presents two layers (horizontal and vertical) which contain a two-way dynamic process within the layer and between layers [ 40 ]. Covid 19 is a good example to clarify this model as one of the infectious diseases which is contagious from bat animals to human. In this case, we can model human and their dynamic process as first layer and the same for bats animals as a second layer. There are human-to-human interactions, as there are human-to-animal relationships. Table 1 summarizes all of these network models. For each network model, we highlight its advantages as well as its limits.
Network models and their characteristics
Authors | Networks models | Advantages | Limits |
---|---|---|---|
Jozef Sumec | Regular lattices | Simple models of networks. Suitable for solving analytic problems. | Unrealistic compared with real networks. |
Erdos and Renyi [ ] | Random regular network | Simple prototype of network, homogeneous. | Too restrictive. |
Watts and Strogatz [ ] | Small world networks | Realistic roused from social networks. | With a power-law basis, it is unable to construct heterogeneous degree distribution. |
Barbasi and Albert [ ] | Barbasi Albert model | Appropriate for generating the time growth characteristic among several real—world networks. Model of emergence graph. | The dynamic process is treated as static in this network. Fitness of nodes is not considered for making new links. |
Bianconi and Barbasi [ ] | Fitness model | Similar to BA model. Consider degree and fitness of nodes for making new connections. | Does not predict the impact of homophily. |
Almeida et al. [ ] | Homophilic model | Consider similitude of nodes. Model of emergence of small-world features and power-law degree distribution. | Produces undirected networks, It faces some difficulties in extending this model to directed networks. |
Catanzaro et al. [ ] | Uncorrelated random networks | It is important for checking theoretical solutions of the interactions of dynamical systems. | Unusual in real networks. |
Waxman [ ] | Spatial Waxman model | generalization of the Erdos–Renyi graph Consider geographical properties. | Weak in the prediction of most real systems. |
Rozenfeld et al. [ ] | Scale free on lattice | When creating new links, keep the Euclidean distance between nodes in consideration. | The entire length of the system's links can be kept to a minimum. |
Perra et al. [ ] | Activity driven model | Actor action drives relationships. Example of temporal social network. | Do not consider other features of actor activity like different weights associated with each connection. |
Gross et al. [ ] | Adaptive networks | Useful to model many real systems. With adaptive way, topologies change with changes of node’s states. | There is yet no clear theoretical explanation for large-scale adaptive network limitations. |
Colizza and Vespignani [ ] | Metapopulation model | A network of networks that describes a connected population. Widely used because of the mobility of node. | In spatial epidemiology, it is difficult to represent the essential aspects of spatial transmission of infectious diseases [ ]. |
Mucha et al. [ ] | Multilayer networks | The dynamic process has the potential to propagate inside and between layers. | The spectral characteristics of the graph can be used to identify distinct multiplexity regimes and coupling between layers [ ]. |
In the last few years, there has been a growing interest in community structure and influential nodes in the field of complex network analysis. A large number of articles were published, including a different approach to the problem of community detection as in [ 47 – 53 ]. These referred approaches are classified as approach-based static non-overlapping communities, approach-based static overlapping communities, approach-based hierarchical communities and approach-based dynamic communities [ 54 ]. Researches are also interested in identifying influential nodes. Many approaches are proposed in this context as explained in the following section.
Influential nodes finding approaches
In network science, each node plays a specific role. Nodes do not have the same importance, and some nodes are more important in the network than others remaining nodes due to their important capability of spreading in the whole network. These nodes are known as influential nodes. The identification of significant nodes is necessary in network attacks, network of terrorists, and disease spreading studies. Reason for what, approaches for finding important nodes in complex networks have attracted much interest. Several methods are proposed to identify these nodes: Degree centrality (DC) [ 55 ], betweenness centrality (BC),closeness centrality (CC) [ 56 ], page rank (PR) [ 57 ], Leader rank (LR) [ 58 ], H-index [ 59 ], Hyperlink-Induced Topic Search (HITS) [ 60 ], weighted formal concept analysis (WFCA) [ 61 ], weighted TOPSIS (W-TOPSIS) [ 62 ], Analytic hierarchy process AHP [ 63 ], Least-squares support vector machine LS-SVM [ 64 ]…. These proposed approaches are divided into four categories: structured approaches, vector-based approaches, MCDM-based approaches and machine learning-based approaches.
Structured approaches
In structured approaches, there are several types: local, semi-local, global and hybrid approaches. These techniques can also be classified into two classes: one is based on each node’s neighborhood (including degree centrality, K-shell, and H-index techniques), whereas the other is based on node pathways (such as closeness centrality and betweenness centrality). For local approaches, they determine the impact of nodes based on local data which means they depend on nodes and their neighbors to indicate their influence (impact). For example, H-index and degree centrality (DC), these approaches’ advantages are their simplicity and minimal computational complexity. However, the overall system structure is neglected and important nodes are found mostly in big components of multi-component [ 65 ], which diminishes the adequacy of these methods in extensive scale networks [ 66 ]. In global approaches, the importance of nodes is described by the entire structure of the network, e.g., closeness centrality (CC), betweenness centrality (BC), Coreness centrality (Cnc) [ 56 ], Kshell decomposition [ 59 ]. Centralities like closeness and betweenness are based on paths between nodes. These two measures are not as impactful in large-scale networks as a result of their great complexity of information, Kshell decomposition (Ks) indicates a global location features of network nodes but is not ideal for tree networks. Semi-local approaches use information on neighbors' neighbors (second-order neighbors) not withstanding information on neighbors to determine the spread capacity of a node. Example of these approaches: Weight Degree Centrality ( W DC ) [ 67 ] and Extended Weight Degree Centrality (EW DC ) [ 10 ], in W DC and EW DC the computation of the diagrams assortativity is vital which can prompt more prominent time intricacy in vast scale graphs. Hybrid approaches use global information in conjunction with local information to specify these influential nodes and to determine the extended ability of these nodes, these techniques are based on the Ks index these methods, which are based entirely on the ks index include mixed degree decomposition [ 68 ], neighborhood coreness [ 69 ], k-shell iteration factor [ 66 ] and mixed core, degree and entropy [ 70 ].
Eigenvector-based approaches
Eigenvector-based approaches take into account the quantity of neighbors and their influences, such as: eigenvector centrality [ 71 ], Pagerank (PR) [ 57 ], LeaderRank (LR) [ 58 ], HITS (Hyperlink-Induced Topic Search) [ 60 ]. Eigenvector centrality can be productively determined utilizing a power iteration approach, yet it might end up caught in a zero status, on account of the presence of many nodes without in-degree [ 61 ]. PageRank is a variant of the eigenvector centrality. This famous algorithm is used in Google search engine. Firstly, acquainted with measure the ubiquity of a website page. It expects that the significance of a page is dictated by the amount and nature of the pages connected to it. It has been used in several areas and works well in networks without scale. However, it is sensitive to disturbances of random networks and presents thematic drifts in special network structures [ 61 ]. The HITS algorithm considers every node in the system by including two jobs: the authority and the hub similarly HITS introduces a wonder of topical drift. LeaderRank works well in complex directed networks but seems to be inapplicable on non-directed complex networks.
MCDM-based approaches
Recently, multi-criteria analysis methods (MCDMs) or multiple attribute decision making methods (MADMs) have been used to classify nodes according to their importance, like TOPSIS [ 14 ] W-TOPSIS [ 62 ] and AHP [ 63 ]. Various measurements of centrality have been utilized as multiple attributes of complex networks. However, each attribute assumes an imperative job in TOPSIS, which is not sensible, to cure this issue W-TOPSIS not just considers diverse centrality measures as multiple network attributes, However, it also suggests a new technique for calculating the weight of each attribute. AHP is also applied to detect important nodes and uses the model susceptible-Infected SI to obtain the weights. Yang also mixes entropy with TOPSIS to generate EW—TOPSIS [ 72 ]. In this combination, TOPSIS is based on centrality measures as multi-criteria and the entropy is used to calculate the weight of each factor.
Machine learning-based approaches
Recently, there has been a significant focus on machine learning-based approaches. Least Square Support Vector Machine (LS-SVM) was used by Wen et al. to identify the mapping rules among basic indicators and AHP performance evaluation [ 64 ]. LS-SVM furnishes good supervision for identifying important nodes in large-scale networks. Zhao et al. proposed a model to identify vital nodes based on seven algorithms of machine learning (Naïve Bayes, Decision Tree, Random Forest, Support Vector Machine SVM, K-Nearest Neighbor KNN, Logistic Regression, and Multi-layer Perceptron MLP). This model relies on graph model and rate of infection in the ranking of nodes. Approaches based on machine learning rely a lot on feature engineering, and the selection of these features can influence the performance of these approaches. To handle this task, Zhao et al. [ 73 ] introduced a deep learning model called infGCN. It is based on graph convolutional networks. InfGCN treat in the same time the features of node and the link between them.
Classification summary
In this section, we present different well-known approaches that are used to identify influential nodes and we perform a comparison between them based on some factors. The selected approaches do not present an exhaustive list of research on influential finding nodes approaches. In this comparison, we will focus on the type, the nature and the direction of network used in the approach. The network’s type indicates whether the network is weighted or unweighted. The network's nature indicates whether it is static or dynamic. The network direction indicates whether or not the network is directed. Network size provides the size of the used network. The implementation datasets present datasets used in the implementation of the approach. Table 2 presents the abbreviation and its description for the used complex networks datasets for each technique implementation.
Operational network datasets implemented in the main comparison's referred research
Networks dataset | Common abbreviation | Description |
---|---|---|
LFR benchmark | LFR | Lancichinetti–Fortunato–Radicchi benchmark (An artificial network produced by the LFR algorithm that resembles a real-world network). |
Zebra | ZBR | Animal network that contains interactions between 28 Grévy's zebras ( ) in Kenya. Zebras are represented by nodes, and an edge between two zebras indicates that there was interaction between them during the study. |
Zachary karate club | ZKC | Human Social network of university of karate club that gathers students of the club of karate by Wayne Zachary in 1977. Each node represents a member of the club, and each edge represents a tie between two members of the club. |
Contiguous | CTG | The contiguous zone, the marin boundary between 12NM (Nautical miles) and 24NM. |
Dolphins | DLP | A social network of bottlenose dolphins. The nodes are the bottlenose dolphins (genus ) of a bottlenose dolphin community living off Doubtful Sound, a fjord in New Zealand (spelled in New Zealand). An edge indicates a frequent association. The dolphins were observed between 1994 and 2001. |
Copperfield | CPF | Network of common word (adjacencies between noun and adjectives) for the novel David Copperfield by Charles Dickens. Nodes represent the most commonly occurring adjectives and nouns in the book. Edges connect any pair of words that occur in adjacent position in the text of the book. |
Co authorship in network science | NTS | Co-authorship of scientists in network theory and experiments. |
ELG | Neural network of neurons and synapses in , a type of worm. It consists of around 1000 cells including 302 neurons. | |
Euroroad | ERD | A international E-road network located mostly in Europe. Network includes cities, and an edge connecting two cities indicates that they are linked. It contains 1174 cities. |
Chicago | CCG | Contains a comprehensive list of all current City of Chicago workers with details. |
Hamsterster | HMS | Network is of the friendships and family links between users of the website . It is an independent site created in 2003 or 2004. Hamsterster appears to have been shut down as of October 2014. |
US power grid | UG | Undirected infrastructure network provides data concerning the Western States of the USA of America's power grid. An edge represents a power supply line. A node is either a generator, a transformator or a substation. |
Pretty good privacy | PGP | An online contact network or an interaction network of users of the pretty good privacy (PGP) algorithm. The network contains only the giant connected component of the network. |
Astro physics | ASP | Collaboration or cooperation network based on the e-print arXiv and includes scientific partnerships between authors of articles submitted to the Astro Physics field. If an author co-authored a paper with author , the graph contains a undirected edge from to . The data covers papers in the period from January 1993 to April 2003 (124 months). It begins within a few months of the inception of the arXiv, and thus represents essentially the complete history of its ASTRO-PH section. |
Enron email network | ENR | The Enron email dataset comprises about 500,000 emails sent by Enron Corporation employees. This data was originally made public, and posted to the web, by the Federal Energy Regulatory Commission during its investigation. Nodes of the network are email addresses and if an address sent at least one email to address , the graph contains an undirected edge from to |
Jazz musicians | JZ | Collaboration network between Jazz artists. Each node represents a Jazz artist, and each edge indicates that two artists have collaborated in a band. Two levels of collaborations are studied. First, the collaboration network between individuals, where two musicians are connected if they have played in the same band and second, the collaboration between bands, where two bands are connected if they have a musician in common. |
Email network of URV | URV | The email communication network of the University Rovira I Virgili in Tarragona, Catalonia, Spain. Nodes are users and each edge represents that at least one email was sent. The direction of emails and the number of emails between two persons are not stored. |
BLOGS | BG | Communication network between users of MSN’s (windows live) blog. It’s composed of 3982 nodes and 6803 edges. |
COND-MAT (condense matter physics) | CoundMath | Collaboration network based on the e-print arXiv and includes research partnerships between authors who have submitted articles to the Condense Matter category. If an author co-authored a paper with author , the graph contains a undirected edge from to . If the paper is co-authored by authors this generates a completely connected (sub) graph on nodes. The data covers papers in the period from January 1993 to April 2003 (124 months). It begins within a few months of the inception of the arXiv, and thus represents essentially the complete history of its COND-MAT section. |
Live journal | LJ | Free online blogging community with almost 10 million members where individuals express their friendship toward others. LiveJournal allows members to maintain journals, individual and group blogs, and it allows people to declare which other members are their friends they belong. |
Contact network of inpatients | CNI | Presents link between two inpatients if they have both been admitted to the same hospital. |
Internet Movie database actors in adult films | IMDB | Network of connections between actors who have co-starred in films, whose genre has been labeled by the Internet Movie Database as ‘adult’. The dataset is a bipartite graph in which each node either corresponds to an actor or to a movie. Edges go from a movie to each actor in the movie. It also provides metadata for the nodes like movie/actor name, year of the movie, and genre of the movie. |
Email contact network | EM | The network of email contacts is formed on email messages sent and received at University College London's Computer Sciences Department. |
The Internet at the router level (RL) | RL | The nodes of the RL Internet network are the Internet routers. Two routers are connected if there exists a physical connection between them. |
The Internet at the autonomous system level (AS) | AS | The nodes are autonomous systems that are linked if there is a real connection beyond them. graph of routers comprising the Internet can be organized into sub-graphs called Autonomous Systems (AS). Each AS exchanges traffic flows with some neighbors (peers). We can construct a communication network of who-talks-to- whom from the BGP (Border Gateway Protocol) logs. The data was collected from University of Oregon Route Views Project—Online data and reports. The dataset contains 733 daily instances which span an interval of 785 days from November 8 1997 to January 2 2000. In contrast to citation networks, where nodes and edges only get added (not deleted) over time, the AS dataset also exhibits both the addition and deletion of the nodes and edges over time. |
Product space of economic goods | PS | Is a network that formalizes the idea of relatedness between products traded in the global economy. Proximity network between products according to Ref. |
Word | WAN | Represents an adjacency relation in English text. |
ECP | Presents the problem of identifying proteins based on amino acid sequences in cell localization regions. It contains 336 proteins split into 8 different classes. | |
Tandem affinity purification | TAP | Yeast protein–protein binding network generated by tandem affinity purification experiments. |
Yeast 2 hybrid | Y2H | Yeast protein–protein binding network generated using yeast two hybridization. It is originally created by Fields and Song. Is a genetic system wherein the interaction between two proteins of interest is detected via the reconstitution of a transcription factor and the subsequent activation of reporter genes under the control of this transcription factor. |
Power | PWR | Connections between power stations. |
Internet (router level) | Int | Symmetrized snapshot of the Internet ‘s structure at the level of autonomous systems, the network size is 22963. |
FB | This consists of friends lists from . Nodes represents actors or friends and edge represent the relationship between them. | |
TW | Microblogging social network operated by the company Twitter Inc. It allows a user to send free text messages, called tweets, over the internet, by instant messaging or by SMS. | |
The John Padgett—Florentine Families Dataset | JPFF | Multiplex network with 2 edge types representing marriage alliances and business relationships between Florentine families during the Italian Renaissance. Data hosted by Manlio De Domenico. Marriage and commercial links between Renaissance Florentine families are represented in this dataset. |
Delicious.com | DLC | Feature network. This dataset includes labeled web pages obtained from the website delicious.com. Left nodes represent tags, right nodes represent URLs and an edge shows that a URL was tagged with a tag. |
UsairPort | UP | Network of direct flights linking US airports in 2010. Each edge represents a connection from one airport to another, and the weight of an edge shows the number of flights on that connection in the given direction, in 2010. |
AirLines | AL | Flight arrival and departure data for all commercial flights from 1987 to 2008. |
American College Football Network | ACF | Interaction network that represents Football games between Division IA institutions during the regular season in the Fall 2000. |
Yeast | YST | Metabolic network. The dataset consists of a protein–protein interaction network. Research showed that proteins with a high degree were more important for the survival of the yeast than others. A node represents a protein and an edge represents a metabolic interaction between two proteins. The network contains loops. |
Router | RTR | Routing network composed of 5022 nodes and 12 516 connections. |
Human protein | HP | A network of protein–protein interactions that includes physical contacts between proteins that have been experimentally demonstrated in humans, such as metabolic enzyme-coupled interactions and signaling interactions. Nodes represent human proteins and edges represent physical interaction between proteins in a human cell. |
General relativity and quantum cosmology collaboration network | CA-GrQc | The collaboration network derives from the e-print arXiv and contains scientific partnerships between authors on articles submitted to the category of General Relativity and Quantum Cosmology. If an author co-authored a paper with author , the graph contains a undirected edge from to . The data covers papers in the period from January 1993 to April 2003 (124 months). It begins within a few months of the inception of the arXiv, and thus represents essentially the complete history of its GR-QC section. |
High energy physics theory collaboration network | Ca-HepTh | collaboration network is from the e-print arXiv and covers scientific collaborations between authors papers submitted to High Energy Physics—Theory category. If an author co-authored a paper with author , the graph contains a undirected edge from to . If the paper is co-authored by authors this generates a completely connected (sub)graph on nodes. The data covers papers in the period from January 1993 to April 2003 (124 months). It begins within a few months of the inception of the arXiv, and thus represents essentially the complete history of its HEP-TH section. |
Groad | GRD | Highway network of 1168 nodes. |
For the benchmarking approaches used in the detection of influential nodes, a list of real and artificial networks is presented above in Table 2 . This step of benchmarking is important to see how the approach or the algorithm is efficient, and also, it can give us the ability to compare results of different approaches on the same dataset.
We give, in the following comparison table, examples of employed implementation datasets (refer to Table 2 ) in each specified reference, as well as other features as follows:
The following comparison offers an overview of the most widely used techniques in this problematic of influential node’s detection. All of these techniques show their effectiveness throw various experimentation and produce results differentiated by their calculation, limitations, complexity, time of execution, nature and size of network.
In this table, there are some approaches that are in the same spirit for example PageRank and HITS. Both of them utilize the connection structure of the Web graph to determine the pertinence of the pages. HITS works on small subgraph representing the connection between hub and authority websites from the webgraph which explains their complexity that is inferior of O log N . The limitations of PageRank are that does not account for time; also, it is unable to handle advanced search queries. It is unable to analyze a text in its entirety while searching for keywords. Instead, Google interprets these requests and filters search results using natural language processing NLP. From these experiments on the datasets mentioned above, there are some methods that have low time complexity, for example, the k-shell algorithm, HKS, MDD, KS-IF, and Cnc, their time complexity is O n where n is the number of edges in the network. The k-shell decomposition approach was initially developed for unweighted undirected networks, but it has lately been expanded to other kinds of networks. The k-shell approach was expanded by Garas et al. [ 74 ] to recognize core-periphery structure in weighted networks. In K-shell decomposition, the K-shell value is not an appropriate metric for measuring influence. The k-shell index's monotonicity is lower than other centrality indices. MDD is proposed to remedy the problem of the k-shell method where the exhausted degree, as well as the residual degree, are taken into account. AHP, TOPSIS, and W-TOPSIS also have the same philosophy to aggregate centralities to evaluate the influence of nodes. They consider local information and global structure to identify influential nodes. TOPSIS is implemented under four real directed and undirected networks, and it demonstrates their practicability. AHP is implemented under four real undirected networks, and the SI model is used to confirm the accuracy of ranking nodes using AHP. This method outperforms W-TOPSIS. W-TOPSIS is extended to dynamic networks in other work by Pingle Yang et al. [ 75 ]. LS-SVM is implemented on an artificial network using two network models: WS-small world network and power-law BA scale-free network, real networks also are used in an implementation like the US aviation network, dolphin social network, American college football, netscience, and email network. LS-SVM reduced the computation-intensive evaluation of node importance to a basic calculation of the nodes' basic indicators. infGCN proves its accuracy on five different real networks (different types and sizes). Experimental results on these networks indicate that InfGCN can strongly increase prediction accuracy.
The topology characteristics of the networks have an effect on the index accuracy. The performance of the same index varies among networks. In some situations, it can be challenging to select the indices that will best identify the influential nodes. Therefore, finding influential nodes is still a current unresolved problem.
In this paper, a short review of complex networks is presented. Some taxonomy around complex networks is summarized, like the structure of networks, measurements of the network, and social influence within networks. A literature review is provided including the evolution of networks and models through the years, from simple lattices to more complex models. The pros and cons of each model are highlighted with some references for those who want to go further with this issue. In addition, we provide a detailed comparison review between approaches used to identify influential nodes as mentioned above in Table 3 . Throw this comparison, this paper clarifies some strengths of each approach in order to help beginner researchers in this field to identify the relevant directives for their future contributions to this problem of influential node identification. This given work of literature review does not cover all available works related to the identification of influential nodes. Although dynamic networks rely on variations in characteristics and the emergence of properties of networks over time, the majority of approaches are applied to static networks rather than dynamic ones. It really requires working on dynamic networks again. From future perspectives, we can adapt existing approaches of identifying influential nodes to dynamic networks. Additionally, we can combine existing methods with the aim of taking advantage of both methods and achieving a balance between them as we can combine machine learning and deep learning algorithms with other methods.
Influential nodes finding approaches comparison
References | Approach | Network type | Network nature | Network direction | Network size | Implementation datasets |
---|---|---|---|---|---|---|
[ ] | HKS | Unweighted/weighted | Static | Undirected | All | LFR, ZBR, ZKC, CTG, DLP, CPF,NTS, ELG, ERD, CCG, HMS, UG, PGP, ASP, ENR |
[ ] | Coreness centrality (Cnc) | Unweighted | Dynamic | Undirected | All | ZKC, DLP, JZ,ELG,NTS, URV, BG, UG, BA, LFR PG, ASP, CA-CondMat, ENR, EM |
[ ] | Kshell decomposition | Weighted/Unweighted | Dynamic | Directed/undirected | Medium and large | LJ, EM, CNI, IMDB, CondMat RL, AS, PS |
[ ] | Mixed degree decomposition (MDD) | Unweighted | Static | Undirected | All | DLP, JZ, NTS, EM, Ca-HepTh, PGP, ASP CondMat, WAN, ECP, ELG, TAP, Y2H, PWR, Int |
[ ] | k-shell iteration factor (KS-IF) | Unweighted | Dynamic | Undirected | All | LFR,ZKC,DLP, JZ, NTS, EM, BG, PGP, ENR,FB, TW |
[ ] | Eigenvector centrality | Unweighted | Static | Directed | Small | JPEF |
[ ] | PageRank | Unweighted/weighted | Dynamic | Directed | Large | Google search Engine |
[ ] | LeaderRank | Unweighted | Static | Directed | Large | DLC |
[ ] | HITS | Weighted/unweighted | Dynamic | Directed | Small | Clever search engine |
[ ] | TOPSIS | Unweighted | Static | Undirected, directed | Medium and large | UP, AL, EM, ACF |
[ ] | W-TOPSIS | Unweighted | Static | Undirected | Large | YST, BG, RTR, PGP |
[ ] | AHP | Unweighted | Static | Undirected | Medium and large | EM, GRD, YST, UP |
[ ] | LS-SVM | Unweighted/Weighted | static | Undirected/directed | All | WS small-world network, power-law, BA scale-free network, UP, DLP,ACF, NTS, EM |
[ ] | infGCN | Unweighted | Static | Undirected | Large | HMS, HP, CA-GrQc, CA-HepTh, CondMat |
Acknowledgements
Not applicable.
Abbreviations
CN | Complex networks |
MCDM | Multi-criteria decision making |
WS | Watts and Strogatz |
BA | Barabasi and Albert |
DC | Degree centrality |
BC | Betweenness centrality |
CC | Closeness centrality |
PR | PageRank |
LR | LeaderRank |
HITS | Hyperlink-induced topic search |
WFCA | Weighted formal concept analysis |
W-TOPSIS | Weighted TOPSIS |
AHP | Analytic hierarchy process |
LS-SVM | Least-squares support vector machine |
Cnc | Coreness centrality |
Ks | Kshell decomposition |
W | Weight degree centrality |
EW | Extended weight degree centrality |
MADMs | Multiple attribute decision making methods |
EW–TOPSIS | Entropy weighted TOPSIS |
SVM | Support vector machine |
KNN | K-nearest neighbor |
MLP | Multi-layer perceptron |
MDD | Mixed degree decomposition |
KS-IF | K-shell iteration factor |
NLP | Natural language processing |
Author contributions
This work was carried out in collaboration among all authors. All authors read and approved the final manuscript.
Availability of data and materials
Declarations.
The authors declare that they have no conflict of interest.
Publisher's Note
Springer Nature remains neutral with regard to jurisdictional claims in published maps and institutional affiliations.
To read this content please select one of the options below:
Please note you do not have access to teaching notes, networking capability: a systematic review of literature and future research agenda.
Journal of Business & Industrial Marketing
ISSN : 0885-8624
Article publication date: 5 April 2021
Issue publication date: 3 January 2022
The purpose of this paper is to scrutinize the existing literature and provide a comprehensive overview by compiling and synthesizing the dispersed body of knowledge in the networking capability (NC) literature stream. Therefore, this review study contributes to the research by presenting a summary of the NC complex construct.
Design/methodology/approach
The authors have tried to cover the literature gap in the NC area through a systematic review.
The theoretical contributions of this study to the NC literature stream are fourfold. First, while critically reviewing existing definitions, the authors present an integrated definition of NC. Second, the authors delineate the conceptual boundary between NC and other similar capabilities. Third, the authors found that the focal firms’ NC consists of two key dimensions: network development capability (NDC) and network management capability (NMC). Fourth, they contribute to the literature by providing a set of suggestions for further research in the NC literature stream.
Research limitations/implications
This study has some limitations, like other review studies. First, although the authors have tried their best to conduct a comprehensive literature search, they may have missed a study. Second, it should be noted that this study has confined itself to a particular stream of literature. Undoubtedly, in other streams, there will be issues similar to the concepts discussed in this study. The main purpose of this study is to integrate a series of studies that have a common theoretical foundation and fall within a particular literature stream. Although the focus of this study may be positive, it can be said that the deprivation of achievements from other similar streams is one of the limitations of this study. Third, another limitation of this study is the lack of verification of the conceptual framework derived from the literature that needs to be addressed in future research.
Practical implications
This study suggests a set of practical implications for industrial managers. First, the authors summarize the firms’ capabilities needed to develop and manage networks in one single source. Based on the conceptual framework of this study, firms can develop managerial tools, technological options, checklists and questionnaires and monitor the status of NC in their surrounding network on an ongoing basis. Second, the present research shows that some NCs are more repetitive than others, and therefore, it can be said that managers should pay more attention to them. The critical NCs, such as determining network position, determining network size, partner selection and attraction, coordination, inter-firm knowledge sharing and conflict resolution, are included in this study. Third, the argument of this study that both NDC and NMC should be considered simultaneously can be thought of as an important practical implication. Although in terms of the network life cycle, NDC is required earlier than NMC, these two capabilities should be used in parallel. Managers should not expect network development to be perfect and then network management to begin; therefore, NMCs should be used during network development.
Originality/value
Research shows that most network studies focus more on network antecedents and outputs and less on processes and related capabilities. Some researchers have made few efforts to identify and scrutinize capabilities related to business relationships. In this context, IMP scholars have introduced NC to emphasize the network perspective. Despite the considerable conceptual and empirical richness, the existence of different nomenclatures and theoretical diversity in NC literature has led to conceptual ambiguity and a lack of consensus on this crucial complex construct.
- Systematic review
- Network perspective
- Networking capability
- Network development capability
- Network management capability
Arasti, M. , Garousi Mokhtarzadeh, N. and Jafarpanah, I. (2022), "Networking capability: a systematic review of literature and future research agenda", Journal of Business & Industrial Marketing , Vol. 37 No. 1, pp. 160-179. https://doi.org/10.1108/JBIM-06-2020-0273
Emerald Publishing Limited
Copyright © 2021, Emerald Publishing Limited
Related articles
All feedback is valuable.
Please share your general feedback
Report an issue or find answers to frequently asked questions
Contact Customer Support
Help | Advanced Search
Computer Science > Software Engineering
Title: understanding web application workloads and their applications: systematic literature review and characterization.
Abstract: Web applications, accessible via web browsers over the Internet, facilitate complex functionalities without local software installation. In the context of web applications, a workload refers to the number of user requests sent by users or applications to the underlying system. Existing studies have leveraged web application workloads to achieve various objectives, such as workload prediction and auto-scaling. However, these studies are conducted in an ad hoc manner, lacking a systematic understanding of the characteristics of web application workloads. In this study, we first conduct a systematic literature review to identify and analyze existing studies leveraging web application workloads. Our analysis sheds light on their workload utilization, analysis techniques, and high-level objectives. We further systematically analyze the characteristics of the web application workloads identified in the literature review. Our analysis centers on characterizing these workloads at two distinct temporal granularities: daily and weekly. We successfully identify and categorize three daily and three weekly patterns within the workloads. By providing a statistical characterization of these workload patterns, our study highlights the uniqueness of each pattern, paving the way for the development of realistic workload generation and resource provisioning techniques that can benefit a range of applications and research areas.
Subjects: | Software Engineering (cs.SE) |
Cite as: | [cs.SE] |
(or [cs.SE] for this version) | |
Focus to learn more arXiv-issued DOI via DataCite |
Submission history
Access paper:.
- HTML (experimental)
- Other Formats

References & Citations
- Google Scholar
- Semantic Scholar
BibTeX formatted citation

Bibliographic and Citation Tools
Code, data and media associated with this article, recommenders and search tools.
- Institution
arXivLabs: experimental projects with community collaborators
arXivLabs is a framework that allows collaborators to develop and share new arXiv features directly on our website.
Both individuals and organizations that work with arXivLabs have embraced and accepted our values of openness, community, excellence, and user data privacy. arXiv is committed to these values and only works with partners that adhere to them.
Have an idea for a project that will add value for arXiv's community? Learn more about arXivLabs .
A Literature Review of the Urban Heat Island (UHI) Phenomenon Connected with Smart Cities Paradigm
New citation alert added.
This alert has been successfully added and will be sent to:
You will be notified whenever a record that you have chosen has been cited.
To manage your alert preferences, click on the button below.
New Citation Alert!
Please log in to your account
Information & Contributors
Bibliometrics & citations, view options, index terms.
Human-centered computing
Visualization
Visualization application domains
Geographic visualization
Information systems
Information systems applications
Decision support systems
Data analytics
Spatial-temporal systems
Geographic information systems
Recommendations
The manifold challenges for modeling the urban heat island.
Urban heat islands (UHIs) can have negative effects on cities. Under tropical climate and for large and high-dense mega cities like Singapore the elevated temperature levels yield to higher energy consumption for cooling, reduced outdoor thermal comfort,...
Urban planning and smart cities: interrelations and reciprocities
Smart cities are emerging fast and they introduce new practices and services which highly impact policy making and planning, while they co-exist with urban facilities. It is now needed to understand the smart city's contribution in the overall urban ...
Smart and Fermented Cities: An Approach to Placemaking in Urban Informatics
What makes a city meaningful to its residents? What attracts people to live in a city and to care for it? Today, we might see such questions as concerns for HCI, given the emerging agendas of smart and connected cities, IoT, and ubiquitous computing: ...
Information
Published in.

https://ror.org/00x27da85Department of Mathematics and Computer Science, University of Perugia, Perugia, Italy
School of Engineering, University of Basilicata, Potenza, Italy
Department of Civil and Environmental Engineering and Architecture, University of Cagliari, Cagliari, Italy
Faculty of Information Technology, Clayton Campus, Monash University, Clayton, VIC, Australia
Algoritmi Research Centre, University of Minho, Braga, Portugal
Department of Chemistry, Biology and Biotechnology, University of Perugia, Perugia, Italy
Springer-Verlag
Berlin, Heidelberg
Publication History
Author tags.
- Urban Heat Island
- Smart Cities
- Urban Planning
- Systematic Literature Review
- Sustainability
- City Dashboard
Contributors
Other metrics, bibliometrics, article metrics.
- 0 Total Citations
- 0 Total Downloads
- Downloads (Last 12 months) 0
- Downloads (Last 6 weeks) 0
View options
Login options.
Check if you have access through your login credentials or your institution to get full access on this article.
Full Access
Share this publication link.
Copying failed.
Share on social media
Affiliations, export citations.
- Please download or close your previous search result export first before starting a new bulk export. Preview is not available. By clicking download, a status dialog will open to start the export process. The process may take a few minutes but once it finishes a file will be downloadable from your browser. You may continue to browse the DL while the export process is in progress. Download
- Download citation
- Copy citation
We are preparing your search results for download ...
We will inform you here when the file is ready.
Your file of search results citations is now ready.
Your search export query has expired. Please try again.
Designing resilient supply chain networks: a systematic literature review of mitigation strategies
- Original - Survey or Exposition
- Open access
- Published: 18 September 2024
Cite this article
You have full access to this open access article
- Abdolreza Roshani ORCID: orcid.org/0000-0002-3067-0633 1 ,
- Philip Walker-Davies 3 &
- Glenn Parry ORCID: orcid.org/0000-0002-6432-2055 2
143 Accesses
Explore all metrics
With increased globalisation supply chain (SC) disruption significantly affects people, organisations and society. Supply chain network design (SCND) reduces the effects of disruption, employing mitigation strategies such as extra capacity and flexibility to make SCs resilient. Currently, no systematic literature review classifies mitigation strategies for SCND. This paper systematically reviews the literature on SCND, analysing proposed mitigation strategies and the methods used for their integration into quantitative models. First to understand the key failure drivers SCND literature is categorised using geography, with local, regional or global disruptions linked to vulnerable sections of a SC. Second, the strategies used in mathematical models to increase SC resilience are categorized as proactive, reactive, or SC design quality capabilities. Third, the relative performance of mitigation strategies is analysed to provide a comparison, identifying the most effective strategies in given contexts. Forth, mathematical modelling techniques used in resilient SCND are reviewed, identifying how strategies are integrated into quantitative models. Finally, gaps in knowledge, key research questions and future directions for researchers are described.
Avoid common mistakes on your manuscript.
1 Introduction
The 9/11 terror attacks, the 2011 Japanese tsunami, and the 2020 COVID-19 pandemic are events that significantly impacted supply chains [SCs] (Aldrighetti et al., 2021 ; Snyder et al., 2016 ; Suryawanshi & Dutta, 2022 ). Although the risk likelihood of these events is low, they significantly impact SC performance (Tang, 2006 ) and encourage design of resilient SCs that can adjust to disruption (Wieland & Durach, 2021 ). Supply Chain Network Design [SCND] is of interest to academics and practitioners as it decreases (increases) the cost (profit) of SCs (Simchi-Levi & Kaminsky, 2004 ) and effects performance and resilience (Shen, 2007 ). Resilience can be achieved through creating redundancy (Sheffi, 2005 ), including holding safety/emergency stocks, having multiple suppliers and low capacity utilization rates to hedge against disruptions. In the literature, different redundancies are modelled to create mitigation strategies (Hosseini et al., 2019a , 2019b ), hedging against disruption risk (Ivanov et al., 2017 ). Mitigation strategies have two subcategories, proactive and reactive, depending if their applied pre- or post-disruption (Elluru et al., 2019 ).
Supply chain disruption and resilience have been analysed using both qualitative and quantitative models. Empirical and conceptual qualitative models are commonly used to identify, assess, and manage risks of disruption (Hervani et al., 2022 ; Hosseini et al., 2019a , 2019b ). Quantitative models are applied to assess the impacts of disruptions on supply chains and to evaluate relevant mitigation strategies (Azad & Hassini, 2019 ; Snyder et al., 2016 ; Taleizadeh et al., 2022 ). Quantitative work integrates mitigation strategies into mathematical models to design resilient SCs [RSCs] (Abbasian et al., 2023 ; Aldrighetti et al., 2021 ; Kabadurmus & Erdogan, 2020 ). However, before integration several critical challenges must be addressed. First disruptive events may affect different parts of SCs. Thus, the failure parameters in the models must be adjusted to show the actual condition of SCs’ vulnerability. Second, the efficiency of resilience strategies differs with context. When modelling resilient SCND [RSCND] problems, identifying an optimum strategy needs information of the relative performance of mitigation strategies in specific context. Third, RSC design requires that modelling approaches integrate the appropriate mitigation strategies and define their characteristics.
To address these challenges, a systematic literature review (SLR) of quantitative models of SCND was undertaken, focusing on mitigation strategies, their relative performance and their integration into mathematical models. The SLR is a well-established method used in analysing literature, including supply chain resilience (Aldrighetti et al., 2021 ; Maharjan & Kato, 2022 ; Naghshineh & Carvalho, 2022 ). However, few literature reviews examine quantitative models of RSC design that consider strategic facility location and supplier selection decisions. Table 1 details the content of extant literature reviews articles; √, o and x imply the area is covered, partially covered, and not covered respectively.
Whilst this review has some overlap with references from previous work (Table 1 ), significant differences exist. To our knowledge, no published review classified mitigation strategies applied in designing SCs based on vulnerabilities, none categorises them into proactive, reactive and SC design quality groups, none identify the relative performance of mitigation strategies that improve SC resilience, and none identify methods to integrate mitigation strategies into quantitative models. This paper aims to advance research in these areas by answering the following research questions:
RQ1: What is the classification of disruptions based on their geographic scope and the part of SCs they affect?
RQ2: Focussing on vulnerable SC sections, what strategies have been introduced to improve resilience?
RQ3: Drawing on performance criteria from literature, how have these strategies improved the resilience of supply chains?
RQ4: How are resilience strategies integrated into mathematical models?
The remainder of this paper is organized as follows: Sect. 2 presents the research method. Section 3 addresses RQ1, Sect. 4 addresses RQ2 & 3, whilst Sect. 5 addresses Q4. Concluding remarks, managerial insights, and future research directions will follow in Sects. 6 .
2 SLR methodology
The SLR was undertaken in three stages, Fig. 1 (Tranfield et al., 2003 ). Stage one is process planning, in stage two the review process is undertaken and in stage 3 findings from analysis of the literature are reported, and each are now detailed.
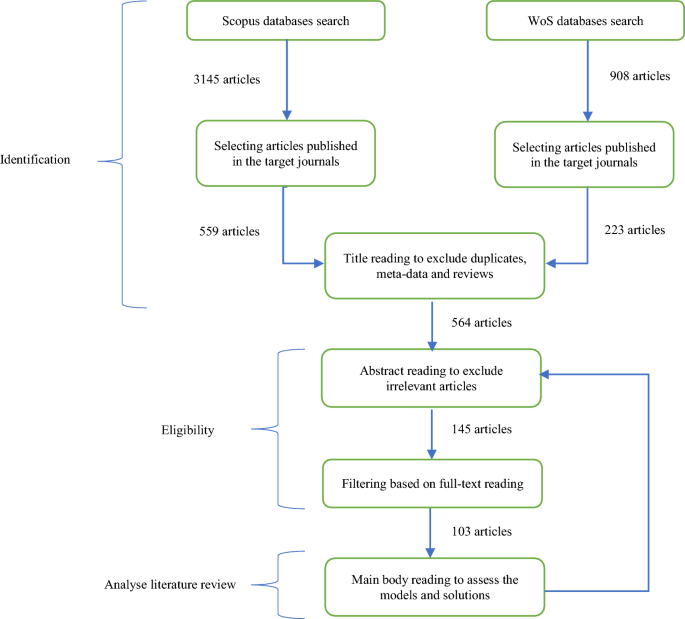
Literature review methodology. A flow diagram represents the process of searching selected keywords in Scopus and WoS databases, to find reference articles by excluding duplicates, reviews and irrelevant articles
2.1 Data source, inclusion, and exclusion criteria
Scopus and Web of Science (WoS) databases were selected as the most relevant sources, in accordance with previous reviews papers (Aldrighetti et al., 2021 ; Katsaliaki et al., 2021 ). The earliest articles on SC disruption and resilience were published the early 2000s, following the 9/11 Terrorist attacks (Katsaliaki et al., 2021 ), so the sample selection timeframe is restricted to 2001–2024. To ensure the inclusion of only top-tier research and industrial development, our analysis specifically targets articles published in prominent English-language journals. In this regard, we exclusively consider articles published in journals listed in the latest Chartered Association of Business Schools (CABS) 2021 ranking. This ranking incorporates multiple journal quality assessments, providing a reliable measure of research rigor and excellence. While journal rankings are inevitably subject to debate, the CABS ranking is widely recognized for its consistent and high standards of research quality (Kamal & Irani, 2014 ; Miemczyk & Johnsen, 2012 ; Rajagopal et al., 2017 ). Thus, in accordance with (Kamal & Irani, 2014 ; Rajagopal et al., 2017 ) only journals rated CABS 3, 4, and 4* are selected, and lower ranked and grey literature excluded (selected journals are listed in table i in supplementary materials).
Content criteria filters are employed in selecting articles to review. First, selected articles should be categorized as SC design problems. Second, only resilient decision-making [DM] problems with strategic and tactical time scales are considered. Third, papers must contain mathematical models, with binary variables used for selecting suppliers or opening facilities. Following Aldrighetti et al. ( 2021 ), we focus on SCND applied in industrial commercial sectors, excluding design of water, telecommunication and healthcare SCs. R-interdiction and fortification problems are also omitted since their goal is to select existing facilities to fortify, rather than relocating them (Liberatore et al., 2012 ; Starita & Paola Scaparra, 2021 ).
2.2 Retrieval strategy and review process
Following Saunders et al. ( 2009 ) the article retrieval strategy employed an iterative procedure of defining appropriate keywords, searching, analysing the literature and finalising results. Examination of research reviews on similar topics gave rise to two groups of keywords. The first relates to SCND and the second to resilience. The keyword combinations used to search given databases are provided in the supplementary material (table i).
The SLR starts (Fig. 1 ) with a keyword search on Scopus (3145 articles identified) and WoS (908 articles) databases, and was performed at the end of April 2024. Article titles were used to exclude duplicates. The abstract and main body of the paper were examined to exclude irrelevant papers and those not published in target journals, leaving 145 papers. Finally, the full-text was read and to avoid missing relevant papers, forward and backward snow balling methods performed. 103 articles were selected for final analysis (Supplementary Material Figure i shows number of articles published by year).
3 Definitions
To establish a clear and consistent understanding of terms related to supply chain disruption and resilience several researchers' definitions for these terms have been analysed. Through analysis and consideration of contexts of these definitions, we have sought to unify a comprehensive understanding. Five key terms were selected, which include "supply chain disruption," "supply chain resilience," "resilience or mitigation strategies," "proactive strategies," and "reactive or contingency strategies." The definitions and explanations of these terms have been thoroughly examined, and the resulting insights presented in Table 2 .
4 Finding from the content analysis
In this section we present the findings from the content analysis, which explore various aspects of supply chain disruptions and mitigation strategies. We investigate the types of disruptions and vulnerable sections in 4.1, followed by a detailed review of proposed mitigation strategies in designing supply chains in 4.2. Furthermore, we investigate the integration of resilience strategies into mathematical models in subSect. 4.3 , focusing on disruption-related parameters in 4.3.1 and the methodology for incorporating these strategies into the mathematical models in 4.3.2.
4.1 Type of disruption and supply chain vulnerable sections
To answer to RQ1, “what is the classification of disruptions based on their geographic scope and the part of SCs they affect?”, disruption events reported in the SCND literature were categorized based on their geographic affect: local, regional, or global (Sawik, 2013b , 2019 , 2014 ). Local disruptions are characterized by their confined impact, typically affecting specific facilities or locations within a supply chain. For instance, an illustrative example of a local disruption is the Philips microchip plant fire that occurred in New Mexico in 2000, which had repercussions limited to that particular facility. Researchers and scholars, such as (Norrman & Jansson, 2004 ), have explored and documented local disruptions extensively.
Moving beyond localized disruptions, regional disruptions encompass a broader scale, impacting multiple nodes and arcs within a particular geographic region. These disruptions can be caused by various events, including but not limited to regional labour strikes, logistical errors, and natural disasters like floods or earthquakes. The devastating Tohoku earthquake of 2011 (Park et al., 2013 ) and the unexpected 2018 UK KFC chicken shortage (Young & Bhattacharyya, 2020 ) are compelling instances of regional disruptions that affected different parts of the supply chain within their respective areas.
On a grander scale, global disruptions have the potential to disrupt entire supply chains, transcending regional and local boundaries. These disruptions are often caused by significant global events such as economic crises, labor strikes in the transportation sector, or catastrophic events like the Covid-19 pandemic in 2019 (Remko, 2020 ). The Covid-19 pandemic, in particular, had far-reaching consequences, profoundly impacting supply chains worldwide (Paul et al., 2021 ).
To organize the body of literature, Table 3 provides a comprehensive categorization based on the type of disruption and the corresponding decision-making (DM) problems studied. The DM problems are grouped into four main classes: resilient supplier selection (RSS), reliable facility location (RFL), resilient logistic network design (RLND), and integrated reliable facility location (IRFL). The table includes a summary column presenting the percentages of studies that address each DM problem based on the categories of disruptions, namely, global, regional, and local.
From the table, it becomes evident that the majority of the studies have focused on local disruptions, accounting for approximately 96.12% of the research. Regional disruptions constituted about 14.56% of the studies, while global disruptions represented 4.85% of the analysed articles (total exceeds 100% due to overlaps in the data). When dealing with global and regional disruptions, researchers have primarily concentrated on RSS and RLND, likely due to the broader and more severe impact these disruptions can have on the supply chain. In contrast, studies addressing local disruptions have often explored RLND and RFL, given the more contained scope of these disruptions.
Supply chain networks usually encompass multiple tiers, including suppliers, manufacturers (plants), distribution centres (warehouses or depots), retailers, and customers (demand zones). To represent this interconnected system, a general graph, G = (V, A), is employed, where V denotes the set of nodes representing the different facilities and customer zones dispersed across R disjoint geographic regions. The set of arcs, denoted by A, captures the various routes that connect these nodes, symbolizing the intricate flow of goods and information within the supply chain network. Table 3 summarizes the percentage of studies that have addressed specific vulnerable areas of the supply chain concerning the type of disruption. From the table, it is evident that researchers have primarily focused on studying the vulnerabilities of suppliers, which represents approximately 55.34% of the examined articles. Following closely, distribution centres have garnered significant attention, constituting 30.1% of the studies, while general facilities have been the subject of 29.13% of the analysed research.
4.2 Mitigation strategies
In this subsection, we provide a comprehensive exploration of different aspects related to the design and evaluation of supply chain mitigation strategies. Included is a thorough review of proposed mitigation strategies in designing supply chains (Sect. 4.2.1 ), an analysis of mitigation strategy combinations (Sect. 4.2.2 ), and an assessment of the relative performances of various mitigation strategies (Sect. 4.2.3 ).
4.2.1 Review of proposed mitigation strategies in designing supply chains
RQ2 asked “focussing on vulnerable SC sections, what strategies have been introduced to improve resilience?”. We identify SCs resilience strategies used for different vulnerable sections and examine their use in the design of SCs. Chowdhury and Quaddus ( 2017 ) define three groups of capabilities that determine the resilience of a SC against disruptions: (a) proactive capabilities, (b) reactive capabilities, and (c) SC design quality capabilities. Proactive capabilities, such as SC readiness and flexibility, help systems to recognise, anticipate and defend against the risk of disruption, reducing impact before it occurs. Reactive capabilities of SCs form from SC response and recovery. SC response is the capability of the system to mitigate the disruption in the shortest possible time and with smallest impact, while recovery capability is defined as the ability to rapidly return to a normal operational state (Pettit et al., 2013 ). SC design quality capability is determined by node density, complexity and critically (Chowdhury & Quaddus, 2017 ; Craighead et al., 2007a , 2007b ). The full list of 133 mitigation strategies from literature is in Table 8 , categorising resilient strategies based on specified vulnerable section i.e. suppliers, general facilities etc., and the three SC capabilities from Chowdhury and Quaddus ( 2017 ).
Proactive strategies are mainly applied to suppliers (PrasannaVenkatesan & Goh, 2016 ; Sawik, 2020 ), DCs (Gholami-Zanjani et al., 2021a ; Hasani & Khosrojerdi, 2016 ) and plant and manufacturers (Gholami-Zanjani et al., 2021b ; Hasani & Khosrojerdi, 2016 ). SC design quality strategies are used extensively for suppliers (Nooraie & Parast, 2016 ; Sawik, 2011 ), general facilities (Snyder & Daskin, 2005 ; Saha et al., 2023), DCs (Hasani & Khosrojerdi, 2016 ) and transportation (Ghavamifar et al., 2018 , Wang and Yao 2023). Reactive strategies are commonly applied to suppliers (Cheng et al., 2018 , Ghomi-Avili et al., 2021; Fattahi et al., 2020 ), DCs (Alikhani et al., 2021 ; Zhang et al., 2024 ), demand points (Hosseini et al., 2019a , 2019b ; Alikhani et al., 2023a , 2023b ), plant and manufacturers (Feng et al., 2023 ; Sabouhi et al., 2020 ) and general facilities (Egri et al., 2023 ; Xie et al., 2019 ).
Reviewing information summarised in Table 8 reveals a set of mitigation strategies commonly used to make SCs more robust and resilient. Table 4 lists those strategies applied to at least two different vulnerable sections of SCs. We have also assigned a code referring to each mitigation strategy in column one of Table 4 . The last column reports the percentage of reference articles that apply the respective strategy in their quantitative model. Table 4 contains eight proactive mitigation strategies (labelled P1–P8). P5 (11.65%), and P7 (9.71%) utilising reserve capacity , and P1 (11.65%) from SC readiness are the most commonly studied approaches in the sample. Though commonly used, these strategies may perform differently in different SCND contexts. For example, in designing a global supply chain (GSC) for an electro-medical device manufacturer, with the objective of maximising total net present value of the GSC after-tax profit, Hasani and Khosrojerdi ( 2016 ) applied P1 to protect suppliers, manufacturers, and warehouses against disruptions. They applied P7 in warehouses to deal with the finished product shortage resulting from capacity disruption of facilities in the upper tiers. They found that P1 significantly mitigated the risk of disruptions, while the efficiency of strategy P7 was not significant. In contrast, Rezapour et al. ( 2017 ) analysed the design of a resilient automotive parts manufacturer SC under competition to maximise total expected profit. They applied P5 to enable suppliers to increase their production capacities and P7 to allow retailers to hold emergency stock as a mitigation strategy. They showed that P7 is more efficient than P5 in both reducing profit variation and improving the SC worse case profit. Recently, Alikhani et al., ( 2023a , 2023b ) applied various combinations of mitigation strategies, including P1 and P7, to design resilient retail supply chains. They demonstrated that combining P1 with SD8 (see Table 8 ) yields superior results by generating synergistic effects among resilience strategies under budget limitations for supply chain resilience.
Table 4 lists nine reactive supply chain resilience strategies (R1 to R9) commonly used to hedge vulnerable sections of SCs against disruptions. The most frequently employed reactive strategies are R1 (28.15% of 103 reference articles), R2 (13.59% of 103 reference articles) and R3 (8.74% of 103 reference articles). The RSCND literature reveals that, similar to proactive strategies, the efficiency of this group of strategies is dependent on the nature of the SC. For example, Fattahi et al. ( 2017 ) addressed the design of a glass company SC for which the objective function is the minimisation of the total cost of SC network over a planning horizon. Based on several computational experiments, they concluded that R1 the most effective strategy in designing a resilient SC. In contrast Gholami-Zanjani et al. ( 2021a ) addressed a location-inventory problem in a food supply chain where the objective function is the expected total profit minus total strategic costs, andthey found R1 to be dominated by P1 and DR8 strategies.
Table 4 also contains seven mitigation strategies from the SC design quality category (D1 to D7). The most common SC design quality strategies in the academic papers are D2 (33%), D5 (19.42%), and D3 (16.50%) all from complexity group. A less complex SC has fewer nodes and/or fewer interconnections, so increased complexity is expected to create more vulnerabilities. However, additional nodes that create a buffer in the SC reduce vulnerability (Chowdhury & Quaddus, 2017 ). Adding additional nodes, D2 strategy, is found to be effective in comparison to a single sourcing strategy (Berger & Zeng, 2006 ). The efficiency of a D2 strategy has been compared to other mitigation strategies (see Hasani & Khosrojerdi, 2016 ; Sabouhi et al., 2020 ; Aldrighetti et al., 2023 ). The details of the performance comparison of this strategy is given in the next subsection. Strategy D5 is used to increase the reliability of the system in the event of a failure at a facility, and has mainly been applied in RFL models. Each customer is assigned to r closest facilities such that if their primary facility is disrupted their order is met by the next facility and so on. We found no evidence of any comparative performance analysis of D5 against other resilience strategies in the literature. Strategy D3 has been used in the design of many different RSCs (Jabbarzadeh et al., 2018 , Wang & Yao, 2023). Literature indicates that D3’s performance depends on SC context. For example, Sabouhi et al. ( 2020 ) applied several mitigation strategies including D3 to the design of an industrial paint manufacturer SC where suppliers, factories, DCs and routes are at risk of disruption. They found D3 was the most efficient mitigation strategy to minimise total cost.
4.2.2 Mitigation strategy combinations
In the previous subsection, to answer RQ2, the proposed mitigation strategies in the reference articles were categorized into three subcategories and the most common strategies were analysed. In this subsection, building upon the analysis already conducted, our objective is to specify the strategies introduced in each article and explain how they are combined in the proposed mathematical models in reference articles.
Table 9 lists the resilience strategies used in the mathematical models proposed in each reference article in designing RSCs. Reviewing the information summarized in Table 9 reveals that resilience strategies have been used both singularly and in combination to construct the proposed mathematical models. Table 5 displays the number of reference articles that have utilized a subset of strategies in each group in designing their mathematical models. The models have been categorized into two groups: those that applied single resilience strategies and those that combined resilience strategies.
In Table 5 , the models that have employed only one strategy are categorized into three groups based on the capabilities of the supply chain. However, none of these models presented the use of purely proactive strategies. Among the models discussed, eight utilized single reactive strategies, six of which applied FR1 (reassignment of customers to surviving facilities). The remaining models in this category employed one of FR2 (customers rerouting until receiving a service), or FR5 (reassignment of demand nodes to r closer facilities (level-r)) from reactive strategies. In addition, single strategies from SC design quality were employed in 21 articles. Specifically, FD1 (assignment of demand nodes to the closest facilities) was applied in 13 articles, while SD3, DD3, FD2, and RD3, and SD6 were applied in three, two, one, one, and one time respectively.
Table 5 lists possible combinations of different sets of resilience strategies and the number of reference articles that applied such combinations. Among these articles, some only combine strategies selected from the same category, with researchers predominantly focusing on combining more reactive strategies compared to other types.
Furthermore, several researchers have attempted to apply combined strategies selected from different categories. Among these studies, a larger number of authors applied (1) a combination of strategies selected from all categories, and (2) a combination of strategies selected from both reactive and SC design quality categories, with 23 articles referencing each approach.
Strategies such as SD3 (Using dual or multiple sourcing) and SD4 (contracting with (reliable or unreliable) recovery or backup suppliers) from the SC design quality category, as well as SR4 (customers’ demand reallocation or recalculation of purchase or shipment from primary or backup suppliers), and DR6 (recalculating inventory position), DR3 (recalculating the amount of products transferred from DCs) and MR1 (recalculating the amount of lost sales or unmet demand to apply penalties) from the reactive strategies category, were frequently combined in the design of RSCNs. Additionally, strategies such as SP1 and FP1 (protection or fortification), SP10 and DP7 (pre-positioned inventory & holding safety and emergency stocks), and SP8 and PP8 (additional extra production capacity) and DP6 (Adding extra inventory capacity) were among the strategies that were combined more often than others.
Researchers have explored the potential to enhance supply chain resilience by combining strategies and applying them simultaneously. For instance, Alikhani et al., ( 2023a , 2023b ) uses a multi-method approach that integrates analytical modeling and qualitative theory development to propose a framework for selecting the optimal set of resilience strategies for SCND problems. Their approach includes a two-stage stochastic programming model to design a resilient network for a three-echelon RSC comprising multiple suppliers, DCs, and retail stores. The model aims to select the best resilience strategies to maximize their synergistic effects while minimizing the fixed and operational costs of SCND. Considering different vulnerable sections within a supply chain is another reason for employing a combination of resilience strategies in its design. For example, in their study Jabbarzadeh et al. ( 2018 ), introduce a model to design a three echolnes RSC that accounts for random disruptions at both suppliers and factories by employing resilience strategies, such as 'contracting with backup suppliers and facilities,' 'multiple sourcing,' and 'adding extra supply/production capacities'. In another study, Sabouhi et al. ( 2020 ) addressed the problem of designing a four-echelon RSC (Resilient Supply Chain) in which suppliers, factories, DCs (Distribution Centers), and transportation routes were identified as vulnerable sections of the supply chain. In this study, the authors aimed to combine different resilience strategies to hedge the supply chain against disruptions. Some of the mitigation strategies are interrelated, and implementing one strategy from a particular group may necessitate the application of another strategy from a different group. For instance, SP10 and DP7 from the proactive strategies category, along with SR3 from the reactive strategies category can be synergistically applied together. Strategies like SD3 and SD4 have been integrated with various strategies from diverse groups (see Table 9 ). Various reference articles have examined the efficiency of several resilience strategies in enhancing the resilience of a supply chain (see Sect. 4.2.3 ) without providing justification for the combination of these strategies (see for example: Fattahi et al., 2017 ; Azad & Hassini, 2019 ).
4.2.3 Mitigation strategies relative performances
One of the challenges faced in SCND is deciding on the most effective mitigation strategy. RQ3 asks “how have these strategies improved the resilience of supply chains?”, and draws on relative performance of mitigation strategies literature. In the previous section, several mitigation strategies were identified place into three categories: proactive, reactive and SC design quality. However, few studies analyse and compare mitigation strategy application. In our literature review we identify 21 strategies where comparative analysis is reported.
The results in Table 6 show: (1) The most common strategies, D2 and P1, often out-perform other strategies regardless of industrial application (Fattahi et al. ( 2017 ) provide an exception). (2) The relative performance of mitigation strategies is related to the objective function (see D3, P3 in Kamalahmadi et al. ( 2022 ), D2 and single sourcing in Sawik ( 2013b )). (3) Combined strategies often outperform single applications (see D2, D3, P5, and D5 in Jabbarzadeh et al. ( 2018 ) and SD8, P1, SR10, P7 in Alikhani et al., ( 2023a , 2023b )). (4) The most commonly employed strategies, identified in Table 4 , do not necessarily provide the best performance in a given context (see SP9, SP10, D2, P5 in Yoon et al. ( 2018 ) and D2, D3, P1, and R9 in Aldrighetti et al. ( 2023 )).
4.3 Integration of resilience strategies into mathematical models
This section aims to show how resilience strategies are integrated into mathematical models by analysing: (1) decision making environments and proposed modelling approaches; and (2) the main characteristics of the mathematical models used for common strategies.
4.3.1 Disruption related parameters
In developing mathematical models for RSCND, decision making environment play an essential role, specifying how failure probabilities, and disruption scenarios are defined. In this article, decision making environments are categorised into three groups in accordance with Rosenhead et al. (1972): certainty, risk, and uncertainty situations (see table iv in the supplementary material). Certainty situation (DET) involves models in which all parameters are deterministic and known. DET models do not include any disruption related parameters and are used to investigate the impact of disruptions events on SCs for each pre-defined disruption scenario separately (Kungwalsong et al., 2022 ). Models designed for supply chains known to be at risk of disruption (RSK) containing parameters where exact risk values aren’t known, but have known probability distributions (Snyder et al., 2006 ). In this paper, we categorise RSK models into five sub categories (the first three categories are from Snyder et al. ( 2016 )): implicit functions (IF), reliable backup (RB), scenario-based (SB), stochastic programming (SP), and reliability and risk based (R&R) models. In IF modelling methods, three different categories of failure parameters are used. Facilities are often given identical local disruption probabilities to make modelling easier (Albareda-Sambola et al., 2017 ; Alcaraz et al., 2015 ; Snyder & Daskin, 2005 ; Yun et al., 2015 ; Zhang et al., 2016 ). However, this approach is not representative of practice (Aboolian et al., 2013 ; Cui et al., 2010 ). To better simulate reality, researchers define site- or facility-dependent failure probabilities for local independent failure modes (Albareda-Sambola et al., 2015 ; Berman et al., 2007 ; Yu & Zhang, 2018 ; Yun et al., 2020 ). Another approach is to remove the facility failure independence hypothesis and define failures as correlated disruption probabilities (Li et al., 2013a , 2013b , 2013c ; Xie et al., 2019 ). In RB models, as with some IF models, facility failure probability is considered as facility-dependent (Benyoucef et al., 2013 ; Lim et al., 2010 ). In IF and RB modelling facility failure probabilities are implicitly imposed and the decision process is not divided into pre- and post-disruption phases (Lu et al., 2015 ). For this reason, IF and RB have not been applied for modelling reactive strategies, however, used to apply several proactive and SC design quality strategies (Azad et al., 2019 ; Lim et al., 2010 ). SB models presented in reference articles fall into the class of two-stage programming models. In these models, the first-stage decisions are made prior to realizing any stochastic event (e.g. facilities disruptions) while the second-stage decisions are made after the uncertainty is revealed as a set of disruption scenarios. The probability for each scenario is calculated independently, taking into account the probability of facility disruption, which may be global, regional, or local (Sawik, 2011 , 2013a , 2017 ). SB aims to optimise the first-stage objective function and the expected value of the random second-stage objective across all possible disruption scenarios. SB is one of the most widely applied modelling approaches in designing RSCs (see Table iv in supplementary materials) and have been successfully applied to model proactive, reactive, and SC design quality strategies (Alikhani, Torabi, and Altay 2021). However, one of the main limitations of SB models is that when the number of scenarios increases, the problem size increases exponentially making solving the problem difficult and in some cases impossible (Sabouhi et al., 2020 ). In SP models, some model’s parameters (such as demand and lead-time) follow specified statistical distributions (Saputro et al., 2021 ). Facility failure parameters in SP models are defined as the disruption frequency rate and disruption downtime rate (Firouz et al., 2017 ). SC design quality such as multiple sourcing and inventory control (Yoon et al., 2018 ) and proactive strategies (Saputro et al., 2021 ) are among the mitigations strategies that have been integrated into SP models. R&R models are used to either minimise risk in the entire SC, or maximise reliability. In Ravindran et al. ( 2010 ), each facility is associated with two risk types; value-at-risk (VaR) and miss-the-target (MtT). The risk of selecting a supplier or opening a facility is defined as a value between 0 and 1 (Kaur and Prakash Singh 2021; Yoon et al., 2018 ). Some researchers (Yildiz et al., 2016 ) aim to maximise network reliability by assigning each node and arc a given reliability index. The SCND objective function is first to minimise the total cost and second to maximise total reliability.
In models defined under uncertainty situations (UCT), parameters are uncertain or vague and no information about the probabilities is known. In this paper, we categorise UCT models into two subcategories: robust (RO) and Fuzzy (FUZZ) models. In RO models, parameters are uncertain and no information about probabilities are known. Similar to SB methods, RO models have been successfully applied to model proactive, reactive, and SC design quality strategies (An et al., 2014 ; Cheng et al., 2021 ; Hernandez et al., 2014 ; Lu et al., 2015 ). The main advantage of RO models is that they do not rely on probability distributions or the generation of scenarios (Cheng et al., 2021 ). A solution to an RO model is defined either as solution robust or model robust. A solution robust remains ‘close’ to optimal for all scenarios of the input data, and a model robust solution remains ‘almost’ feasible for all data scenarios (Jabbarzadeh et al., 2014). The RO model aims to measure trade-offs between solution and model robustness (Lu & Cheng, 2021 ). FUZ models are used when some critical parameters (such as demand and capacity levels) are imprecise in nature due to incompleteness and/or unavailability of data (Torabi & Hassani, 2008). Torabi et al. ( 2015 ) proposed a fuzzy enhanced possibilistic programming approach to deal with epistemic uncertainty in input data such as costs, demands, and number of returned products. The scenario-based method including scenario dependent failure probabilities is applied to define the possible disruption of facilities. They integrated proactive, reactive and SC design quality strategies in their model.
4.3.2 Integrating resilience strategies into the mathematical models
In this subsection, we show how mitigation strategies have been integrated into the mathematical models by analysing the characteristics of the mathematical models employing most efficient and frequently applied strategies in the models in the references articles; these include P1 from proactive resilience strategies, R1 from reactive strategies and D2 and D5 from SC design complexity strategies. Tables 7 summarize the information related to proactive, reactive and SC design strategies, respectively. A review of the characteristic of the models listed in Table 7 shows that RB (Jabbarzadeh et al., 2016 ; Li et al., 2013a , 2013b , 2013c ), SB (Aldrighetti et al., 2023 ; Alikhani et al., 2021 ; Gholami-Zanjani, et al., 2021a ; Sawik, 2013a ; Torabi et al., 2015 ; Zhang et al., 2024 ) and RO (Aksen & Aras, 2012 ; Hasani & Khosrojerdi, 2016 ) models have been applied to model P1 strategy. For example, Aldrighetti et al. ( 2023 ) propose that facilities can be reinforced through investments in protection systems. This resilience investment is quantified as a percentage of the standard facility establishment costs and is categorized into various protection levels. Each level corresponds to a reduction in disruption magnitude. In these models, non-scenario dependent binary variables are used as the dominant variables in modelling. As an exception, Gholami-Zanjani et al. ( 2021a ) employ the fortification of facilities as a continuous function based on investment costs, which can be adjusted at any time period under each disruption scenario. The facilities which are protected in case of disruption either do not fail (Aksen & Aras, 2012 ; Li et al., 2013a , 2013b , 2013c ; Sawik, 2013a ), or lose capacity according to their fortification level or the amount of investment (Gholami-Zanjani, et al., 2021a ). In the implementation of this strategy, the protection cost is minimized as the objective function and the amount of investment may be limited to available budget (Hasani & Khosrojerdi, 2016 ; Jabbarzadeh et al., 2016 ; Zhang et al., 2024 ).
Table 7 also presents the main characteristics of the mathematical models that applied R1 strategy as a post disruption scenario. As reported in Table 7 , SB (e.g. Hosseini et al., 2019a , 2019b ; Fahimnia & Jabbarzadeh, 2016; Ghanei et al., 2023 ), RO (e.g. Alikhani et al., 2023a , 2023b ; Hasani & Khosrojerdi, 2016 ), DET (Maliki et al., 2022 ), and FUZ (Namdar et al., 2021 ) models have been applied to model R1. A review of the decision variables used in modelling R1 shows that they are defined as a scenario dependent integer or continuous variables that determine the number of products sent to customers or other facilities in any disruption scenario. Transportation costs, selling price, unit purchasing cost of materials, distance between facilities and customers, and expected defect rate of products supplied by each supplier are related parameters that makes it possible to optimise the integration of this strategy by taking into account the objective function (column 4, Table 7 ). Capacity at each facility is the most commonly applied constraint in models and scenario-based modelling is the dominant method in this strategy.
SC design quality strategies are used before disruptions, increasing resilience and robustness. To explore how such strategies are integrated into mathematical models focus was placed on the two strategies identified as most efficient in research articles, D2 and D5 (see Sect. 4.2 ). Table 7 shows the characteristics of the mathematical models applying these strategies. IF (Snyder & Daskin, 2005 ; Chen et al., 2011; PrasannaVenkatesan & Goh, 2016 , Enayati et al., 2024 ) and SB (Dupont et al., 2018 ; Feng et al., 2023 ; Sawik, 2020 ) are among the modelling approaches being applied to model D2, and D5. The goal of strategy D2 is to reduce the potential effects of disruptions on the SC by selecting the best combination of suppliers to allocated customer demand. To include this strategy in mathematical models, similar to proactive strategies, non-scenario dependent binary variables are used for supplier selection (column 2 of Table 7 ). Continuous variables, such as ‘Fraction of total demand assigned to each supplier’ determine demand attributed to suppliers (PrasannaVenkatesan & Goh, 2016 ; Sawik, 2014 , 2020 ). Supplier capacity and demand satisfaction constraints are key limitations frequently used to regulate this strategy. IF is the only modeling approach used for D5, defined by binary variables such as 'assigning customers to a facility at different levels,' or continuous variables like 'the probability that a facility is assigned a customer at different levels' (see column two of Table 7 for the full list of variables). The objective function defined for modelling this strategy mainly includes the minimisation of the expected transportation cost. In some of the presented models, assignment of customers to the facilities is limited by their capacity. The mathematical formulation for this strategy must include constraints such as 'each customer can only be assigned to one facility at each assignment level' and 'customers must be assigned to facilities that have been opened.' IF models are not applicable in modelling reactive strategies since they do not explicitly define failure scenarios. However, unlike SB models that can exponentially increase numbers of variables and constraints, IF models are more compact, polynomial in size, so produce solutions more quickly (Cui et al., 2010 ).
5 Future research directions
5.1 disruption types.
The Covid-19 pandemic demonstrated how global disruption can cause all sections of a SC to fail simultaneously. These types of disruptions in supply chain networks can be studied either separately or in an integrated manner. Scenario-based (SB) and robust optimization (RO) approaches have the potential to model these disruptions on a semi-global or global scale. However, only a few studies have investigated these scenarios, whether separately or in an integrated manner. Integrated global or semi-global disruption types, alongside regional ones, are often addressed in RSS problems; however, designing RLND networks demands even greater attention. Furthermore, studies focusing on RFL and IRFL problems that integrate such disruption events are also limited (Table 3 ) meaning further research in this area is required in future studies. In a multi-period stochastic setting, the accommodation of multiple disruptions occurring in succession during the recovery process in all decision-making problems addressed by SCND has not been studied. By incorporating this capability into the expanded framework, a deeper analysis of the sequential disruptions and their cumulative effects on the supply chain can be conducted (Azad & Hassini, 2019 ; Sawik, 2021 ). It is essential to recognize that different types of disruptions can have varying impacts on different regions. The effects of disruptions can be heterogeneous, with some regions experiencing more severe consequences than others, so insights into the relationship between supply chain resilience and robustness require exploration of such situations (Fahimnia et al., 2018 ).
5.2 Vulnerable sections
Our analysis revealed researchers focus on suppliers and plants as vulnerable sections of SCs. In retail SCs distributors and retailers are at risk of disruption, and this area warrants further attention. Moreover, in much of the analyzed research, it is assumed that customer demand remains independent of supply chain disruptions. However, during regional or global disruptions, simultaneous changes in customer demand are likely to occur, as evidenced by the increased demand for essentials like pasta and rice during the Covid-19 pandemic. This issue deserves particular attention in the context of RSS, IRFL, and RFL problems.
The possibility of local, regional or global disruptions occurring in the transportation routes or modes in RFL and RSS problems has not been considered until now and should be addressed in future research. Disruption in SC transportation sectors significantly impact on performance since meeting customer demand for physical products is only possible when this sector is operative. Future research should address disruption at transportation nodes and the implications of port congestion as a significant source of delays on supply chain performance (Namdar et al., 2021 ). This entails exploring mitigation strategies, operational adjustments, and decision-making frameworks to enhance resilience in the face of such disruptions. For instance, studying the coordination and synchronization of port operations, or optimizing resource allocation can help alleviate the negative impact of port congestion on supply chains.
Finally, the impact of facility disruptions on reachability and access distances presents an intriguing avenue for future research (Yan & Ji, 2020). Disruption, reachability and access distances opens opportunities to explore integrated RSCND problems, along with other optimization problems such as routing optimization under disruption scenarios. A comprehensive understanding of the performance of supply chain networks, requires consideration of the wider effects of disruptions, including the impact of disruptions on critical factors such as lead time or capacity of different sections of SCs (Hasani & Khosrojerdi, 2016 ).
5.3 Mitigation strategies
Suggestions for future research in this section are made by comparing the results obtained in this research and the aspects of the triple capabilities presented in Chowdhury and Quaddus (2017).
5.3.1 Proactive strategies
Proactive strategies related to disaster readiness capabilities of SCs including readiness resource, disruption detection, and security, is under researched. Specifically, when considering digital technologies now central to all supply chains in practice, greater focus needs to be placed upon cyber attacks and proactive cybersecurity strategies within the models. Our analysis in Table 8 reveals that only one study has proposed a cybersecurity strategy in modelling RLND problems. This strategy deserves greater attention, and incorporating this into future models across all types of resilient supply chain network design problems will advance understanding.
Few studies consider product substitution in RFL and assign flexibility indices to facilities in RLND problems. Exploring other types of flexibility strategies, such as time flexibility (the ability to adjust production lead times) and production volume flexibility, could open new avenues for future research. Flexibility in the workforce, products and production has not been considered too.
Efficiency gains from increased productivity and hardworking employees, and quality control are among the SC proactive capabilities that can be used as the basis of improving the resiliency of SCs by introducing suitable mitigation strategies. Another proactive strategy that requires more attention is the application of insurance as a mitigation strategy. Investigating the impact of insurance on supply chain resilience and developing insurance strategies could yield valuable insights.
A review of the mitigation strategies listed in the Table 8 shows that the application of proactive strategies for different vulnerable sections of SCs have not been equal. For suppliers, plants and manufacturers, and DCs, more than 10% of the reference articles applied proactive strategies, while for general facilities, retailers, and the transportation section, this number is reduced to below 5%. Proactive strategies have not been employed for geographically defined areas of customer demand. Future studies should investigate the effectiveness of proactive strategies for vulnerable SC sectors that have received little attention. In particular, future studies should explore approaches to enhance the robustness and resilience of arcs and transportation routes (Meng et al., 2021 ). This may involve evaluating alternative routes, and optimizing resource allocation for route protection, or implementing real-time monitoring systems to detect and respond to disruptions promptly. In addition, collaboration has been limited to suppliers, facilities, and DCs but it is increasingly recognized that the involvement and support of all supply chain partners are critical to the success of the proposed strategy. To address capability maintenance and control challenges, it is advisable to focus future efforts on the development of a comprehensive monitoring framework. This framework can leverage potential solutions from recent advancements in Internet-based technologies, including the Internet of Things (IoT), blockchain technology, artificial intelligence, and other related fields (Vishnu et al., 2021).
5.3.2 Reactive strategies
A number of reactive strategies have been proposed to increase the response capability of SCs (e.g., Alikhani et al., 2021 ; Sawik, 2013a , 2019 , 2021 ; Tucker et al., 2020 ). However, several research avenues remain in this area. First, by replenishing capacity through non-disrupted facilities and maintaining customer allocations, companies can effectively navigate challenges and maintain operational capabilities (Lu & Cheng, 2021 ). Secondly, in the event of disruptions, the availability of parts in the inventory for transhipment can be compromised (Sawik, 2019 ). To address these issues, analysis is required of contingency plans that can effectively deal with part non-availability for transhipment and constraints on the transhipment process. The analysis provided in Table 8 indicates that limited research has been conducted on applying reactive strategies to increase resilience in general facilities, retailers, transportation modes, routes, and customer zones within supply chains. More exploration is needed to understand how reactive strategies can be tailored and implemented at the facility level, within retail operations, for transportation modes and routes, and in customer zones. The concept of relocating DCs has recently been examined by Maliki et al. ( 2022 ) within the scope of dynamic RFL problems. Their sensitivity analysis revealed that utilizing mobile facilities can result in cost savings by eliminating the extra expenses of opening and closing facilities associated with dynamic relocations during each period of a finite planning horizon. Further exploration is necessary to determine if similar cost savings and operational efficiencies can be achieved within the context of other types of reverse supply chain network design (RSCND) problems, such as reverse logistics network design (RLND) and integrated reverse flow logistics (IRFL) problems.
Limited research has been done in the field of recovery related strategies. This group of strategies has only been applied where suppliers, manufacturers, DCs are the vulnerable sections of SC. Recovery strategies have not been examined in other sections defined as vulnerable and could be examined in future research.
5.3.3 SC design quality:
SC design quality related strategies are most often employed to improve the complexity related capabilities of SCs when suppliers, general facilities, plants, DCs and retailers are vulnerable to disruption. The greatest attention is given to strategies at transportation modes and routes, and customer demand disruptions. There is a knowledge gap related to capabilities that further improve resilience, such as SC node density, critically for suppliers, general facilities, plants, DCs and retailers, customer demand, and complexity for transportation modes and routes. Strategies can then be devised to enhance the robustness and redundancy of these critical nodes, ensuring uninterrupted flow of materials and minimizing disruptions. There is a notable lack of studies focusing on modeling facility segregation and dispersion strategies, despite their critical importance, particularly in addressing vulnerabilities among manufacturers, DCs, and retailers within supply chains (see Table 8 ). Currently, there is a lack of studies addressing mitigation strategies that prioritize the resilience of demand nodes. Future research could explore the advantages of having multiple buyers instead of relying heavily on a few large buyers. Another compelling area for further investigation is comparing strategies in concentrated markets versus diversified markets within different node density groups.
5.3.4 Mitigation strategy combinations
Several authors have attempted to integrate diverse mitigation strategies from various categories. However, it is evident that only one paper (Alikhani et al., 2023a , 2023b ) provides justifications for these integrations, specifically studying the synergistic effects of combining resilience strategies. A promising direction for future research entails conducting a qualitative study to delve into the underlying reasons behind these combinations, complemented by a quantitative analysis of their overall performance.
When combining resilience strategies, only a limited number of studies have explored the integration of supply chain design quality resilience strategies. Moreover, there is a scarcity of studies that combine proactive and reactive strategies. Therefore, in the future, authors can focus their efforts on proposing models that leverage the advantages of combining such strategies and investigate both their potential benefits and drawbacks. For example, among these strategies listed in Table 8 , facility collaboration (SP11, PP10, and DP9), cybersecurity (SP4, and DP3)—proactive approaches—and facility dispersion (SD2, PD1, and DD1)—a SC design quality approach—have shown promising results when integrated with other proactive or reactive strategies. Their integration can also be studied alongside approaches from supply chain design quality and reactive strategies to explore their effectiveness in enhancing supply chain resilience.
5.4 Mitigation strategies relative performance
The results of the analysis presented (Sect. 4.2 ) shows that in most research the effectiveness of strategies and their relative performance has not been investigated, or is only compared against a SC model not employing resilience strategies. Future research should focus on the relative effectiveness of different strategic options. The findings in Sect. 4.2 show that the performance of resilience strategies can depend on objective functions. The efficiency of strategies with both monetary and non-monetary objective functions such as visibility, responsiveness, social and environmental performance measures should be considered. Very little research exists where models are defined as multi-objective.
Exploration of the relative performance of mitigation strategies in different contexts, taking into account various objective functions, is required to develop knowledge in this area. Such studies will contribute to developing standardized approaches for selecting mitigation strategies tailored to specific types of supply chains.
5.5 Modelling
First, in multi-objective models presented using IF, RB, SSO, RO and SP, the objective functions are defined to minimise SC disruption costs, where usually the disruption cost is defined as the expected cost of transportation. Going forward researchers should consider non-monetary objective functions such as environmental related criteria and green transportation (Erdoĝan & Miller-Hooks, 2012 ) as well as social criteria such as fairness (Jiang & Zografos, 2021 ). Taking into account a longer time horizon, it is valuable to explore the enhancement of visibility, agility, external flexibility, and integral integration through the utilization of objective functions, such as maximizing visibility and internal integration (Nooraie et al., 2020). Exploring the trade-offs between sustainability and resilience can provide valuable insights for businesses in making tactical and operational decisions, such as determining sales prices, discounts, and customer service levels (Jabbarzadeh et al., 2018 ).
Secondly, the majority of models focus on designing resilient supply chains (RSCs) for a single product and within a single time period. However, there is a need for the development of resilience strategies and quantitative models that address multi-period and multi-product problems. Multi-period models serve as a basis for studying the ripple effects (Dolgui et al., 2018 ; Gholami-Zanjani, et al., 2021a ) and recovery options in supply chain design. Additionally, these models provide a foundation for analysing lead-time constraints, delays, and late orders in supply chain analysis (Kaur & Prakash Singh, 2021). To increase the realism and applicability of multi-period models across diverse industries, it is essential to incorporate seasonal products and consider factors such as inflation and the time value of money (Saha et al., 2020).
Third, few integrative models exist, and research could consider combining location problems with transportation planning, inventory management, and production scheduling. Integrating optimization problems across different planning horizons may prove an effective approach to system design.
Finally, the application of the developed model to real-life case studies within actual supply chains is crucial for advancing knowledge in the field. By conducting empirical studies in real-world contexts, researchers can refine the model, address practical considerations, and provide valuable guidance for industry practitioners seeking to apply the resilience strategies in their RSCND processes.
6 Conclusions, and managerial implications
6.1 summary and conclusions.
Events such as the Covid-19 pandemic highlighted the importance of resilience in SC design. Resilience strategies are key to protecting SC’s through planning for pre- or post-disruption activities. This novel investigation used a systematic literature review method to investigate the mitigation strategies used in mathematical models of RSCND problems and the methods of integrating them in the proposed models to address several challenges in developing quantitative models for designing RSCs.
To address RQ1, ‘What is the classification of disruptions according to the extent of their impact on vulnerable parts of SCs?’ we classified disruptions events based on their geographic scope and the part of SCs they affect, and show that in most articles local disruptions are introduced as the main cause of facility failures, with fewer articles considering regional and global disruptions respectively. When addressing local disruptions, researchers more often considered RLND and RFL problems than RSS and RFL problems. Analysis shows that in the literature, little attention has been paid to the modelling of RSCND problems in the case of national and regional disruption events.
RQ2 asked “focussing on vulnerable SC sections, what strategies have been introduced to improve resilience?” This analysis found that SC design complexity related strategies are most commonly used to address vulnerabilities at suppliers, with general facilities and transportation also often used respectively. Reactive strategies are dominant in the other SC sections, except for retailers where proactive strategies are prevalent. This analysis also examines the performance of mitigation strategies in different SCND contexts by reviewing the efficiency of the most commonly employed strategies selected from proactive, reactive and SC chain quality categories. The results of this analysis show that the effectiveness of mitigation strategies in reducing the effects of disruption depends on the context of SCs.
RQ3 ‘Drawing on performance criteria from literature, how have these strategies improved the resilience of supply chains?’, required analyses of the performance of applied mitigation strategies in improving the resilience of SCs. Strategies D2 and P1 performed well in comparison to other strategies, regardless of context of industrial application, though there were exceptions (see for example (Fattahi et al., 2017 ). We found the relative performance of mitigation strategies related to the objective function, and that combined strategies often outperform discrete applications.
Finally, RQ4 asked ‘How are resilience strategies integrated into mathematical models?’. To address RO4, the proposed mathematical models that addressed RSCND were classified based on their modelling approaches. Our findings indicated that SB and RO modelling approaches from the STH category were the only methods used to model a wide range of disruptive events and resilience strategies. Reactive strategies can only be integrated into mathematical models such as SB and RO, which divide the problem solving process into two stages, pre- and post-disruption. IF and RB modelling approaches were mainly used for proactive and SC design quality strategies. SP and FUZ models were used in modelling RSCND with parameters following specified statistical distributions and fuzzy numbers respectively, while R&R models were applied to risk minimisation and reliability maximization strategies.
The findings of this review provide a basis for both academics and practitioners to utilise and undertake further research into the methods of integration of resilience strategies into mathematical models proposed for different version of RSCND problems to make further effective contributions to the field.
6.2 Managerial implications
This review paper provides several implications for managers, particularly in the design of RSCs. The review guides managers in the design of mathematical models, and in choosing among mitigation strategies for SCs. For example, in a scenario a manager intends to invest in a food SC in Cornwall, a coastal areas in the UK that is exposed to natural disasters such as floods and storms. The manager's goal is to design a SC that includes suppliers, manufacturers, and distribution centres, with suppliers and manufacturers located in the Cornwall region and therefore vulnerable to disruption events. The analysis performed in Sect. 3 as a first step will assist the manager to address the appropriate optimization problem considering the identified vulnerable sectors of the SC. In addition, the analysis presented will help them to understand how to model the disruptions in terms of geographic scope. In this case, a RLND problem may be defined considering the possibilities of regional and local disruption affecting both suppliers and manufacturers. The second implication is that managers can identify resilient strategies to hedge SC’s against the possible disruptions. The analysis in Sect. 4.1 aids managers evaluation of resilience strategies proposed for given conditions they face. Further the analyses in both Sects. 4.1 and 4.2 can be used to compare the performance of mitigation strategies in a given context, supporting the choice of the most efficient strategy. Suppose P1 strategy is selected from the proactive category, which according to the analysis of Sect. 4.2 provides reasonable relative performance. The final implication is related to the design of a suitable mathematical model to solve this problem. The results of the investigations carried out in Sect. 5.1 then aid managers choice of modelling approach. Supplementing the choice of model, the main characterises of the model can be determined by referring to the analyses in Sect. 5.2 .
Data availability
Data supporting the findings of this study are available on a reasonable request from the authors.
Abbasian, M., Sazvar, Z., & Mohammadisiahroudi, M. (2023). A hybrid optimization method to design a sustainable resilient supply chain in a perishable food industry. Environmental Science and Pollution Research, 30 (3), 6080–6103. https://doi.org/10.1007/s11356-022-22115-8
Article Google Scholar
Aboolian, R., Cui, T., & Shen, Z. J. M. (2013). An efficient approach for solving reliable facility location models. INFORMS Journal on Computing, 25 (4), 720–729. https://doi.org/10.1287/ijoc.1120.0534
Aksen, D., & Aras, N. (2012). A bilevel fixed charge location model for facilities under imminent attack. Computers and Operations Research, 39 (7), 1364–1381. https://doi.org/10.1016/j.cor.2011.08.006
Albareda-Sambola, M., Hinojosa, Y., & Puerto, J. (2015). The reliable P-median problem with at-facility service. European Journal of Operational Research, 245 (3), 656–666. https://doi.org/10.1016/j.ejor.2015.03.049
Albareda-Sambola, M., Landete, M., Monge, J. F., & Sainz-Pardo, J. L. (2017). Introducing capacities in the location of unreliable facilities. European Journal of Operational Research, 259 (1), 175–188. https://doi.org/10.1016/j.ejor.2016.10.013
Alcaraz, J., Landete, M., & Monge, J. F. (2012). Design and analysis of hybrid metaheuristics for the reliability p-median problem. European Journal of Operational Research, 222 (1), 54–64. https://doi.org/10.1016/j.ejor.2012.04.016
Alcaraz, J., Landete, M., Monge, J. F., & Sainz-Pardo, J. L. (2015). Strengthening the reliability fixed-charge location model using clique constraints. Computers and Operations Research, 60 , 14–26. https://doi.org/10.1016/j.cor.2015.02.003
Aldrighetti, R., Battini, D., & Ivanov, D. (2023). Efficient resilience portfolio design in the supply chain with consideration of preparedness and recovery investments. Omega, 117 , 102841.
Aldrighetti, R., Battini, D., Ivanov, D., & Zennaro, I. (2021). Costs of resilience and disruptions in supply chain network design models: A review and future research directions. International Journal of Production Economics, 235 (5), 25. https://doi.org/10.1016/j.ijpe.2021.108103
Alikhani, R., Ali Torabi, S., & Altay, N. (2021). Retail supply chain network design with concurrent resilience capabilities. International Journal of Production Economics . https://doi.org/10.1016/j.ijpe.2021.108042
Alikhani, R., Eskandarpour, M., & Jahani, H. (2023a). Collaborative distribution network design with surging demand and facility disruptions. International Journal of Production Economics, 262 , 108912.
Alikhani, R., Ranjbar, A., Jamali, A., Torabi, S. A., & Zobel, C. W. (2023b). Towards increasing synergistic effects of resilience strategies in supply chain network design. Omega, 116 , 102819.
An, Y., Zeng, B., Zhang, Y., & Zhao, L. (2014). Reliable P-median facility location problem: Two-stage robust models and algorithms. Transportation Research Part b: Methodological, 64 , 54–72. https://doi.org/10.1016/j.trb.2014.02.005
Aydin, N., & Murat, A. (2013). A swarm intelligence based sample average approximation algorithm for the capacitated reliable facility location problem. In International Journal of Production Economics, 145 , 173–183. https://doi.org/10.1016/j.ijpe.2012.10.019
Azad, N., & Hassini, E. (2019). A benders decomposition method for designing reliable supply chain networks accounting for multimitigation strategies and demand losses. Transportation Science, 53 (5), 1287–1312. https://doi.org/10.1287/trsc.2018.0875
Benyoucef, L., Xie, X., & Tanonkou, G. A. (2013). Supply chain network design with unreliable suppliers: a lagrangian relaxation-based approach. International Journal of Production Research, 51 (21), 6435–6454. https://doi.org/10.1080/00207543.2013.824129
Berger, P. D., & Zeng, A. Z. (2006). Single versus multiple sourcing in the presence of risks. Journal of the Operational Research Society, 57 (3), 250–261. https://doi.org/10.1057/palgrave.jors.2601982
Berman, O., Krass, D., & Menezes, M. B. C. (2007). Facility reliability issues in network p-median problems: strategic centralization and co-location effects. Operations Research, 55 (2), 332–350. https://doi.org/10.1287/opre.1060.0348
Chapman, P., Christopher, M., Jüttner, U., Peck, H., & Wilding, R. (2002). Identifying and managing supply chain vulnerability. Logistics and Transport Focus, 4 (4), 256.
Google Scholar
Cheng, C., Adulyasak, Y., & Rousseau, L. M. (2021). Robust facility location under demand uncertainty and facility disruptions. Omega (united Kingdom) . https://doi.org/10.1016/j.omega.2021.102429
Cheng, C., Qi, M., Zhang, Y., & Rousseau, L. M. (2018). A two-stage robust approach for the reliable logistics network design problem. Transportation Research Part b: Methodological, 111 (May), 185–202. https://doi.org/10.1016/j.trb.2018.03.015
Chopra, S. & Sodhi, M. S. (2004). Managing risk to avoid supply-chain breakdown. MIT Sloan Management Review .
Chowdhury, M. M. H., & Quaddus, M. (2017). Supply chain resilience: Conceptualization and scale development using dynamic capability theory. International Journal of Production Economics, 188 (June), 185–204. https://doi.org/10.1016/j.ijpe.2017.03.020
Christopher, M., & Peck, H. (2004). Building the resilient supply chain. The International Journal of Logistics Management, 15 (2), 1–14.
Craighead, C. W., Jennifer Blackhurst, M., Rungtusanatham, J., & Handfield, R. B. (2007a). The severity of supply chain disruptions: Design characteristics and mitigation capabilities. The Author Journal Compilation C, 38 , 25–28.
Craighead, C. W., Jennifer Blackhurst, M., Rungtusanatham, J., & Handfield, R. B. (2007b). The severity of supply chain disruptions: design characteristics and mitigation capabilities. Decision Sciences, 38 (1), 131–156. https://doi.org/10.1111/j.1540-5915.2007.00151.x
Cui, T., Ouyang, Y., & Shen, Z. J. M. (2010). Reliable facility location design under the risk of disruptions. Operations Research, 58 (4 PART 1), 998–1011. https://doi.org/10.1287/opre.1090.0801
Dolgui, A., Ivanov, D., & Sokolov, B. (2018). Ripple effect in the supply chain: An analysis and recent literature. International Journal of Production Research, 56 (1–2), 414–430. https://doi.org/10.1080/00207543.2017.1387680
Dupont, L., Bernard, C., Hamdi, F., & Masmoudi, F. (2018). Supplier selection under risk of delivery failure: A decision-support model considering managers’ risk sensitivity. International Journal of Production Research, 56 (3), 1054–1069. https://doi.org/10.1080/00207543.2017.1364442
Egri, P., Dávid, B., Kis, T., & Krész, M. (2023). Robust facility location in reverse logistics. Annals of Operations Research, 324 (1), 163–188.
Elluru, S., Gupta, H., Kaur, H., & Singh, S. P. (2019). Proactive and reactive models for disaster resilient supply chain. Annals of Operations Research, 283 (1–2), 199–224. https://doi.org/10.1007/s10479-017-2681-2
Enayati, S., & Özaltın, O. Y. (2024). Supplier selection under disruption risk with hybrid procurement. Computers and Operations Research, 45 , 106593.
Erdoĝan, S., & Miller-Hooks, E. (2012). A green vehicle routing problem. Transportation Research Part e: Logistics and Transportation Review, 48 (1), 100–114. https://doi.org/10.1016/j.tre.2011.08.001
Fahimnia, B., Jabbarzadeh, A., & Sarkis, J. (2018). Greening versus resilience: A supply chain design perspective. Transportation Research Part e: Logistics and Transportation Review, 119 (November), 129–148. https://doi.org/10.1016/j.tre.2018.09.005
Fattahi, M., Govindan, K., & Keyvanshokooh, E. (2017). Responsive and resilient supply chain network design under operational and disruption risks with delivery lead-time sensitive customers. Transportation Research Part e: Logistics and Transportation Review, 101 (May), 176–200. https://doi.org/10.1016/j.tre.2017.02.004
Fattahi, M., Govindan, K., & Maihami, R. (2020). Stochastic optimization of disruption-driven supply chain network design with a new resilience metric. International Journal of Production Economics . https://doi.org/10.1016/j.ijpe.2020.107755
Feng, Y., Chen, Y., & Liu, Y. (2023). Optimising two-stage robust supplier selection and order allocation problem under risk-averse criterion. International Journal of Production Research, 61 (19), 6356–6380.
Firouz, M., Keskin, B. B., & Melouk, S. H. (2017). An integrated supplier selection and inventory problem with multi-sourcing and lateral transshipments. Omega (united Kingdom), 70 (July), 77–93. https://doi.org/10.1016/j.omega.2016.09.003
Garvey, M. D., Carnovale, S., & Yeniyurt, S. (2015). An analytical framework for supply network risk propagation: A Bayesian network approach. European Journal of Operational Research, 243 (2), 618–627. https://doi.org/10.1016/j.ejor.2014.10.034
Ghanei, S., Contreras, I., & Cordeau, J. F. (2023). A two-stage stochastic collaborative intertwined supply network design problem under multiple disruptions. Transportation Research Part e: Logistics and Transportation Review, 170 , 102944.
Ghavamifar, A., Makui, A., & Taleizadeh, A. A. (2018). Designing a resilient competitive supply chain network under disruption risks: A real-world application. Transportation Research Part e: Logistics and Transportation Review, 115 (July), 87–109. https://doi.org/10.1016/j.tre.2018.04.014
Gholami-Zanjani, S. M., Jabalameli, M. S., Klibi, W., & Pishvaee, M. S. (2021a). A robust location-inventory model for food supply chains operating under disruptions with ripple effects. International Journal of Production Research, 59 (1), 301–324. https://doi.org/10.1080/00207543.2020.1834159
Gholami-Zanjani, S. M., Klibi, W., Jabalameli, M. S., & Pishvaee, M. S. (2021b). The design of resilient food supply chain networks prone to epidemic disruptions. International Journal of Production Economics . https://doi.org/10.1016/j.ijpe.2020.108001
Hasani, A., & Khosrojerdi, A. (2016). Robust global supply chain network design under disruption and uncertainty considering resilience strategies: A parallel memetic algorithm for a real-life case study. Transportation Research Part e: Logistics and Transportation Review, 87 (March), 20–52. https://doi.org/10.1016/j.tre.2015.12.009
Hernandez, I., Ramirez-Marquez, J. E., Rainwater, C., Pohl, E., & Medal, H. (2014). Robust facility location: Hedging against failures. Reliability Engineering and System Safety, 123 , 73–80. https://doi.org/10.1016/j.ress.2013.10.006
Hervani, A. A., Nandi, S., Helms, M. M., & Sarkis, J. (2022). A performance measurement framework for socially sustainable and resilient supply chains using environmental goods valuation methods. Sustainable Production and Consumption, 30 (March), 31–52. https://doi.org/10.1016/j.spc.2021.11.026
Hosseini, S., Ivanov, D., & Dolgui, A. (2019a). Review of quantitative methods for supply chain resilience analysis. Transportation Research Part e: Logistics and Transportation Review, 125 (May), 285–307. https://doi.org/10.1016/j.tre.2019.03.001
Hosseini, S., Morshedlou, N., Dmitry Ivanov, M. D., Sarder, K. B., & Khaled, A. A. (2019b). Resilient supplier selection and optimal order allocation under disruption risks. International Journal of Production Economics, 213 (July), 124–137. https://doi.org/10.1016/j.ijpe.2019.03.018
Ivanov, D., Dolgui, A., Sokolov, B., & Ivanova, M. (2017). Literature review on disruption recovery in the supply chain. International Journal of Production Research, 55 (20), 6158–6174. https://doi.org/10.1080/00207543.2017.1330572
Jabbarzadeh, A., Fahimnia, B., & Sabouhi, F. (2018). Resilient and sustainable supply chain design: sustainability analysis under disruption risks. International Journal of Production Research, 56 (17), 5945–5968. https://doi.org/10.1080/00207543.2018.1461950
Jabbarzadeh, A., Fahimnia, B., Sheu, J. B., & Moghadam, H. S. (2016). Designing a supply chain resilient to major disruptions and supply/demand interruptions. Transportation Research Part b: Methodological, 94 (December), 121–149. https://doi.org/10.1016/j.trb.2016.09.004
Jiang, Y., & Zografos, K. G. (2021). A decision making framework for incorporating fairness in allocating slots at capacity-constrained airports. Transportation Research Part c: Emerging Technologies . https://doi.org/10.1016/j.trc.2021.103039
Kabadurmus, O., & Erdogan, M. S. (2020). Sustainable, multimodal and reliable supply chain design. Annals of Operations Research, 292 (1), 47–70. https://doi.org/10.1007/s10479-020-03654-0
Kamal, M. M., & Irani, Z. (2014). Analysing supply chain integration through a systematic literature review: A normative perspective. Supply Chain Management, 19 (September), 523–557. https://doi.org/10.1108/SCM-12-2013-0491
Kamalahmadi, M., Shekarian, M., & Parast, M. M. (2022). The impact of flexibility and redundancy on improving supply chain resilience to disruptions. International Journal of Production Research, 60 (6), 1992–2020. https://doi.org/10.1080/00207543.2021.1883759
Katsaliaki, K., Galetsi, P., & Kumar, S. (2021). Supply chain disruptions and resilience: A major review and future research agenda. Annals of Operations Research . https://doi.org/10.1007/s10479-020-03912-1
Kaur, H., & Singh, S. P. (2021). Multi-stage hybrid model for supplier selection and order allocation considering disruption risks and disruptive technologies. International Journal of Production Economics . https://doi.org/10.1016/j.ijpe.2020.107830
Kinra, A., Ivanov, D., Das, A., & Dolgui, A. (2020). Ripple effect quantification by supplier risk exposure assessment. International Journal of Production Research, 58 (18), 5559–5578. https://doi.org/10.1080/00207543.2019.1675919
Kungwalsong, K., Mendoza, A., Kamath, V., Pazhani, S., & Marmolejo-Saucedo, J. A. (2022). An application of interactive fuzzy optimization model for redesigning supply chain for resilience. Annals of Operations Research . https://doi.org/10.1007/s10479-022-04542-5
Li, Q., Zeng, Bo., & Savachkin, A. (2013b). Reliable facility location design under disruptions. Computers and Operations Research, 40 (4), 901–909. https://doi.org/10.1016/j.cor.2012.11.012
Li, X., Ouyang, Y., & Peng, F. (2013a). A supporting station model for reliable infrastructure location design under interdependent disruptions. Transportation Research Part e: Logistics and Transportation Review, 60 , 80–93.
Li, X., Ouyang, Y., & Peng, F. (2013c). A supporting station model for reliable infrastructure location design under interdependent disruptions. Transportation Research Part e: Logistics and Transportation Review, 60 , 80–93. https://doi.org/10.1016/j.tre.2013.06.005
Liberatore, F., Scaparra, M. P., & Daskin, M. S. (2012). Hedging against disruptions with ripple effects in location analysis. Omega, 40 (1), 21–30. https://doi.org/10.1016/j.omega.2011.03.003
Lim, M., Daskin, M. S., Bassamboo, A., & Chopra, S. (2010). A facility reliability problem: formulation, properties, and algorithm. Naval Research Logistics, 57 (1), 58–70. https://doi.org/10.1002/nav.20385
Lu, M., Ran, L., & Shen, Z. J. M. (2015). Reliable facility location design under uncertain correlated disruptions. Manufacturing and Service Operations Management, 17 (4), 445–455. https://doi.org/10.1287/msom.2015.0541
Lu, X., & Cheng, C. (2021). Locating facilities with resiliency to capacity failures and correlated demand uncertainty. Transportation Research Part e: Logistics and Transportation Review . https://doi.org/10.1016/j.tre.2021.102444
Maharjan, R., & Kato, H. (2022). Resilient supply chain network design: a systematic literature review. Transport Reviews, 42 (6), 739–761. https://doi.org/10.1080/01441647.2022.2080773
Maliki, F., Souier, M., Dahane, M., & Ben Abdelaziz, F. (2022). A multi-objective optimization model for a multi-period mobile facility location problem with environmental and disruption considerations. Annals of Operations Research, 25 , 1–26.
Meng, L., He, J., Shaolong, Hu., & Han, C. (2021). Strategic reliable supply chain network design: determining tradeoffs between cost and risk. International Journal of Production Research . https://doi.org/10.1080/00207543.2021.1994163
Miemczyk, J., & Johnsen, T. E. (2012). Sustainable purchasing and supply management: A structured literature review of definitions and measures at the dyad, chain and network levels. Supply Chain Management: An International Journal, 17 (5), 478–496. https://doi.org/10.1108/13598541211258564
Naghshineh, B., & Carvalho, H. (2022). The implications of additive manufacturing technology adoption for supply chain resilience: A systematic search and review. International Journal of Production Economics, 2 , 2. https://doi.org/10.1016/j.ijpe.2021.108387
Namdar, J., Ali Torabi, S., Sahebjamnia, N., & Pradhan, N. N. (2021). Business continuity-inspired resilient supply chain network design. International Journal of Production Research, 59 (5), 1331–1367. https://doi.org/10.1080/00207543.2020.1798033
Nayeri, S., Tavakoli, M., Tanhaeean, M., & Jolai, F. (2022). A robust fuzzy stochastic model for the responsive-resilient inventory-location problem: comparison of metaheuristic algorithms. Annals of Operations Research, 25 , 1–41.
Nooraie, S. V., & Parast, M. M. (2016). Mitigating supply chain disruptions through the assessment of trade-offs among risks, costs and investments in capabilities. International Journal of Production Economics, 171 , 8–21.
Norrman, A., & Jansson, U. (2004). Ericsson’s proactive supply chain risk management approach after a serious sub-supplier accident. International Journal of Physical Distribution and Logistics Management, 34 (5), 434–456. https://doi.org/10.1108/09600030410545463
O’Hanley, J. R., Paola Scaparra, M., & García, S. (2013). Probability chains: A general linearization technique for modeling reliability in facility location and related problems. European Journal of Operational Research, 230 (1), 63–75. https://doi.org/10.1016/j.ejor.2013.03.021
Park, Y. W., Hong, P., & Roh, J. J. (2013). Supply chain lessons from the catastrophic natural disaster in Japan. Business Horizons, 56 (1), 75–85. https://doi.org/10.1016/j.bushor.2012.09.008
Paul, S. K., Priyabrata Chowdhury, Md., Moktadir, A., & Lau, K. H. (2021). Supply chain recovery challenges in the wake of COVID-19 pandemic. Journal of Business Research, 136 (November), 316–329. https://doi.org/10.1016/j.jbusres.2021.07.056
Pettit, T. J., Croxton, K. L. & Fiksel, J. (n.d.) Ensuring supply chain resilience: Development and implementation of an assessment tool.
Ponomarov, S. Y., & Holcomb, M. C. (2009). Understanding the concept of supply chain resilience. The International Journal of Logistics Management, 20 (1), 124–143. https://doi.org/10.1108/09574090910954873
PrasannaVenkatesan, S., & Goh, M. (2016). Multi-objective supplier selection and order allocation under disruption risk. Transportation Research Part e: Logistics and Transportation Review, 95 (November), 124–142. https://doi.org/10.1016/j.tre.2016.09.005
Rajabzadeh, H., & Babazadeh, R. (2022). A game-theoretic approach for power pricing in a resilient supply chain considering a dual channel biorefining structure and the hybrid power plant. Renewable Energy, 198 (October), 1082–1094. https://doi.org/10.1016/j.renene.2022.08.118
Rajagopal, V., Venkatesan, S. P., & Goh, M. (2017). Decision-making models for supply chain risk mitigation: A review. Computers and Industrial Engineering . https://doi.org/10.1016/j.cie.2017.09.043
Ravindran, A., Ravi, R. U., Bilsel, V. W., & Yang, T. (2010). Risk adjusted multicriteria supplier selection models with applications. International Journal of Production Research, 48 (2), 405–424. https://doi.org/10.1080/00207540903174940
Rezapour, S., Farahani, R. Z., & Pourakbar, M. (2017). Resilient supply chain network design under competition: a case study. European Journal of Operational Research, 259 (3), 1017–1035. https://doi.org/10.1016/j.ejor.2016.11.041
Sabouhi, F., Jabalameli, M. S., Jabbarzadeh, A., & Fahimnia, B. (2020). A multi-cut L-shaped method for resilient and responsive supply chain network design. International Journal of Production Research, 25 , 7353–7381. https://doi.org/10.1080/00207543.2020.1779369
Saputro, T. E., Figueira, G., & Almada-Lobo, B. (2021). Integrating supplier selection with inventory management under supply disruptions. International Journal of Production Research, 59 (11), 3304–3322. https://doi.org/10.1080/00207543.2020.1866223
Saunders, M., Lewis, P., & Thornhill, A. (2009). Research methods for business students (5th ed.). Pearson Education.
Sawik, T. (2011). Selection of supply portfolio under disruption risks. Omega, 39 (2), 194–208. https://doi.org/10.1016/j.omega.2010.06.007
Sawik, T. (2013a). Selection of resilient supply portfolio under disruption risks. Omega (united Kingdom), 41 (2), 259–269. https://doi.org/10.1016/j.omega.2012.05.003
Sawik, T. (2013b). Integrated selection of suppliers and scheduling of customer orders in the presence of supply chain disruption risks. International Journal of Production Research, 51 (23–24), 7006–7022. https://doi.org/10.1080/00207543.2013.852702
Sawik, T. (2014). Joint supplier selection and scheduling of customer orders under disruption risks: Single vs. dual sourcing. Omega (united Kingdom), 43 (March), 83–95. https://doi.org/10.1016/j.omega.2013.06.007
Sawik, T. (2017). A portfolio approach to supply chain disruption management. International Journal of Production Research, 55 (7), 1970–1991. https://doi.org/10.1080/00207543.2016.1249432
Sawik, T. (2019). Two-period vs. multi-period model for supply chain disruption management. International Journal of Production Research, 57 (14), 4502–4518. https://doi.org/10.1080/00207543.2018.1504246
Sawik, T. (2020). A two-period model for selection of resilient multi-tier supply portfolio. International Journal of Production Research, 58 (19), 6043–6060. https://doi.org/10.1080/00207543.2019.1665204
Sawik, T. (2021). On the risk-averse selection of resilient multi-tier supply portfolio. Omega (united Kingdom) . https://doi.org/10.1016/j.omega.2020.102267
Scaparra, M. P., & Church, R. L. (2008). An exact solution approach for the interdiction median problem with fortification. European Journal of Operational Research, 189 (1), 76–92. https://doi.org/10.1016/j.ejor.2007.05.027
Sepehri, A., Tirkolaee, E. B., Simic, V., & Ali, S. S. (2024). Designing a reliable-sustainable supply chain network: Adaptive m-objective ε-constraint method. Annals of Operations Research, 145 , 1–32.
Sheffi, Y., & Rice Jr., J. B. (2005). A supply chain view of the resilient enterprise. https://www.researchgate.net/publication/255599289
Shekarian, M., & Parast, M. M. (2021). An integrative approach to supply chain disruption risk and resilience management: A literature review. International Journal of Logistics Research and Applications, 24 (5), 427–455. https://doi.org/10.1080/13675567.2020.1763935
Shen, Z.-J. (2007). Integrated supply chain design models: a survey and future research directions. Journal of Industrial and Management Optimization, 3 (1), 25.
Shen, Z.-J.M. (2007). Integrated supply chain design models: a survey and future research directions. Journal of Industrial and Management Optimization, 3 (1), 1.
Shen, Z. J. M., Zhan, R. L., & Zhang, J. (2011). The reliable facility location problem: formulations, heuristics, and approximation algorithms. INFORMS Journal on Computing, 23 (3), 470–482. https://doi.org/10.1287/ijoc.1100.0414
Simchi-Levi, D., & Kaminsky, P. (2004). Managing the supply chain: Definitive guide . McGraw-Hill.
Snyder, L. V., Atan, Z., Peng, P., Rong, Y., Schmitt, A. J., & Sinsoysal, B. (2016). OR/MS models for supply chain disruptions: A review . Taylor and Francis Ltd. https://doi.org/10.1080/0740817X.2015.1067735
Book Google Scholar
Snyder, L. V., & Daskin, M. S. (2005). Reliability models for facility location: The expected failure cost case. Transportation Science, 39 (3), 400–416. https://doi.org/10.1287/trsc.1040.0107
Spiegler, V. L. M., Naim, M. M., & Wikner, J. (2012). A control engineering approach to the assessment of supply chain resilience. In International Journal of Production Research, 50 , 6162–6187. https://doi.org/10.1080/00207543.2012.710764
Starita, S., & Scaparra, M. P. (2021). Improving supply system reliability against random disruptions: strategic protection investment. Journal of the Operational Research Society . https://doi.org/10.1080/01605682.2021.1911605
Suryawanshi, P., & Dutta, P. (2022). Optimization models for supply chains under risk, uncertainty, and resilience: A state-of-the-art review and future research directions. Transportation Research Part e: Logistics and Transportation Review . https://doi.org/10.1016/j.tre.2021.102553
Taleizadeh, A. A., Ghavamifar, A., & Khosrojerdi, A. (2022). Resilient network design of two supply chains under price competition: Game theoretic and decomposition algorithm approach. Operational Research, 22 (1), 825–857. https://doi.org/10.1007/s12351-020-00565-7
Tang, C. S. (2006). Perspectives in supply chain risk management. International Journal of Production Economics . https://doi.org/10.1016/j.ijpe.2005.12.006
Tomlin, B. (2006). On the value of mitigation and contingency strategies for managing supply chain disruption risks. Source: Management Science, 52 , 639–657.
Torabi, S. A., Baghersad, M., & Mansouri, S. A. (2015). Resilient supplier selection and order allocation under operational and disruption risks. Transportation Research Part e: Logistics and Transportation Review, 79 (July), 22–48. https://doi.org/10.1016/j.tre.2015.03.005
Tranfield, D., Denyer, D., & Smart, P. (2003). Towards a methodology for developing evidence-informed management knowledge by means of systematic review. British Journal of Management, 14 (3), 207–222.
Tucker, E. L., Daskin, M. S., Sweet, B. V., & Hopp, W. J. (2020). Incentivizing resilient supply chain design to prevent drug shortages: policy analysis using two- and multi-stage stochastic programs. IISE Transactions, 52 (4), 394–412. https://doi.org/10.1080/24725854.2019.1646441
Tukamuhabwa, B. R., Stevenson, M., Busby, J., & Zorzini, M. (2015). Supply chain resilience: definition, review and theoretical foundations for further study. International Journal of Production Research . https://doi.org/10.1080/00207543.2015.1037934
Vahid Nooraie, S., & Parast, M. M. (2016). Mitigating supply chain disruptions through the assessment of trade-offs among risks, costs and investments in capabilities. International Journal of Production Economics, 171 (January), 8–21. https://doi.org/10.1016/j.ijpe.2015.10.018
van Remko, H. (2020). Research opportunities for a more resilient post-COVID-19 supply chain: Closing the gap between research findings and industry practice. International Journal of Operations and Production Management, 40 (4), 341–355. https://doi.org/10.1108/IJOPM-03-2020-0165
Wieland, A., & Durach, C. F. (2021). Two perspectives on supply chain resilience. Journal of Business Logistics, 42 (3), 315–322. https://doi.org/10.1111/jbl.12271
Xie, S., An, K., & Ouyang, Y. (2019). Planning facility location under generally correlated facility disruptions: Use of supporting stations and quasi-probabilities. Transportation Research Part b: Methodological, 122 (April), 115–139. https://doi.org/10.1016/j.trb.2019.02.001
Yildiz, H., Yoon, J., Talluri, S., & Ho, W. (2016). Reliable supply chain network design. Decision Sciences, 47 , 661–698.
Yoon, J., Talluri, S., Yildiz, H., & Ho, W. (2018). Models for supplier selection and risk mitigation: A holistic approach. International Journal of Production Research, 56 (10), 3636–3661. https://doi.org/10.1080/00207543.2017.1403056
Young, J. A., & Bhattacharyya, K. (2020). No chicken at KFC: A logistics debacle? Journal of Critical Incidents, 13 , 256.
Yu, G., Haskell, W. B., & Liu, Y. (2017). Resilient facility location against the risk of disruptions. Transportation Research Part b: Methodological, 104 (October), 82–105. https://doi.org/10.1016/j.trb.2017.06.014
Yu, G., & Zhang, J. (2018). Multi-dual decomposition solution for risk-averse facility location problem. Transportation Research Part e: Logistics and Transportation Review, 116 (August), 70–89. https://doi.org/10.1016/j.tre.2018.05.010
Yun, L., Qin, Y., Fan, H., Ji, C., Li, X., & Jia, L. (2015). A reliability model for facility location design under imperfect information. Transportation Research Part b: Methodological, 81 (November), 596–615. https://doi.org/10.1016/j.trb.2014.10.010
Yun, L., Wang, X., Fan, H., & Li, X. (2020). Reliable facility location design with round-trip transportation under imperfect information part I: A discrete model. Transportation Research Part e: Logistics and Transportation Review, 133 (January), 25. https://doi.org/10.1016/j.tre.2019.101825
Zhang, Y., Huang, M., Gao, Z., Jiang, S., Fang, S. C., & Wang, X. (2024). Multi-period fourth-party logistics network design from the viability perspective: A collaborative hyper-heuristic embedded with double-layer Q-learning algorithm. International Journal of Production Research . https://doi.org/10.1080/00207543.2024.2339530
Zhang, Y., Snyder, L. V., Ralphs, T. K., & Xue, Z. (2016). The competitive facility location problem under disruption risks. Transportation Research Part e: Logistics and Transportation Review, 93 (September), 453–473. https://doi.org/10.1016/j.tre.2016.07.002
Zhao, K., Zuo, Z., & Blackhurst, J. V. (2019). Modelling supply chain adaptation for disruptions: An empirically grounded complex adaptive systems approach. Journal of Operations Management, 65 (2), 190–212. https://doi.org/10.1002/joom.1009
Download references
Acknowledgements
This work was supported by the UK Engineering and Physical Sciences Research Council (EPSRC) as part of the Responsive Additive Manufacture to Overcome Natural and Attack-based disruption (RAMONA) project grant [EP/V051040/1].
Author information
Authors and affiliations.
Department of Business Analytics and Operations, Surrey Business School, University of Surrey, Guildford, UK
Abdolreza Roshani
Centre of Digital Economy, Surrey Business School, Guildford, UK
Glenn Parry
University of Bristol Business School, University of Bristol, Bristol, UK
Philip Walker-Davies
You can also search for this author in PubMed Google Scholar
Corresponding author
Correspondence to Abdolreza Roshani .
Ethics declarations
Conflict of interest.
The authors declare that there is no conflict of interest regarding the publication of this paper.
Additional information
Publisher's note.
Springer Nature remains neutral with regard to jurisdictional claims in published maps and institutional affiliations.
Electronic supplementary material
Below is the link to the electronic supplementary material.
Supplementary file1 (DOCX 489 kb)
The list of articles are presented for each strategy based on the associated DM problem discussed in Sect. 2.3. The last column of the Table 8 represents how often each strategy is applied in models in the articles (as a percent of total). In order to refer to the strategies more easily, in Table 8 , we have also assigned a code to each mitigation strategy e.g. SP1 is suppliers protection or fortification strategy.
Rights and permissions
Open Access This article is licensed under a Creative Commons Attribution 4.0 International License, which permits use, sharing, adaptation, distribution and reproduction in any medium or format, as long as you give appropriate credit to the original author(s) and the source, provide a link to the Creative Commons licence, and indicate if changes were made. The images or other third party material in this article are included in the article's Creative Commons licence, unless indicated otherwise in a credit line to the material. If material is not included in the article's Creative Commons licence and your intended use is not permitted by statutory regulation or exceeds the permitted use, you will need to obtain permission directly from the copyright holder. To view a copy of this licence, visit http://creativecommons.org/licenses/by/4.0/ .
Reprints and permissions
About this article
Roshani, A., Walker-Davies, P. & Parry, G. Designing resilient supply chain networks: a systematic literature review of mitigation strategies. Ann Oper Res (2024). https://doi.org/10.1007/s10479-024-06228-6
Download citation
Received : 20 December 2022
Accepted : 15 August 2024
Published : 18 September 2024
DOI : https://doi.org/10.1007/s10479-024-06228-6
Share this article
Anyone you share the following link with will be able to read this content:
Sorry, a shareable link is not currently available for this article.
Provided by the Springer Nature SharedIt content-sharing initiative
- Supply chain
- Network design
- Resilience strategies
- Mitigation Strategies
- Systematic literature review
- Find a journal
- Publish with us
- Track your research

COMMENTS
Network monitoring has played an important role in syndromic surveillance and monitoring epidemic spread, as well as monitoring information spread at the onset of social movements, including protests, riots, and uprisings. To date, many existing methodologies in this area rely on principles founded in statistical process monitoring.
Due to the increasingly complex and interconnected nature of global supply chain networks (SCNs), a recent strand of research has applied network science methods to model SCN growth and subsequently analyse various topological features, such as robustness. This paper provides: (1) a comprehensive review of the methodologies adopted in literature for modelling the topology and robustness of ...
We capitalize on this mathematical framework to review theoretical and computational approaches to characterize robustness and resilience of complex networks. We discuss recent approaches to ...
In this review, we show that network science has been and will continue to be a fruitful theoretical and methodological framework that advances our understanding of human cognition. ... A literature review of psychology papers published in the 1970s (which coincides with the burgeoning of connectionism) that mentioned the term 'graph theory ...
This paper provides: (1) a comprehensive review of the methodologies adopted in literature for modelling the topology and robustness of SCNs; (2) a summary of topological features of the real world SCNs, as reported in various data driven studies; and (3) a discussion on the limitations of existing network growth models to realistically ...
The number of network science applications across many different fields has been rapidly increasing. Surprisingly, the development of theory and domain-specific applications often occur in ...
Abstract. Albert-Làaszlò Barabàasi can be credited with bringing network science to the general public. After a series of papers in such heavyweights as Nature and Science, his book Linked gave a popular science account of the field. With Network Science he turns his attention to newcomer students who want to start from scratch and go ...
Omnipresent network/graph data generally have the characteristics of nonlinearity, sparseness, dynamicity and heterogeneity, which bring numerous challenges to network related analysis problem. Recently, influenced by the excellent ability of deep learning to learn representation from data, representation learning for network data has gradually become a new research hotspot. Network ...
For decades, the spatial approach to network analysis has principally focused on planar and technical networks from a classic graph theory perspective. Reference to models and methods developed by other disciplines on non-planar networks, such as sociology and physics, is recent, limited, and dispersed. Conversely, the physics literature that developed the popular scale-free and small-world ...
This paper provides: (1) a comprehensive review of the methodologies adopted in literature for modelling the topology and robustness of SCNs; (2) a summary of topological features of the real ...
In the network science literature, random failures and targeted attacks in networks are typically simulated as follows; ... A review of contemporary literature on SCN topologies reveals that only a limited number of data driven studies are available in this domain. This is mainly due to difficulty in obtaining specific information about ...
Although a comprehensive review of these network generation methods is beyond the scope of this chapter, we discuss the fundamental models in Section 5.2, which have been proposed in the network science literature to characterize the structure of systems from various domains and have also been adopted as models of supply networks in some studies.
The network approach to psychopathology: a review of the literature 2008-2018 and an agenda for future research. Psychol. Med. 50, ... Barabasi, A. L. Network Science ...
2 Methodology. This chapter aims at analysing the main trends and characteristics of the literature regarding networks and organizations in the last 20 years (2002-2021). Following Massaro, Dumay, and Guthrie (2016) we developed a structured literature review, starting with setting boundaries to our research.
A literature review is presented on the modes of effective network leadership within and between organizations. In total, 163 published studies, both empirical and conceptual, were parsed with regard to definitions, contexts, antecedents, and outcomes, leading to four propositions.
Network Science (NWS) is an interdisciplinary journal aiming to consolidate and advance the emerging discipline of the same name. Recognizing an ever-increasing relevance across domains,the journal is focused on inspiring applications of network approaches, rigorous theory building, and innovative methods. We therefore encourage submissions ...
Explore the latest full-text research PDFs, articles, conference papers, preprints and more on NETWORK TOPOLOGY. Find methods information, sources, references or conduct a literature review on ...
Network science is the subject of many conferences in a variety of different fields . ... measurements of the network, and social influence within networks. A literature review is provided including the evolution of networks and models through the years, from simple lattices to more complex models. The pros and cons of each model are ...
Third, the authors found that the focal firms' NC consists of two key dimensions: network development capability (NDC) and network management capability (NMC). Fourth, they contribute to the literature by providing a set of suggestions for further research in the NC literature stream.,This study has some limitations, like other review studies.
We conducted a systematic review of empirical network effects research in 40 public administration and policy journals from 1998 to 2019. We reviewed and coded 89 articles and described the main social theories used in the network effectiveness literature and the associated mechanisms that translate network structures to network effects.
In the face of a large literature, several studies have been conducted to review the literature on various aspects of sustainable/green operations and supply chains, such as conceptual frameworks (Ahi and Searcy, 2013, Dubey et al., 2017), quantitative models (Brandenburg & Rebs, 2015), indicators (Hassini et al., 2012), and empirical research ...
W~ 0 mm R ii Network theory - a systematic method for literature review Nurse Education Todav (1992)12,44-50 Longman Group UK Ltd 1992 Stephen E O'Connor This article sets out the nature and purpose of the literature review as it is found in the nursing research literature. The `standard' method of the construction of a review is set out and ...
Web applications, accessible via web browsers over the Internet, facilitate complex functionalities without local software installation. In the context of web applications, a workload refers to the number of user requests sent by users or applications to the underlying system. Existing studies have leveraged web application workloads to achieve various objectives, such as workload prediction ...
Then, the problem characteristics, modeling uncertainty, and solution methods are analyzed. This systematic literature review serves as a guide for further studies by analyzing uncertainty, risk, and resilience in the biomass supply chain network design, and identifies a list of potential issues for future research directions.
This literature review proposes a systematic approach, analysing the best-known databases in the field of scientific research (Google Scholar, Scopus and Science Direct) in order to catalogue and systematise the most consonant approach according to certain characteristics in the study of UHIs.
A network approach toward literature review 627 2.1 Network measures In addition to visualizing the network we can also analyze network, using metrics computed at the node, dyad and network levels. These metrics provide a descriptive overview of the work that has been done in a specific research area. 2.1.1 Measures at the node level
The review assists with examining and mapping existing knowledge and to identify research gaps in the field of interest and certain subjects within that field which can further grow and develop the knowledge base [37]. further posits that a systematic literature review (SLR) is different from the traditional narrative reviews as it adopts ...
This study introduces a method that uses a network approach towards literature review. To employ this approach, we use hypotheses proposed in scientific publications as building blocks. In network terms, a hypothesis is a directed tie between two concepts or nodes. The network emerges by aggregating the hypotheses from a set of articles in a specific domain. This study explains the method and ...
This case-based systematic literature review was prepared and presented by using the Preferred Reporting Items of Systematic Reviews and Meta-Analysis guidelines (PRISMA) to ensure quality, validity, and transparency (Moher et al., 2015), and it seeks to provide an in-depth understanding of the current stage of urban climate scholarship ...
With increased globalisation supply chain (SC) disruption significantly affects people, organisations and society. Supply chain network design (SCND) reduces the effects of disruption, employing mitigation strategies such as extra capacity and flexibility to make SCs resilient. Currently, no systematic literature review classifies mitigation strategies for SCND. This paper systematically ...